Using machine learning-based boosting algorithm to predict hepatotoxicity after local therapy and association with survival in patients undergoing liver directed therapy
JOURNAL OF CLINICAL ONCOLOGY(2023)
摘要
e16229 Background: Hepatocellular carcinoma typically arises in the setting of chronic liver disease. In carefully selected patients, liver directed therapy has been demonstrated to improve survival; however, this benefit from cancer specific survival may be offset by increased mortality from diminished hepatic functional reserve. Treatment-related hepatotoxicity also may vary between liver directed treatment modalities for a given tumor. Our aim was to determine if a machine learning approach could estimate hepatotoxicity prior to treatment. Methods: We identified all patients with hepatocellular carcinoma who underwent liver directed therapy at a large urban academic health system who also had post-treatment laboratory follow-up at 6 months. Patients were categorized as having worse liver function if they experienced a decline in albumin-bilirubin (ALBI) grade or more than 0.5 absolute decline in ALBI score at post treatment follow-up. A machine learning, extreme-gradient boosting (XGBoost) approach was employed to identify predictive features for hepatotoxicity using fine-tuned hyperparameters. Results: The study cohort consisted of 269 patients. 48 (18%) were categorized as having worsened hepatic function after liver directed therapy, with an average decline of -0.72 in ALBI score. Median survival after liver directed therapy was 5.8 years, with worsening hepatic function associated with a 5 month decline in overall survival. 158 (59%) patients received transarterial chemoembolization, 99 (37%) patients received stereotactic body radiotherapy and 12 (7%) patients received Y-90. A machine learning model was developed resulting in an Area Under the ROC Curve (AUC) of 0.894 in a 10-point decision tree model. On SHAP analysis, the features with the largest impact on hepatotoxicity prior to treatment was pretreatment T-stage followed by Child-Pugh Score. Conclusions: A machine learning model was developed to accurately classify patients who sustained treatment related hepatotoxicity. Significant decline in ALBI score or grade was associated with a worsening of overall survival. Future studies in larger prospective clinical trials utilizing this machine learning-based methodology of assessing liver dysfunction after focal therapy are required to validate these findings.
更多查看译文
关键词
hepatotoxicity,liver,learning-based
AI 理解论文
溯源树
样例
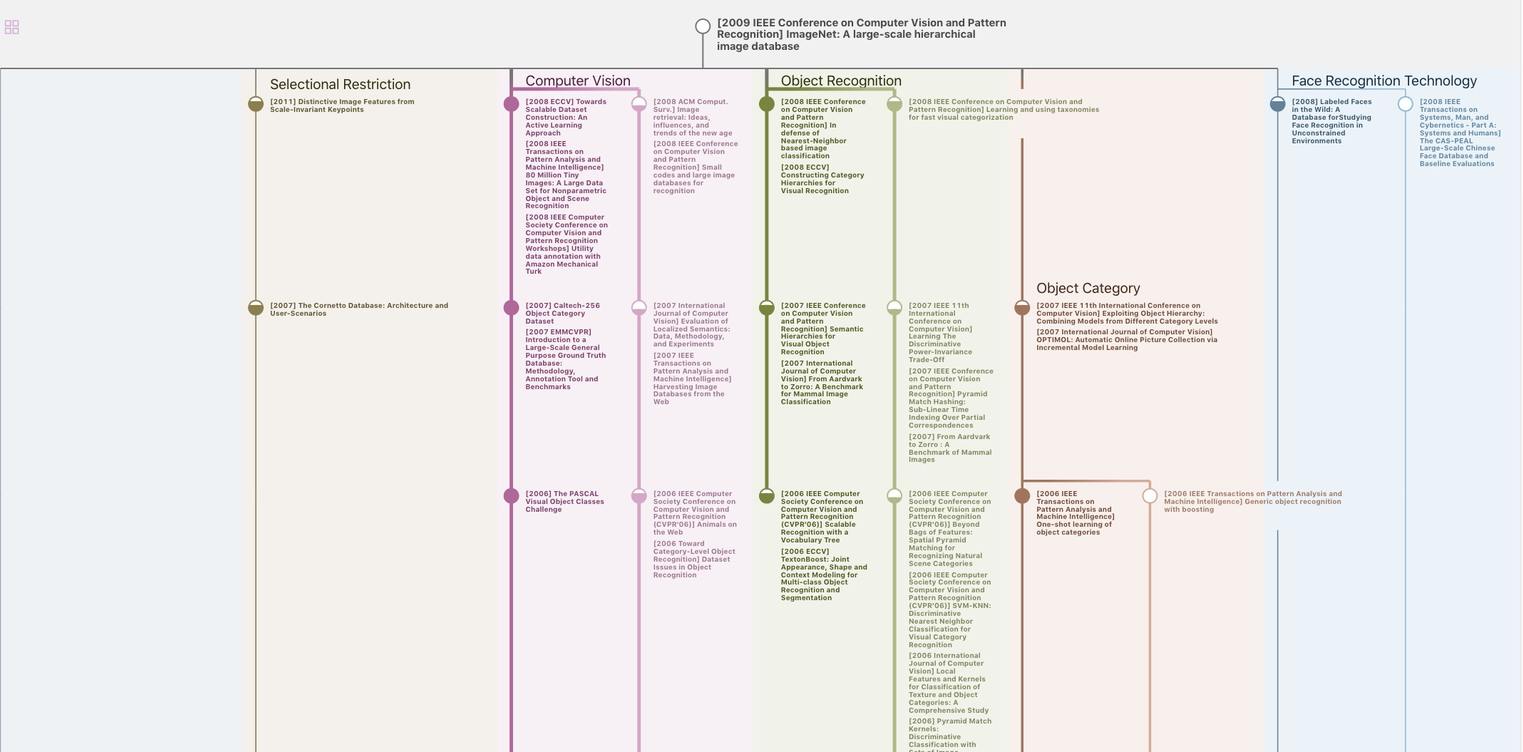
生成溯源树,研究论文发展脉络
Chat Paper
正在生成论文摘要