Condition Monitoring Method for the Gearboxes of Offshore Wind Turbines Based on Oil Temperature Prediction
ENERGIES(2023)
摘要
Traditional machine learning prediction methods usually only predict input parameters through a single model, so the problem of low prediction accuracy is common. Different predictive models extract different information for input, and combining different predictive models can make as much use as possible of all the information contained in the inputs. Therefore, this paper improves the existing oil temperature prediction method of offshore wind turbine gearboxes, and for the actual prediction effect of Supervisory Control And Data Acquisition (SCADA) data in this paper, Bayesian-optimized Light Gradient Boosting Machine (LightGBM) and eXtreme Gradient Boosting(XGBoost) machine learning models are selected to be combined. A method based on the Induced Ordered Weighted Average (IOWA) operator combination prediction model is thus proposed, with simulation results showing that the proposed model improves the accuracy of gearbox condition monitoring. The innovation of this article lies in considering the various negative impacts faced by actual offshore wind turbines and adopting a combination prediction model to improve the accuracy of gearbox condition monitoring.
更多查看译文
关键词
SCADA,fault diagnosis,LightGBM,XGBoost,IOWA
AI 理解论文
溯源树
样例
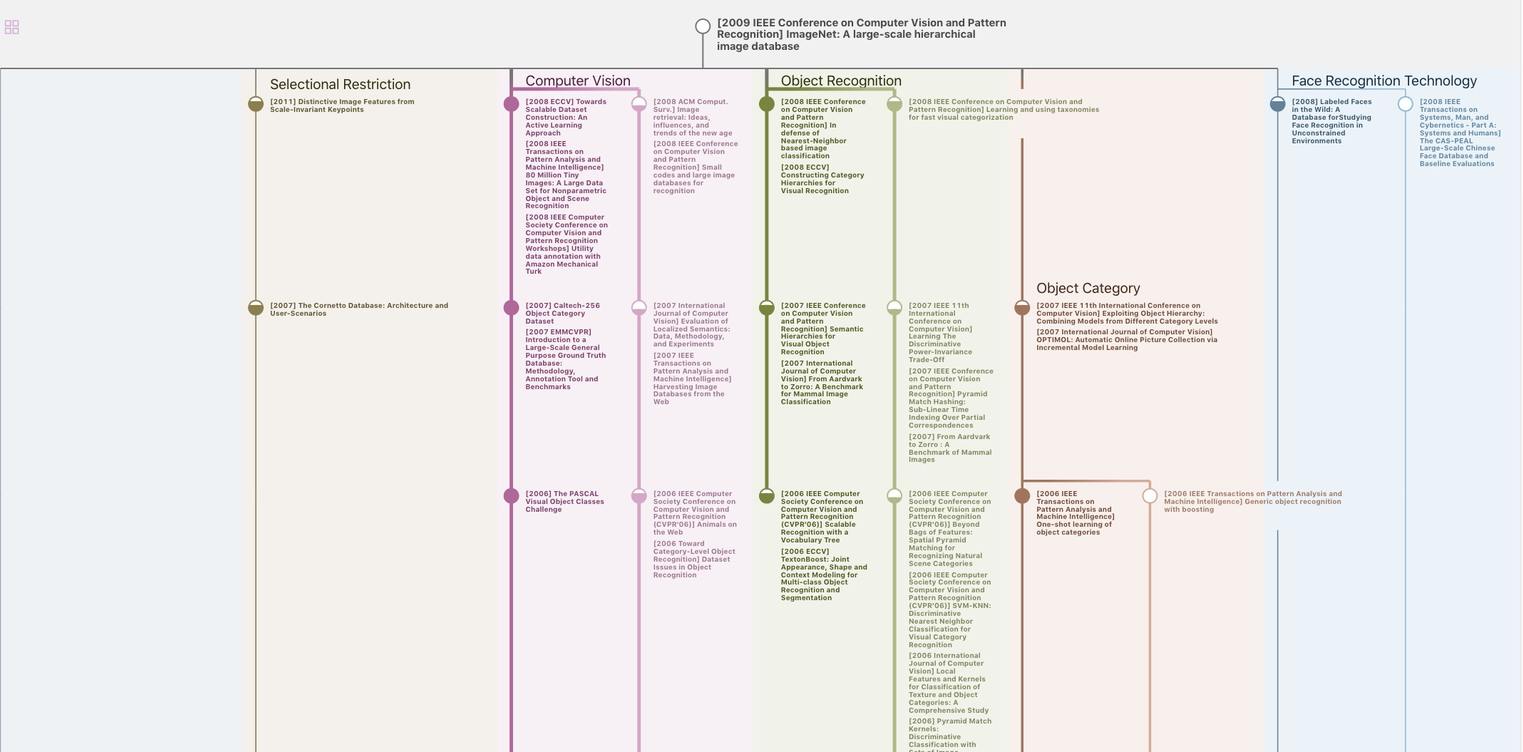
生成溯源树,研究论文发展脉络
Chat Paper
正在生成论文摘要