Development of a synthetic dataset generation method for deep learning of real urban landscapes using a 3D model of a non-existing realistic city
ADVANCED ENGINEERING INFORMATICS(2023)
Abstract
In the urban landscaping field, training datasets for instance segmentation in the detection of building facades are needed for complex analysis and simulation based on data. Manual dataset generation methods are costly, so automatic generation is preferred. However, previous methods are costly and are more reliant on 3D real-city models than LOD2. The objective of this research is to propose a method for automatically generating synthetic datasets for instance segmentation using an image translation technique and procedural modeling. The image translation technique is used to generate street view images for training data, and procedural modeling can automatically specify the composition of generated street view images and generate annotation data, as well as a variety of 3D models. The proposed method generates various kinds of synthetic data at a rate of 4.45 s per set, and the model trained on the generated dataset can detect real buildings for each instance.
MoreTranslated text
Key words
Synthetic dataset, Automatic dataset generation, Instance segmentation, Image-to-image translation, Streetscape dataset, Computer-aided architectural design, Cityscape analysis
AI Read Science
Must-Reading Tree
Example
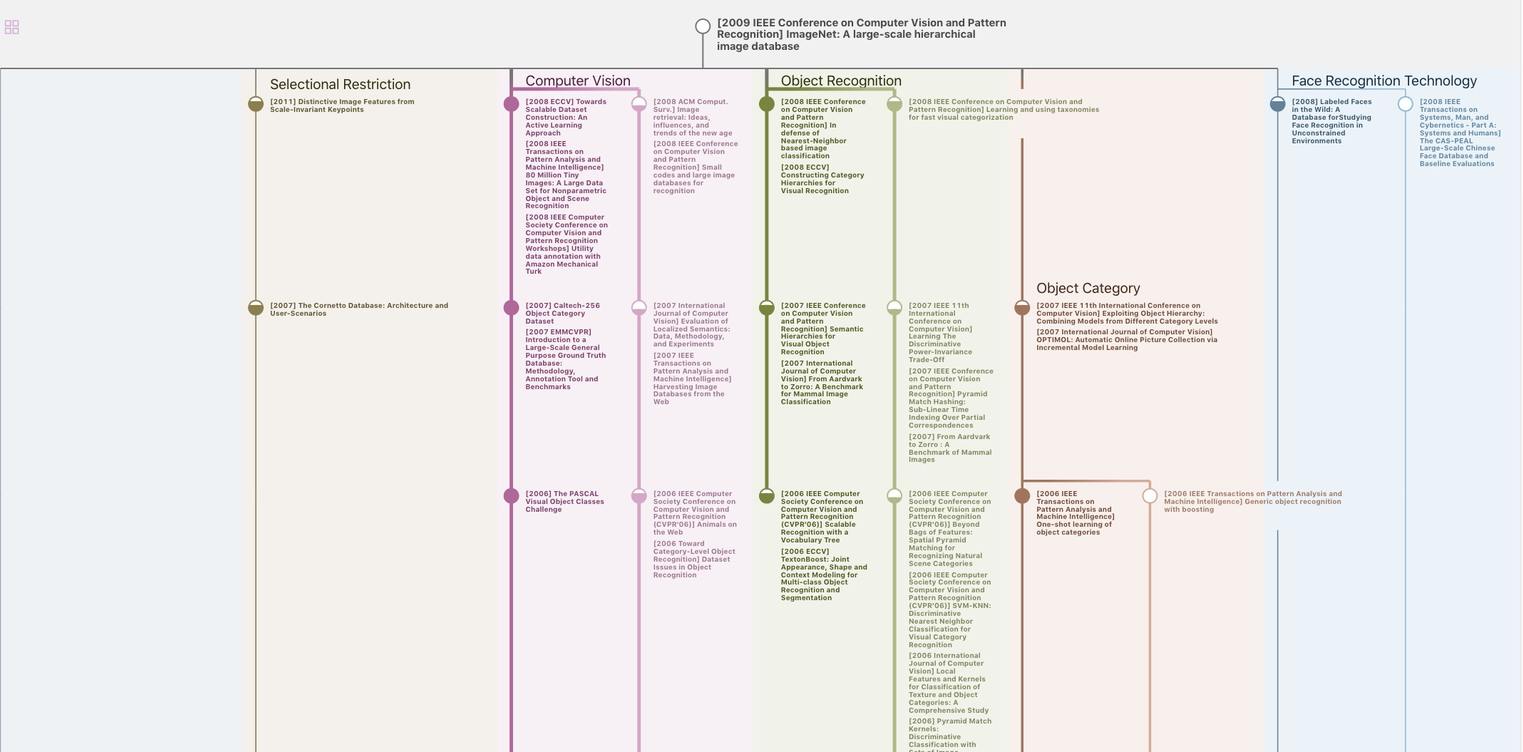
Generate MRT to find the research sequence of this paper
Chat Paper
Summary is being generated by the instructions you defined