Grassland rat-hole recognition and classification based on attention method and unmanned aerial vehicle hyperspectral remote sensing
INMATEH-AGRICULTURAL ENGINEERING(2023)
摘要
Rat-hole area and number of rat holes are indicators of the level of degradation and rat damage in grassland environments. However, rat-hole monitoring has consistently relied on manual ground surveys, leading to extremely low efficiency and accuracy. In this paper, a convolutional block attention module (CBAM) model suitable for rat-hole recognition in desert grassland monitoring, called grassland monitoring-CBAM, is proposed that comprehensively incorporates unmanned aerial vehicle hyperspectral remote-sensing technology and deep-learning methods. Validation results show that the overall accuracy and Kappa coefficient of the model were 99.35% and 98.90%, which were 3.96% and 3.35% higher, respectively, than those of the basic model. This study represents a breakthrough in the intelligent interpretation of rat holes and provides technical support for the subsequent rapid interpretation of grassland rat holes and rat damage evaluation. It also provides a solution for the fine classification and quantitative inversion of similar landscape features.
更多查看译文
关键词
3D convolutional neural networks, Attention mechanism, Rat-hole identification, UAV hyperspectral remote sensing
AI 理解论文
溯源树
样例
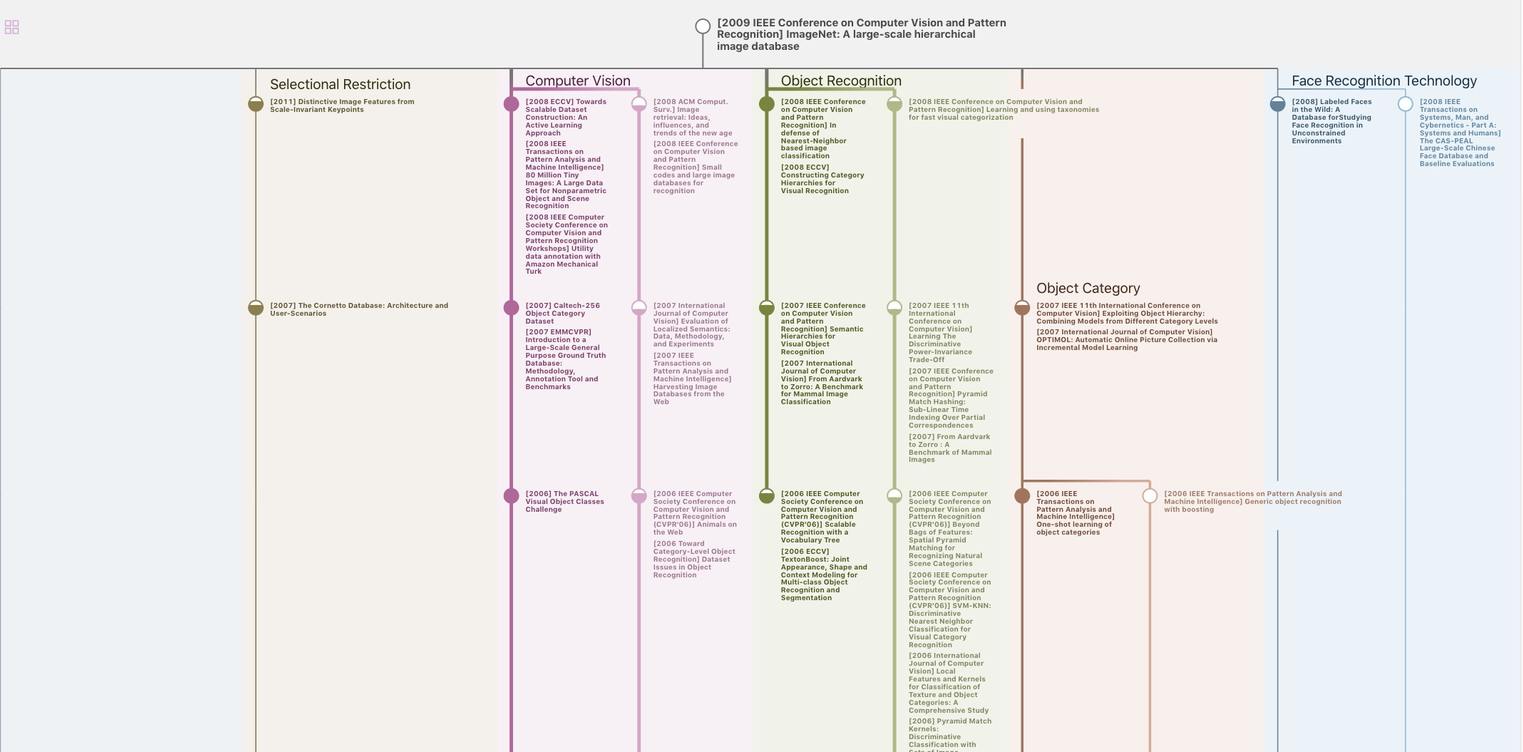
生成溯源树,研究论文发展脉络
Chat Paper
正在生成论文摘要