Using Deep Reinforcement Learning to Automate Network Configurations for Internet of Vehicles
IEEE TRANSACTIONS ON INTELLIGENT TRANSPORTATION SYSTEMS(2023)
摘要
In this paper, we address the issue of automating network configurations for dynamic network environments such as the Internet of Vehicles (IoV). Configuring network settings in IoV environments has proven difficult due to their dynamic and self-organizing nature. To address this issue, we propose a deep reinforcement learning-based approach to configure IoV network settings automatically. Specifically, we use a collection of neural networks to convert the observations of a communication environment (channel power gain, cross-channel power gain, etc.) into key features, which are then supplied to a deep Q neural network (DQN) as input for training. Afterward, the DQN will select the optimal network configuration for vehicles in the IoV environment. In addition, our approach considers both centralized and distributed training strategies. The centralized training strategy conducts the DQN training process on a roadside server, while the distributed training strategy trains the DQN on vehicles locally. Through our designed IoV simulation platform, we evaluate the efficacy of our proposed approach, demonstrating that it can improve the quality of services (QoS) in the IoV environments concerning reliability, latency, and service satisfaction.
更多查看译文
关键词
Internet of Vehicles,deep reinforcement learning
AI 理解论文
溯源树
样例
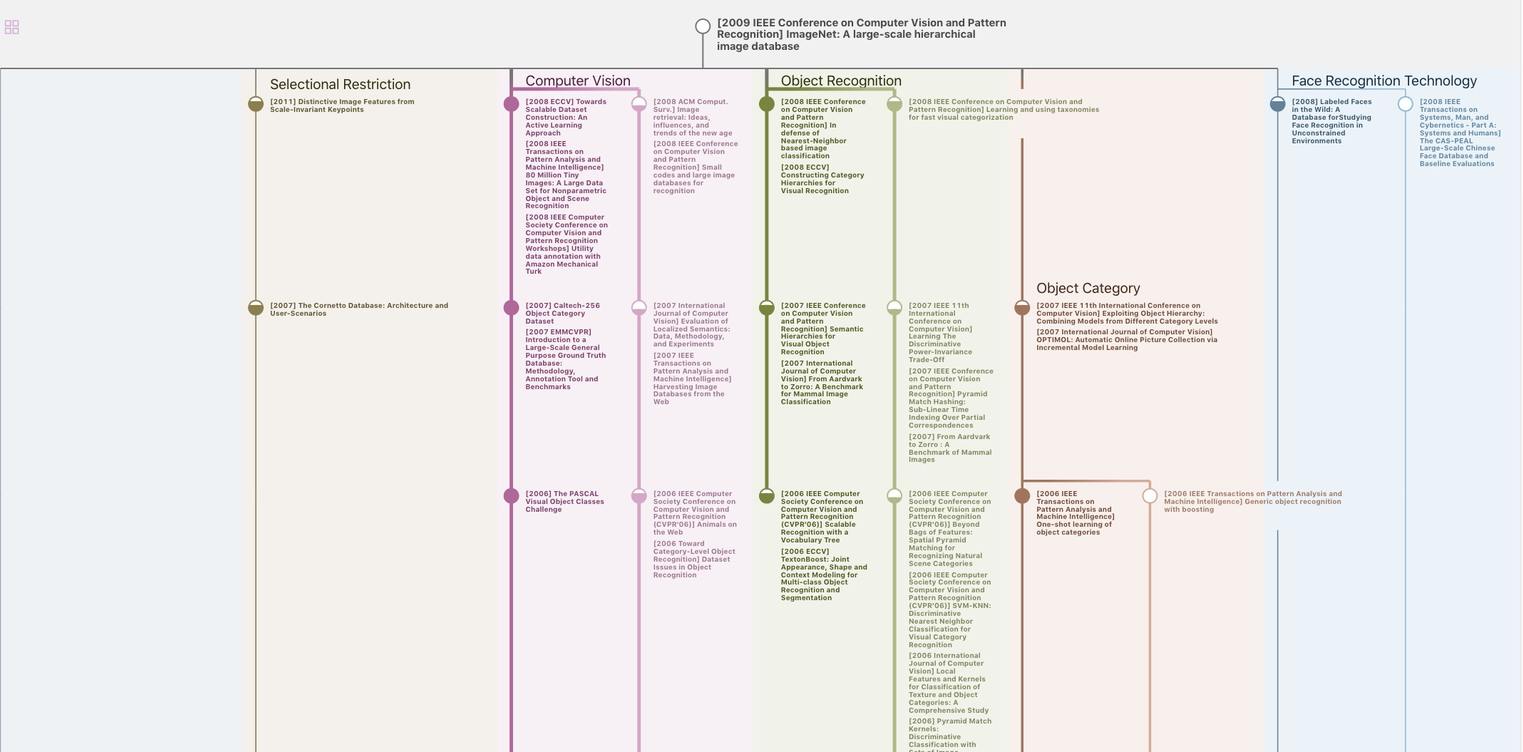
生成溯源树,研究论文发展脉络
Chat Paper
正在生成论文摘要