Modelling supra-classical logic in a Boltzmann neural network: III adaptation
JOURNAL OF LOGIC AND COMPUTATION(2023)
Abstract
The field of belief revision in logic is still in evolution and holds a variety of disparate approaches; a consequence of theoretical conjecture. As a probabilistic model of supra-classical, non-monotonic (SCNM) logic, the Boltzmann machine, offers an experimental gateway into the field. How does the Boltzmann network adapt to new information?Catastrophic forgetting is the default response to retraining in any neural network. We have moderated this irrational non-monotonicity by alterations in the Boltzmann learning algorithm. The spectrum of experimental belief change is limited by the availability of 'new' information, a pragmatic realization co-related to the property of Rational Monotonicity in the domain of SCNM logic. Recognizing this upper boundary of defeasible belief simplifies the task of experimentally exploring machine adaptation.A minority of belief revisions involve new, but unsurprising information, that is at least partially consistent with the previous learned beliefs. In these circumstances, the Boltzmann network incrementally adjusts the priority of model state exemplars in accordance with preference; the traditional approach in SCNM logic. However, in the majority of situations the new information will be surprisingly inconsistent with the previous beliefs. In these circumstances, the pre-order on model states stratified by preference, will not have sufficient granularity to represent the conflicting requirements of ranking based on compositional atomic typicality. This novel experimental finding has not previously been considered in the logical conjecture on Belief Revision.
MoreTranslated text
Key words
Boltzmann machine,supra-classical non-monotonic logic,preference relation,typicality,neural network,incongruence,belief revision,adaptation,prioritization
AI Read Science
Must-Reading Tree
Example
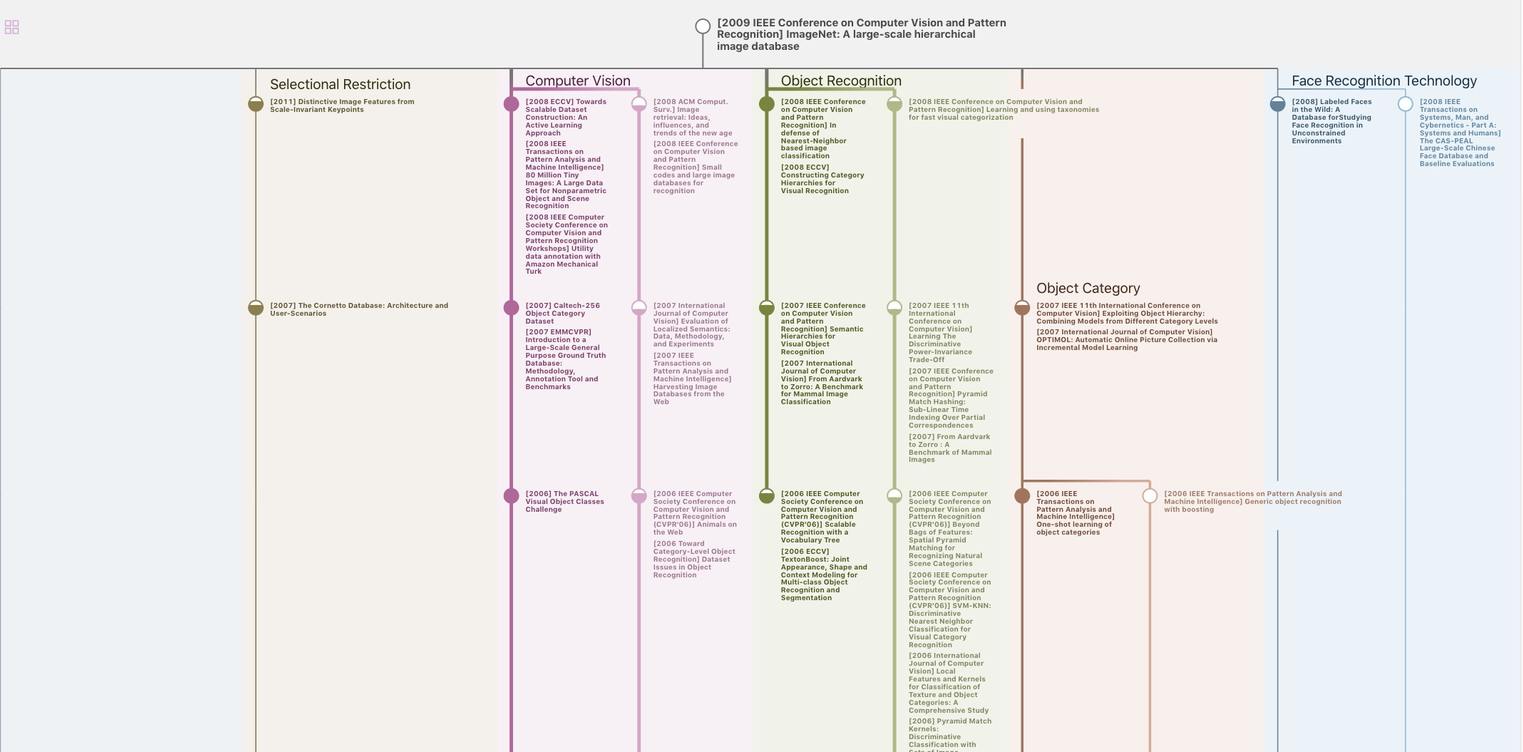
Generate MRT to find the research sequence of this paper
Chat Paper
Summary is being generated by the instructions you defined