Explainable district heat load forecasting with active deep learning
APPLIED ENERGY(2023)
摘要
District heat load forecasting is a challenging task that involves predicting future heat demand based on historical data and various influencing factors. Accurate forecasting is essential for optimizing energy production and distribution in district heating systems. However, most existing forecasting models lack transparency and interpretability and fail to capture the spatial-temporal dependencies in the data. Moreover, they often require a large amount of annotated data for training, which can be costly and time-consuming to obtain. In this paper, we present a novel approach to district heat load forecasting, which involves predicting future heat demand based on historical data and various influencing factors. The proposed approach is based on an Active Graph Recurrent Network (Ac-GRN), which leverages the strengths of active deep learning and graph neural networks to capture the complex spatial-temporal dependencies in the data. The approach also provides explainability for its predictions by using correlation-based attribution methods. The active deep learning component can effectively select the most informative and representative samples from a large pool of data, reducing the frequency and cost of data collection and human effort. The graph neural network component can model both linear and nonlinear relationships among heat meters using bidirectional recurrent connections, enhancing the accuracy and robustness of the predictions. We conduct extensive experiments and compare our approach with eleven state-of-the-art models on a real-world dataset of district heating consumption data from Danish residential buildings. Our results show that our approach outperforms other models in terms of accuracy, robustness, reliability, and computational efficiency for multi-horizon multi-step district heat load forecasting. Our approach also provides meaningful explanations for its predictions by highlighting the most influential factors and heat meters for each prediction. This paper makes a novel contribution to district heat load forecasting with explainability.
更多查看译文
关键词
District heating,Active learning,Explainability,Prediction,Graph neural network
AI 理解论文
溯源树
样例
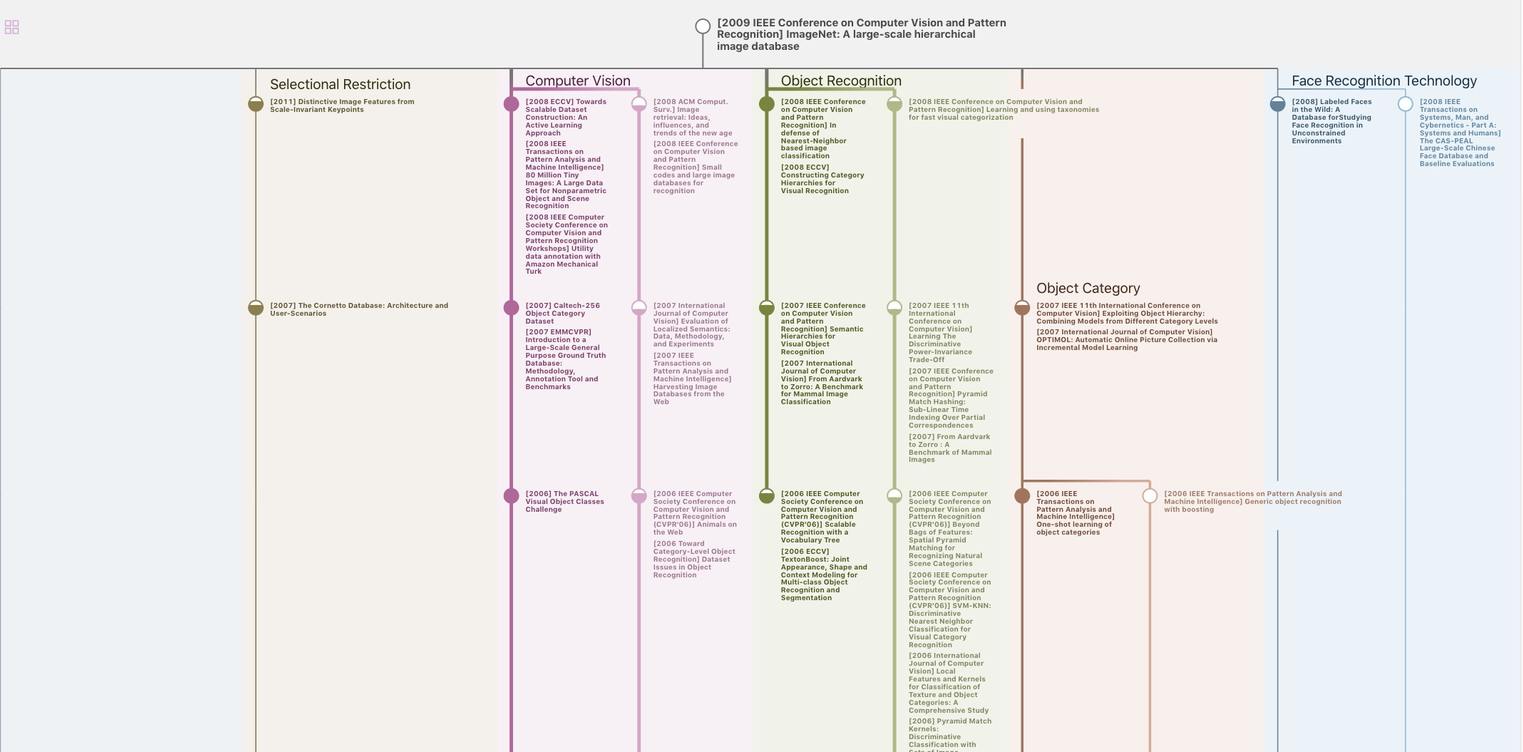
生成溯源树,研究论文发展脉络
Chat Paper
正在生成论文摘要