Dynamic Pruning of Regions for Image-Sentence Matching
SIGNAL PROCESSING-IMAGE COMMUNICATION(2023)
摘要
Image-sentence matching is becoming increasingly essential in the integrated understanding of vision and language. Prior approaches apply a pre-trained detection model to extract region features and explore finegrained relationships between image and sentence by aggregating the similarities of all region-word pairs. However, all images are represented by the same number of regions, regardless of their respective semantic complexity, which results in a large number of redundant regions interfering with semantic inference and bringing additional computational burden. To address the lack of flexibility in image representation and information redundancy, a novel method named Dynamic Pruning of Regions for Image-Sentence Matching (DPRM) is proposed to efficiently capture relationships between text and image. In particular, a dynamic region pruning module is presented to dynamically select the appropriate number of regions according to the semantic complexity of each image, thus pruning redundant regions and reducing superfluous computations. Moreover, an inter-modality refinement module is designed to refine the fine-grained relationships of region-word pairs by retaining meaningful interaction features and suppressing interference from redundant alignments, which learns the more accurate semantic correspondences. Extensive experiments on MSCOCO and Flickr30K datasets prove the superiority of DPRM compared with previous approaches.
更多查看译文
关键词
image–sentence matching,dynamic pruning
AI 理解论文
溯源树
样例
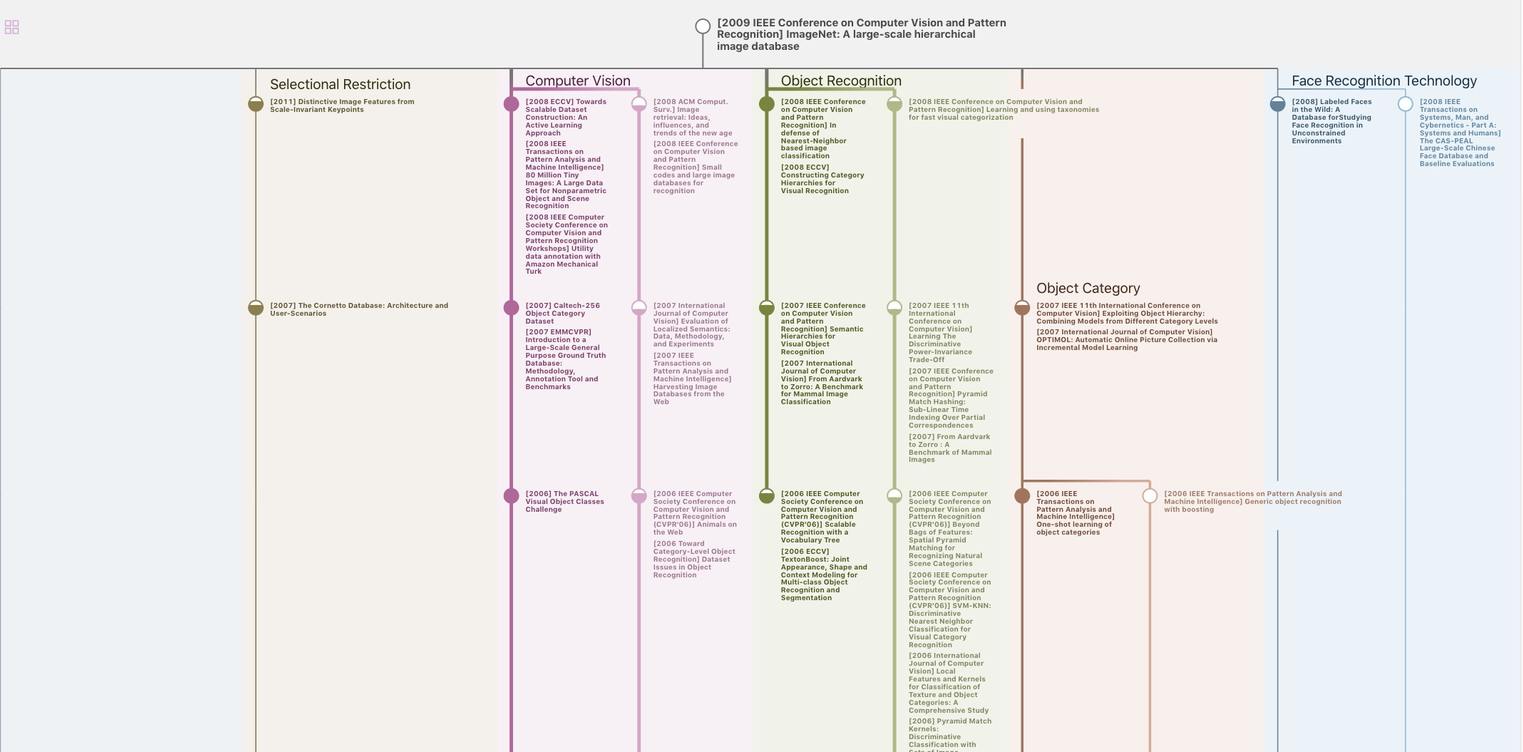
生成溯源树,研究论文发展脉络
Chat Paper
正在生成论文摘要