Spectral Detection of Peanut Southern Blight Severity Based on Continuous Wavelet Transform and Machine Learning
AGRICULTURE-BASEL(2023)
摘要
Peanut southern blight has a severe impact on peanut production and is one of the most devastating soil-borne fungal diseases. We conducted a hyperspectral analysis of the spectral responses of plants to peanut southern blight to provide theoretical support for detecting the severity of the disease via remote sensing. In this study, we collected leaf-level spectral data during the winter of 2021 and the spring of 2022 in a greenhouse laboratory. We explored the spectral response mechanisms of diseased peanut leaves and developed a method for assessing the severity of peanut southern blight disease by comparing the continuous wavelet transform (CWT) with traditional spectral indices and incorporating machine learning techniques. The results showed that the SVM model performed best and was able to effectively detect the severity of peanut southern blight when using CWT (WF770 similar to 780, 5) as an input feature. The overall accuracy (OA) of the modeling dataset was 91.8% and the kappa coefficient was 0.88. For the validation dataset, the OA was 90.5% and the kappa coefficient was 0.87. These findings highlight the potential of this CWT-based method for accurately assessing the severity of peanut southern blight.
更多查看译文
关键词
peanut southern blight,reflection spectrum,spectral index,continuous wavelet transform,machine learning
AI 理解论文
溯源树
样例
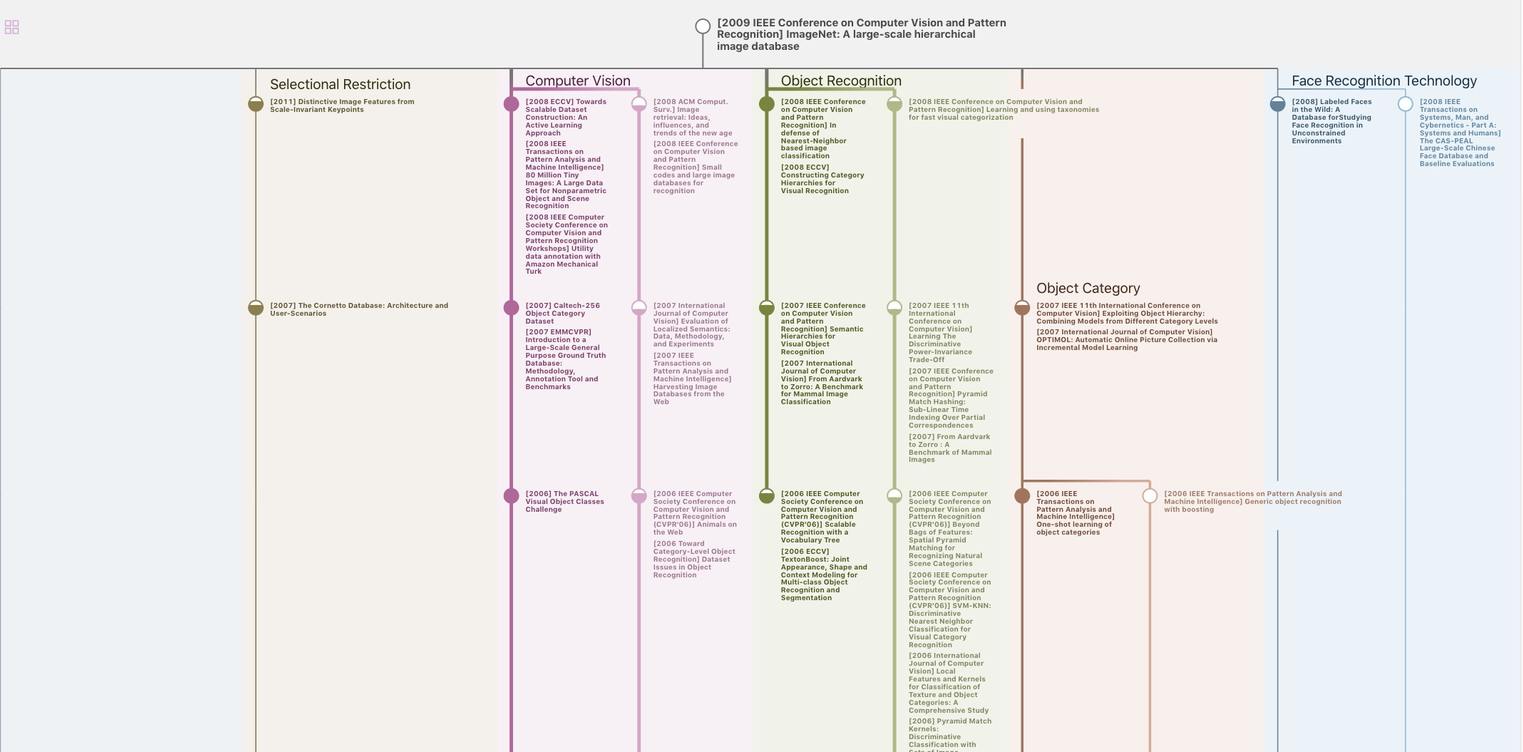
生成溯源树,研究论文发展脉络
Chat Paper
正在生成论文摘要