Registration-enhanced multiple instance learning for cervical cancer whole slide image classification
INTERNATIONAL JOURNAL OF IMAGING SYSTEMS AND TECHNOLOGY(2024)
摘要
Histopathological diagnosis is the golden standard for cancer diagnosis and grading. The past decade has witnessed great successes of vision-based deep learning in computer-aided cancer diagnosis. However, fully-supervised methods require collecting large amounts of annotated data, which is a major challenge. In this research, we propose RMIL, a novel registration-enhanced multiple instance learning pipeline, for cervical cancer biopsy grading of whole slide images as well as patch-level regions. It requires only slide-level annotations and a small amount of patch-level annotations for training. To enhance it, an efficient registration method is implemented to generate more labeled patches with discriminative local features as data augmentations. In addition, attention mechanisms and self-supervised learning are introduced to enhance feature extraction. Experiments showed that its classification accuracy is increased by 11.11%, compared to the vanilla multiple instance learning methods. The method has great potential for transferring to other scenarios.
更多查看译文
关键词
cervical cancer grading,image registration,multiple instance learning,self-attention mechanisms,self-supervised learning
AI 理解论文
溯源树
样例
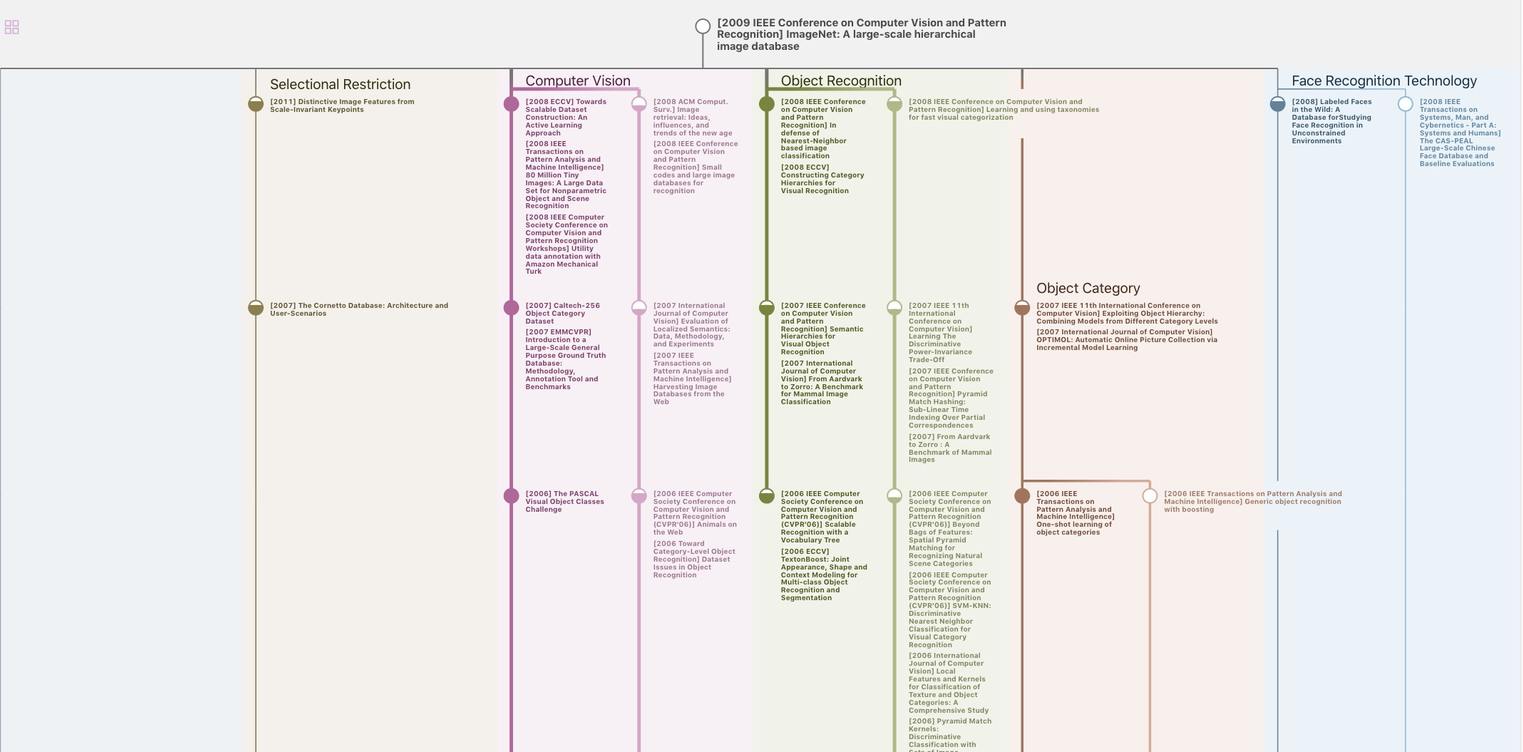
生成溯源树,研究论文发展脉络
Chat Paper
正在生成论文摘要