Encoder-Decoder Structure Fusing Depth Information for Outdoor Semantic Segmentation
APPLIED SCIENCES-BASEL(2023)
摘要
The semantic segmentation of outdoor images is the cornerstone of scene understanding and plays a crucial role in the autonomous navigation of robots. Although RGB-D images can provide additional depth information for improving the performance of semantic segmentation tasks, current state-of-the-art methods directly use ground truth depth maps for depth information fusion, which relies on highly developed and expensive depth sensors. Aiming to solve such a problem, we proposed a self-calibrated RGB-D image semantic segmentation neural network model based on an improved residual network without relying on depth sensors, which utilizes multi-modal information from depth maps predicted with depth estimation models and RGB image fusion for image semantic segmentation to enhance the understanding of a scene. First, we designed a novel convolution neural network (CNN) with an encoding and decoding structure as our semantic segmentation model. The encoder was constructed using IResNet to extract the semantic features of the RGB image and the predicted depth map and then effectively fuse them with the self-calibration fusion structure. The decoder restored the resolution of the output features with a series of successive upsampling structures. Second, we presented a feature pyramid attention mechanism to extract the fused information at multiple scales and obtain features with rich semantic information. The experimental results using the publicly available Cityscapes dataset and collected forest scene images show that our model trained with the estimated depth information can achieve comparable performance to the ground truth depth map in improving the accuracy of the semantic segmentation task and even outperforming some competitive methods.
更多查看译文
关键词
semantic segmentation,RGB-D image,predicted depth map,fusion structure,feature pyramid
AI 理解论文
溯源树
样例
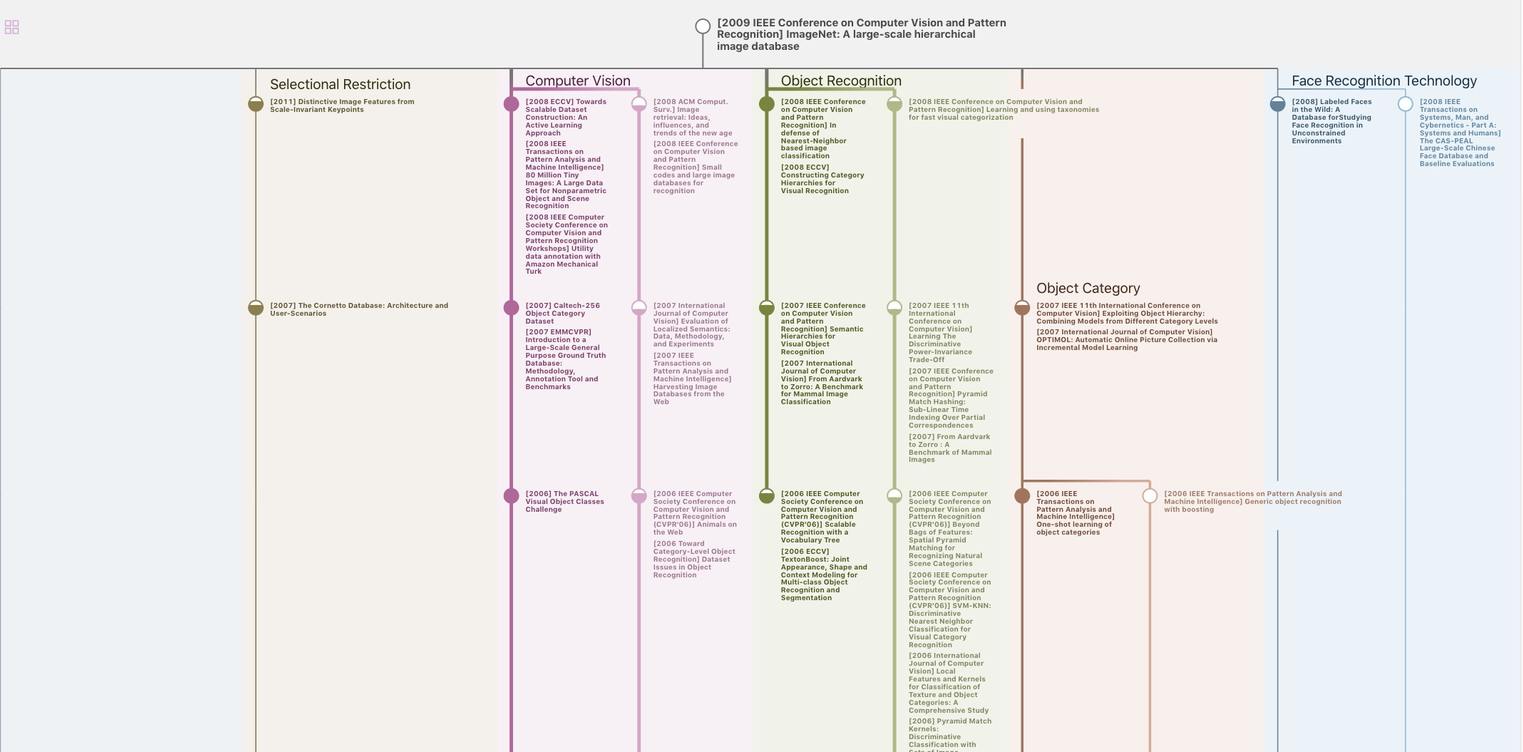
生成溯源树,研究论文发展脉络
Chat Paper
正在生成论文摘要