ALAN as an omic tool to build gene signatures and to stratify patients
JOURNAL OF CLINICAL ONCOLOGY(2023)
摘要
1563 Background: Understanding aberrant gene behavior is critical towards devising clinical therapeutics or gene based diagnostic panels. Clustering and AI/ML have clinical utility, but they do not contextualize changes in gene behavior that result from differences in cell type, microenvironment, or prior treatments. Here, we developed the Algorithm for Linking Activity Networks (ALAN), an informatics algorithm that allows rapid functional comparisons of all detectable genes between clinically annotated patient cohorts. ALAN outputs quantify gene behavior without any additional inputs and can be applied on all forms of clinical omic data. We tested the utility of ALAN to identify the molecular features of resistance and novel precision therapeutics. Methods: We applied the ALAN algorithm in prostate tissue using whole-transcriptome sequencing (WTS) data from SU2C/PCF (n = 209, metastatic prostate cancer), TCGA (n = 493, primary prostate cancer), and GTEX (n = 245, benign prostate tissue). We conducted informatics analysis of whole-exome sequencing (WES) data from SU2C/PCF (n = 444 mPC), Fred Hutch (n = 130 mPC), and MCTP (n = 61 mPC) to identify mPC patients with amplifications in RSPO2. We scored an ALAN gene signature in a patient with single-cell RNA sequencing pre and post AR inhibition (n = 112, 83). We examined ChIP-seq data to evaluate binding of AR and Co-factors at the CD276 transcription regulatory sequences. Results: In mPCs, ALAN unbiasedly identified genes that prior studies have implicated as resistance mechanisms towards enzalutamide. In this process, ALAN nominated RSPO2, a secreted protein, as a potential resistant gene that has never been targeted in mPC. In recent studies, we found RSPO2 amplifications in 19.7-28.5% of mPC. We utilized ALAN outputs to build a novel gene signature that was exclusively enriched (p-value < 0.0001) in the transcriptome collected from single cells of a post-resistant mPC sample. We also utilized ALAN to stratify the optimal mPC patients in receiving precision therapies against B7-H3 ( CD276). B7-H3 is an immune regulatory molecule in the B7 superfamily that includes PD-L1 and CTLA-4 and has not been targeted in mPC. B7-H3 was unlike other B7 family members and exhibited robust association with AR co-factors and AR signaling. We confirmed ALAN findings as multiple B7-H3 transcriptional enhancers and promoters were bound by AR and AR-cofactors. Conclusions: ALAN is an algorithm that can be readily deployed on existing clinical omic data to build gene signatures, identify resistant genes, and risk stratify patients. ALAN nominated a novel therapeutic target, RSPO2, which is recurrently amplified in mPC patients. ALAN predicted that mPCs with high baseline levels of AR activity would be optimal candidates for B7-H3 precision therapeutics. Altogether, ALAN outputs should be considered for making clinical decisions that would benefit by comprehensive comparisons of all detectable genes.
更多查看译文
关键词
gene signatures,omic tool,patients
AI 理解论文
溯源树
样例
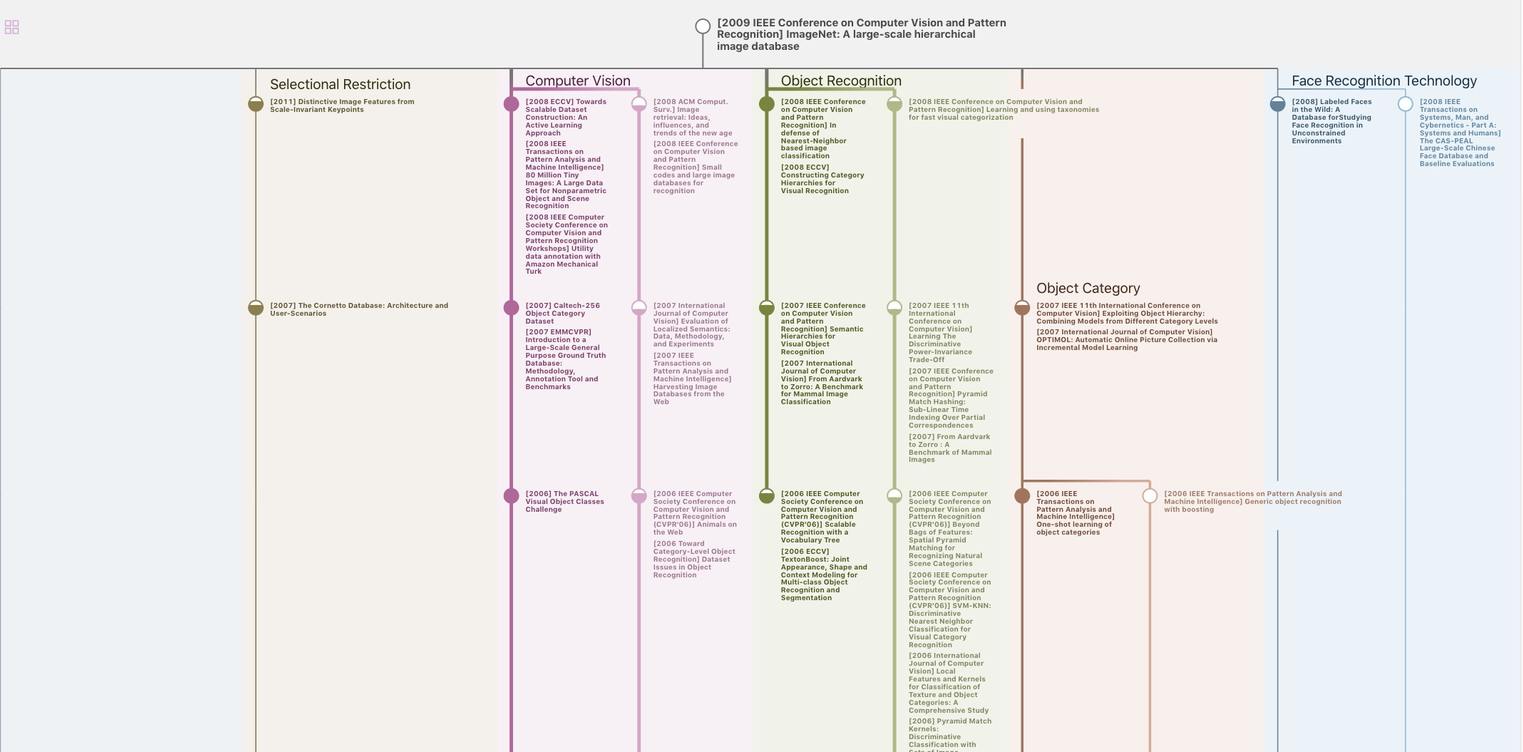
生成溯源树,研究论文发展脉络
Chat Paper
正在生成论文摘要