AI-based identification of FGFR3 mutation status from routine histology slides of muscle-invasive bladder cancer
JOURNAL OF CLINICAL ONCOLOGY(2023)
摘要
e16580 Background: Gain of function mutations of the FGFR3 gene have been reported to be important driver mutations in a subset of muscle-invasive bladder urothelial cancer (MIBC). Subsequently, gene specific kinase inhibitors (e.g., erdafitinib) have been developed to target FGFR3 mutant metastasized MIBC with promising clinical activity. FGFR3 mutational status testing is required for erdafitinib treatment, but FGFR3 mutations are rare in metastatic MIBC (10-15%). Thus, cheap and fast pre-screening tools such as artificial intelligence (AI) based image classifiers could help to reduce turnover times of FGFR3 mutational testing and save costs arising from universal mutational testing. Herein, we propose an AI-based approach to identify MIBC FGFR3 mutated patients on routine histological slides. Methods: Slides from 612 patients with MIBC from two different sources (CCC-EMN Erlangen cohort n = 239, mutant = 20; TCGA-BLCA n = 373, mutant = 50) were used. The CCC-EMN cohort was used as a discovery set while TCGA-BLCA was used as an independent validation set. A two-step AI model was used to 1) extract features from each slide and 2) predict FGFR3 status from these features. The AUC, sensitivity and specificity were used as metrics to assess the performance of our model. Most predictive regions of FGFR3 mutation were reviewed by an expert pathologist. Results: The model reached an AUC/Sensitivity/Specificity of 0.82 [0.76-0.87] / 0.90 [0.82-0.97] / 0.57 [0.53-0.62] on TCGA-BLCA, substantially improving upon results previously reported on the same cohort (Loeffler et al. 2021: AUC = 0.70). Most predictive regions of FGFR3 mutation revealed monomorphous conventional urothelial tumor morphology and low desmoplastic tumor stroma content, while least predictive regions contained pleomorphic tumor morphology and high amounts of desmoplastic tumor content. Conclusions: By ruling out 57% of MIBC FGFR3 wild type tumors with high sensitivity, our model is the first to reach valuable performance for clinical use. Consequently, this study paves the way for the development of FGFR3 pre-screening tools to ease the management of patients with MIBC.
更多查看译文
关键词
fgfr3 mutation status,cancer,ai-based,muscle-invasive
AI 理解论文
溯源树
样例
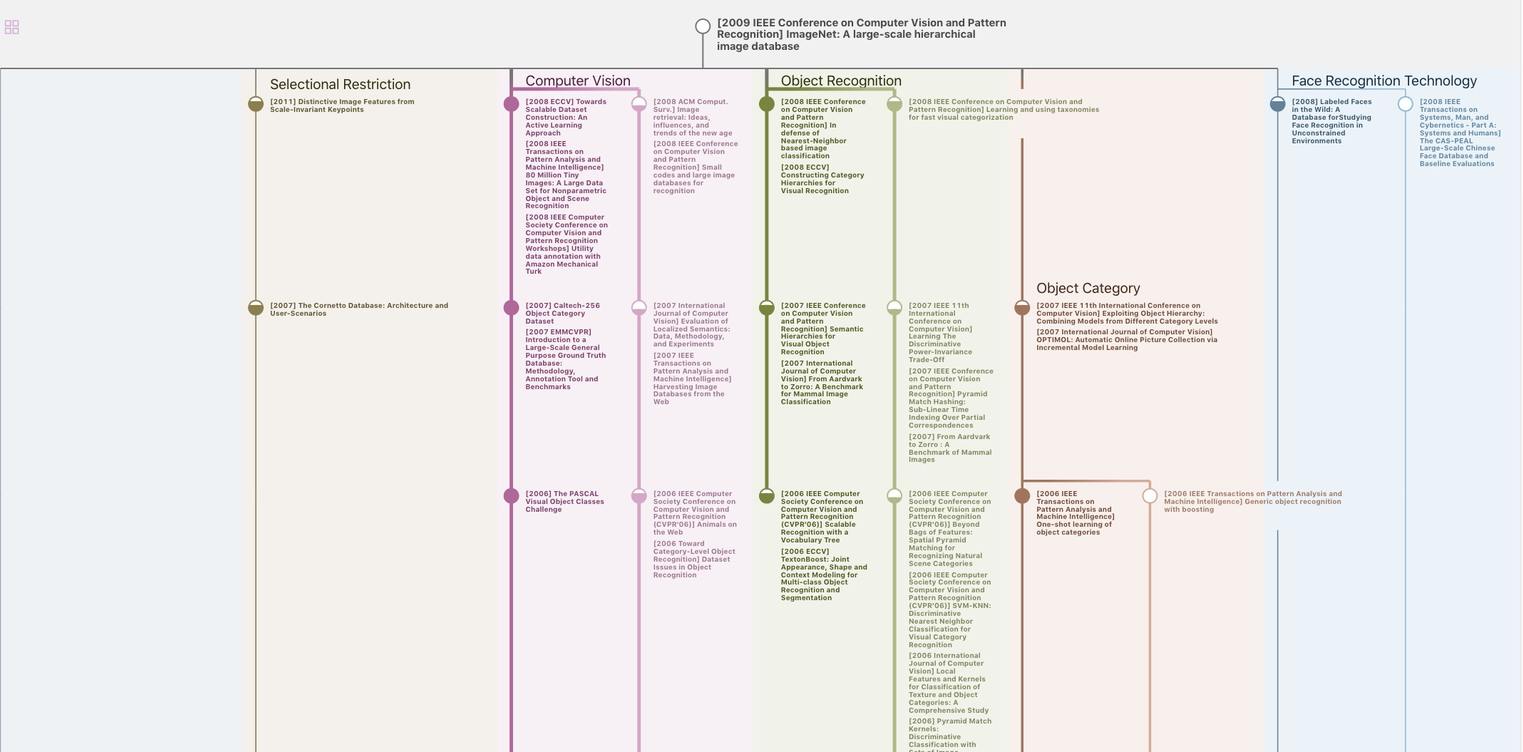
生成溯源树,研究论文发展脉络
Chat Paper
正在生成论文摘要