Bayesian ridge estimators based on copula-based joint prior distributions for logistic regression parameters
COMMUNICATIONS IN STATISTICS-SIMULATION AND COMPUTATION(2023)
摘要
Ridge regression was originally proposed as an alternative to ordinary least-squares regression to address multicollinearity in linear regression and was later extended to logistic and Cox regressions. The ridge estimator is interpreted as the Bayesian posterior mean or median in the Bayesian framework when the regression coefficients have multivariate normal priors. We previously proposed using vine copula-based joint priors on regression coefficients in linear and Cox regressions, including an interaction that promotes the use of ridge regression because the interaction term can result in multicollinearity. We showed that vine copula-based priors improve the estimation accuracy over the multivariate normal prior, and they would be a promising approach in other regression types, such as logistic regression. In this study, we focus on a case involving two covariates and their interaction terms, and propose a vine copula-based prior for Bayesian ridge estimators under a logistic model. Simulation and data analysis results show that Archimedean (Clayton and Gumbel) copula priors are superior to other priors (the Gaussian copula and trivariate normal priors) in the presence of multicollinearity.
更多查看译文
关键词
Logistic regression, Ridge regression, Vine copula, Multicollinearity, Interaction term
AI 理解论文
溯源树
样例
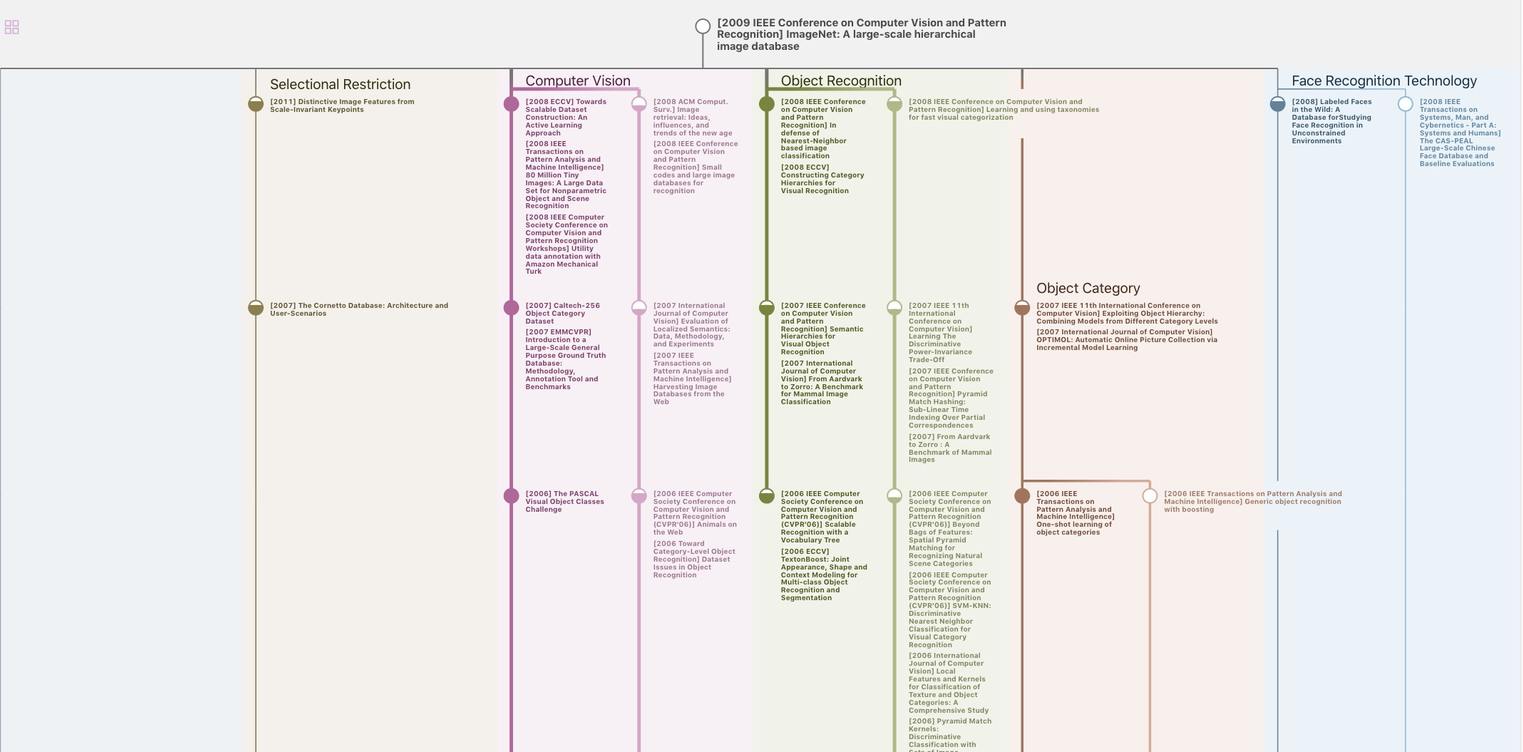
生成溯源树,研究论文发展脉络
Chat Paper
正在生成论文摘要