Multiple population-based multi-objective evolutionary semi-supervised multi-kernel region fuzzy clustering image segmentation
MEMETIC COMPUTING(2023)
摘要
Multi-objective optimization problems require the simultaneous optimization of more than one objective function. Image segmentation algorithm partitions an image into different parts according to its features and properties. It is essentially a multi-objective optimization problem because of multiple requirements. Multi-objective evolutionary fuzzy clustering (MOEFC) algorithms behave much better than traditional fuzzy clustering algorithms due to considering several cluster indices and automatically determining the number of clusters. When applied to image segmentation, MOEFC algorithms generally consume a long running time and cannot well preserve the region-level information in images. To solve these problems and meanwhile utilize some prior information derived from images to further improve the performance of MOEFC algorithms, this paper proposes a multiple population-based multi-objective evolutionary semi-supervised multi-kernel region fuzzy clustering image segmentation. First, effective region features considering color, texture and spatial information are extracted. Next, region-level-based semi-supervised fitness functions are designed using the obtained region features and some propagation supervision information. Further, in order to effectively aggregate image features, the constructed fitness functions are extended to a non-linear version utilizing a multi-kernel function. Then, a multi-population evolutionary strategy is adopted to improve the diversity of population. Except for the traditional crossover and mutation operators, the recombination operator between two populations and a center updating based elitist strategy are designed. Finally, a multiple kernel evaluation index with region information and propagation supervision information is constructed to select an optimal solution from the non-dominant solution set. Experimental results on several image datasets show that the proposed algorithm outperforms state-of-the-art methods in segmentation performance and running efficiency.
更多查看译文
关键词
Color image segmentation,Multi-objective evolutionary optimization,Semi-supervised clustering,Multiple kernel methods,Region information
AI 理解论文
溯源树
样例
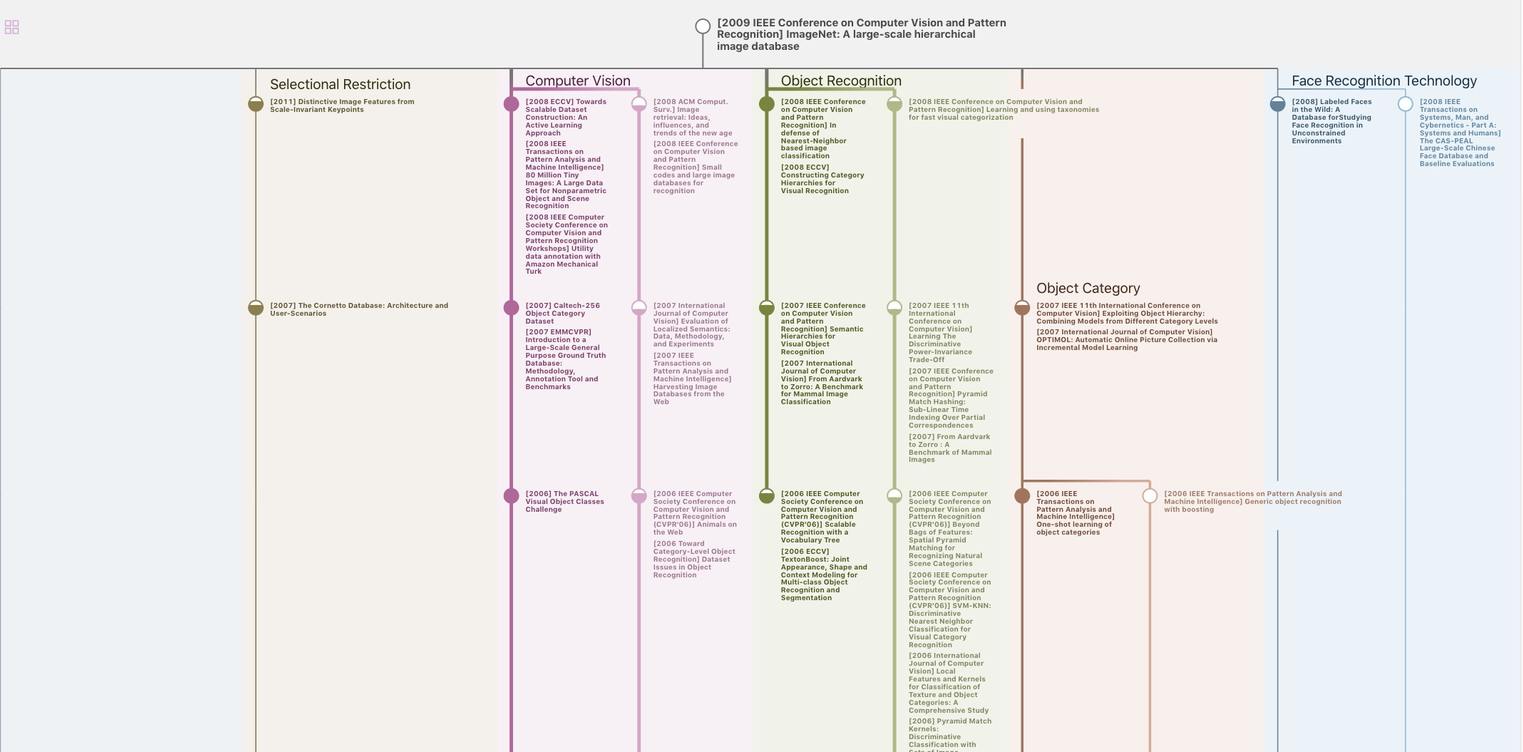
生成溯源树,研究论文发展脉络
Chat Paper
正在生成论文摘要