A Three-Dimensional Hail Trajectory Clustering Technique
MONTHLY WEATHER REVIEW(2023)
摘要
Recent advances in hail trajectory modeling regularly produce datasets containing millions of hail trajectories. Because hail growth within a storm cannot be entirely separated from the structure of the trajectories producing it, a method to condense the multidimensionality of the trajectory information into a discrete number of features analyzable by humans necessary. This article presents a three-dimensional trajectory clustering technique that is designed to group trajectories that have similar updraft-relative structures and orientations. The new technique is an application of a two-dimensional method common in the data mining field. Hail trajectories (or "parent" trajectories) are partitioned into segments before they are clustered using a modified version of the density-based spatial applications with noise (DBSCAN) method. Parent trajectories with segments that are members of at least two common clusters are then grouped into parent trajectory clusters before out-put. This multistep method has several advantages. Hail trajectories with structural similarities along only portions of their length, e.g., sourced from different locations around the updraft before converging to a common pathway, can still be grouped. However, the physical information inherent in the full length of the trajectory is retained, unlike methods that clus-ter trajectory segments alone. The conversion of trajectories to an updraft-relative space also allows trajectories separated time to be clustered. Once the final output trajectory clusters are identified, a method for calculating a representative trajec-tory for each cluster is proposed. Cluster distributions of hailstone and environmental characteristics at each time step in the representative trajectory can also be calculated. SIGNIFICANCE STATEMENT: To understand how a storm produces large hail, we need to understand the paths that hailstones take in a storm when growing. We can simulate these paths using computer models. However, the millions of hailstones in a simulated storm create millions of paths, which is hard to analyze. This article describes a ma-chine learning method that groups together hailstone paths based on how similar their three-dimensional structures look. It will let hail scientists analyze hailstone pathways in storms more easily, and therefore better understand how hail growth happens.
更多查看译文
关键词
clustering,three-dimensional
AI 理解论文
溯源树
样例
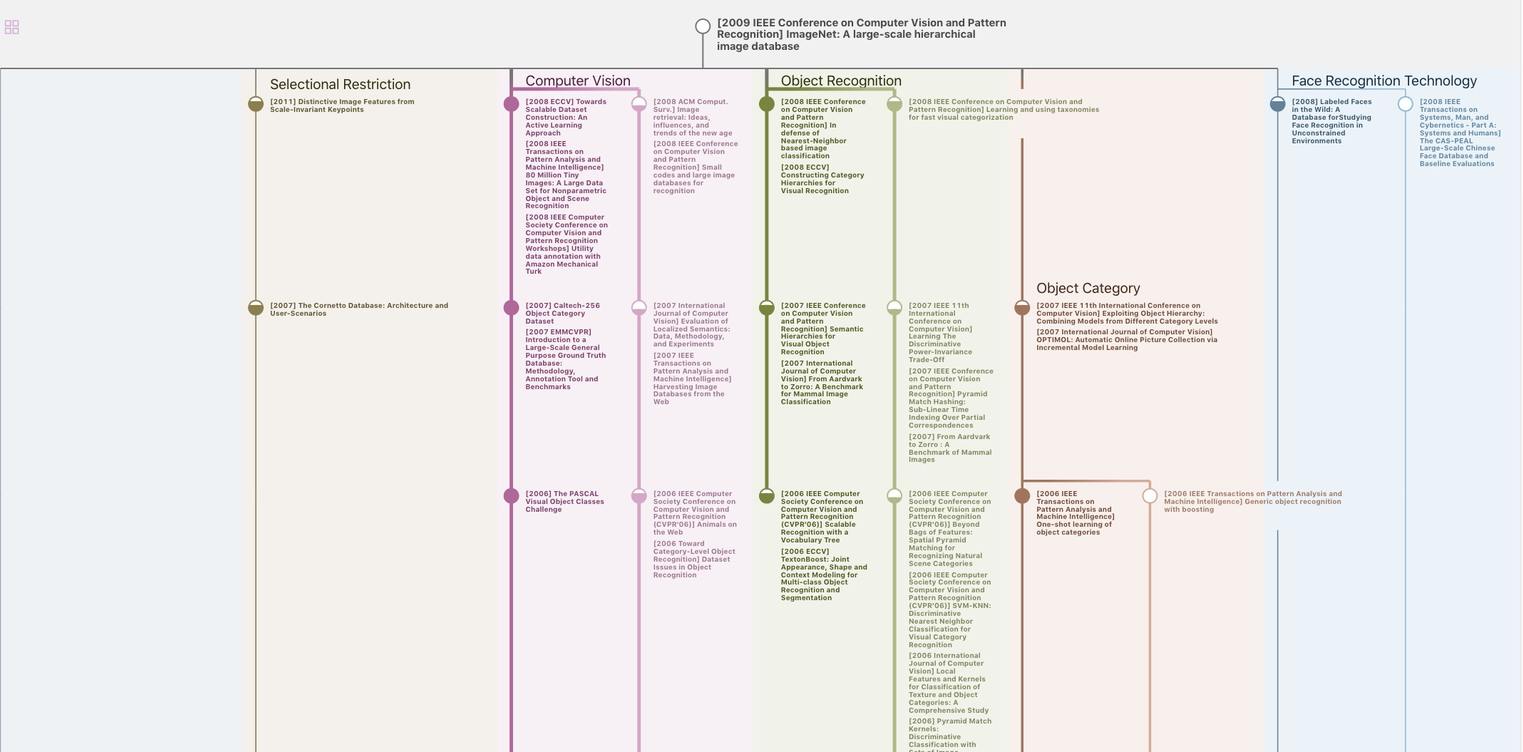
生成溯源树,研究论文发展脉络
Chat Paper
正在生成论文摘要