Merit: multi-level graph embedding refinement framework for large-scale graph
Complex & Intelligent Systems(2024)
摘要
The development of the Internet and big data has led to the emergence of graphs as an important data representation structure in various real-world scenarios. However, as data size increases, computational complexity and memory requirements pose significant challenges for graph embedding. To address this challenge, this paper proposes a multilevel embedding refinement framework (MERIT) based on large-scale graphs, using spectral distance-constrained graph coarsening algorithms and an improved graph convolutional neural network model that addresses the over-smoothing problem by incorporating initial values and identity mapping. Experimental results on large-scale datasets demonstrate the effectiveness of MERIT, with an average AUROC score 8% higher than other baseline methods. Moreover, in a node classification task on a large-scale graph with 126,825 nodes and 22,412,658 edges, the framework improves embedding quality while enhancing the runtime by 25 times. The experimental findings highlight the superior efficiency and accuracy of the proposed approach compared to other graph embedding methods.
更多查看译文
关键词
Graph representation learning,Graph embedding,Graph neural networks,Graph convolutional network,Large-scale graph
AI 理解论文
溯源树
样例
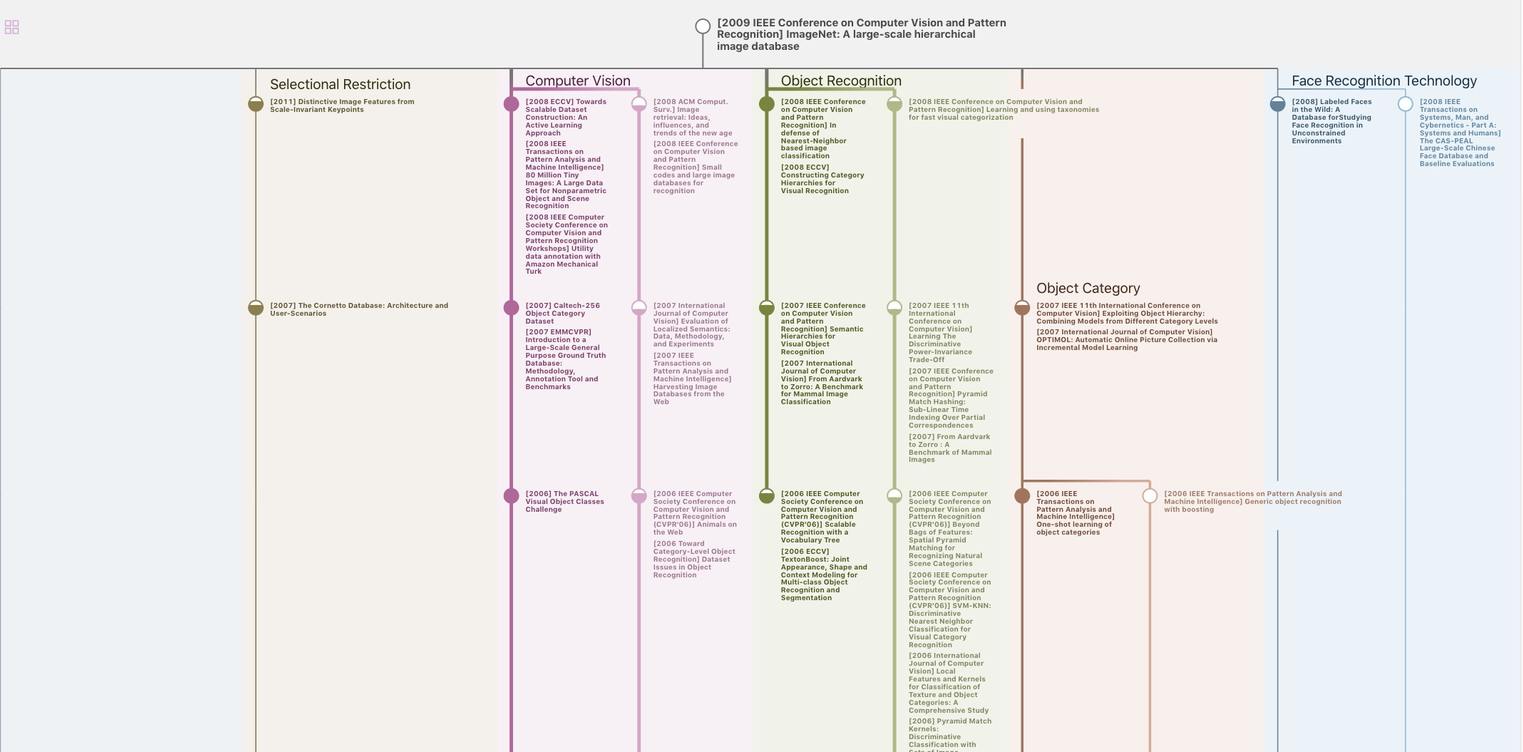
生成溯源树,研究论文发展脉络
Chat Paper
正在生成论文摘要