A Cross-Modal Dynamic Attention Neural Architecture to Detect Anomalies in Data Streams from Smart Communication Environments
APPLIED SCIENCES-BASEL(2023)
摘要
Detecting anomalies in data streams from smart communication environments is a challenging problem that can benefit from novel learning techniques. The Attention Mechanism is a very promising architecture for addressing this problem. It allows the model to focus on specific parts of the input data when processing it, improving its ability to understand the meaning of specific parts in context and make more accurate predictions. This paper presents a Cross-Modal Dynamic Attention Neural Architecture (CM-DANA) by expanding on state-of-the-art techniques. It is a novel dynamic attention mechanism that can be trained end-to-end along with the rest of the model using multimodal data streams. The attention mechanism calculates attention weights for each position in the input data based on the model's current state by a hybrid method called Cross-Modal Attention. Specifically, the proposed model uses multimodal learning tasks where the input data comes from different cyber modalities. It combines the relevant input data using these weights to produce an attention vector in order to detect suspicious abnormal behavior. We demonstrate the effectiveness of our approach on a cyber security anomalies detection task using multiple data streams from smart communication environments.
更多查看译文
关键词
cross-modal learning tasks, dynamic attention mechanism, neural architecture, anomaly detection, data streams, smart communication environments
AI 理解论文
溯源树
样例
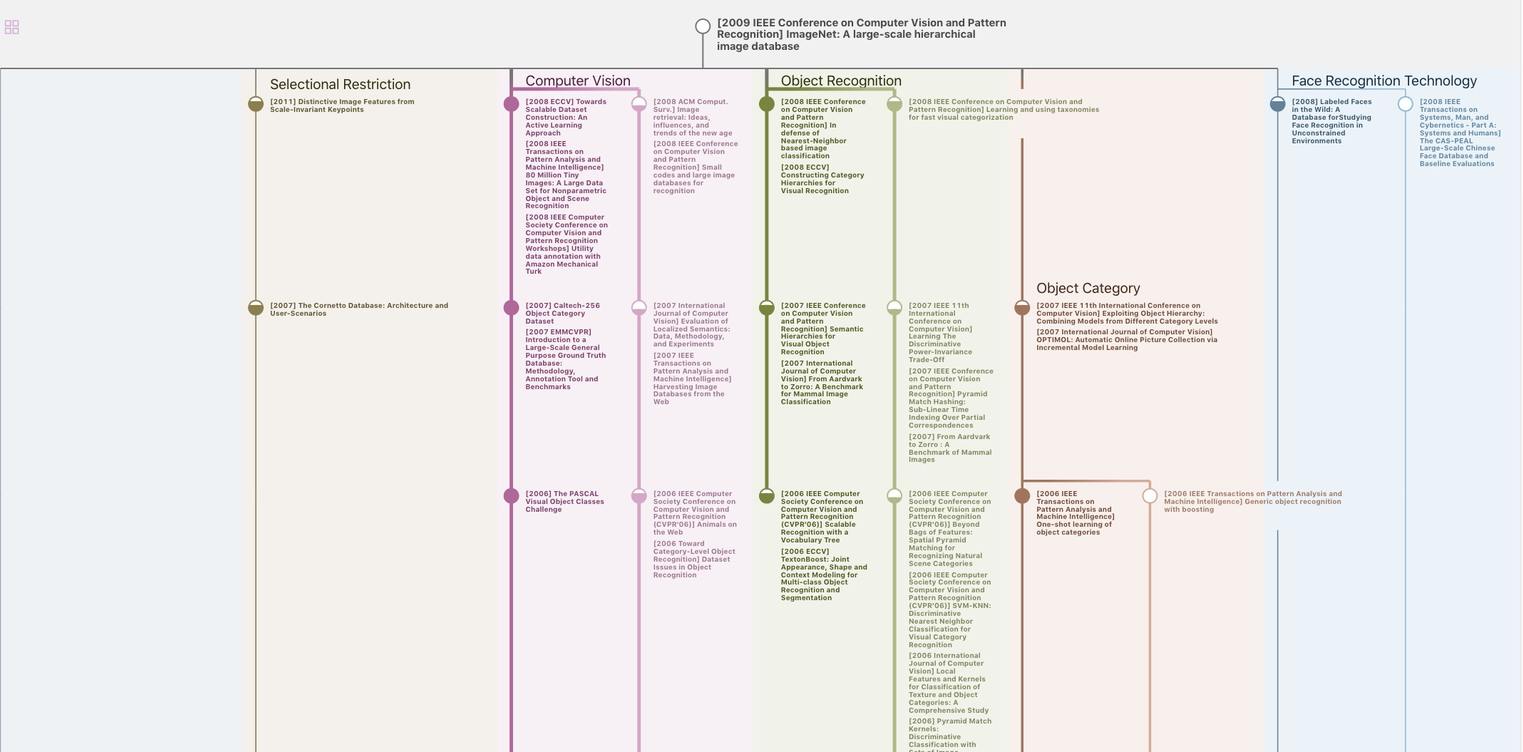
生成溯源树,研究论文发展脉络
Chat Paper
正在生成论文摘要