Transformer-Based Fused Attention Combined with CNNs for Image Classification
NEURAL PROCESSING LETTERS(2023)
摘要
The receptive field of convolutional neural networks (CNNs) is focused on the local context, while the transformer receptive field is concerned with the global context. Transformers are the new backbone of computer vision due to their powerful ability to extract global features, which is supported by pre-training on extensive amounts of data. However, it is challenging to collect a large number of high-quality labeled images for the pre-training phase. Therefore, this paper proposes a classification network (CofaNet) that combines CNNs and transformer-based fused attention to address the limitations of transformers without pre-training, such as low accuracy. CofaNet introduces patch sequence dimension attention to capture the relationship among subsequences and incorporates it into self-attention to construct a new attention feature extraction layer. Then, a residual convolution block is used instead of multi-layer perception after the fusion attention layer to compensate for the limited feature extraction of the attention layer on small datasets. The experimental results on three benchmark datasets demonstrate that CofaNet achieves excellent classification accuracy when compared to some transformer-based networks without pre-traning.
更多查看译文
关键词
Image classification, Swin transformer, Fusion attention, Residual convolution
AI 理解论文
溯源树
样例
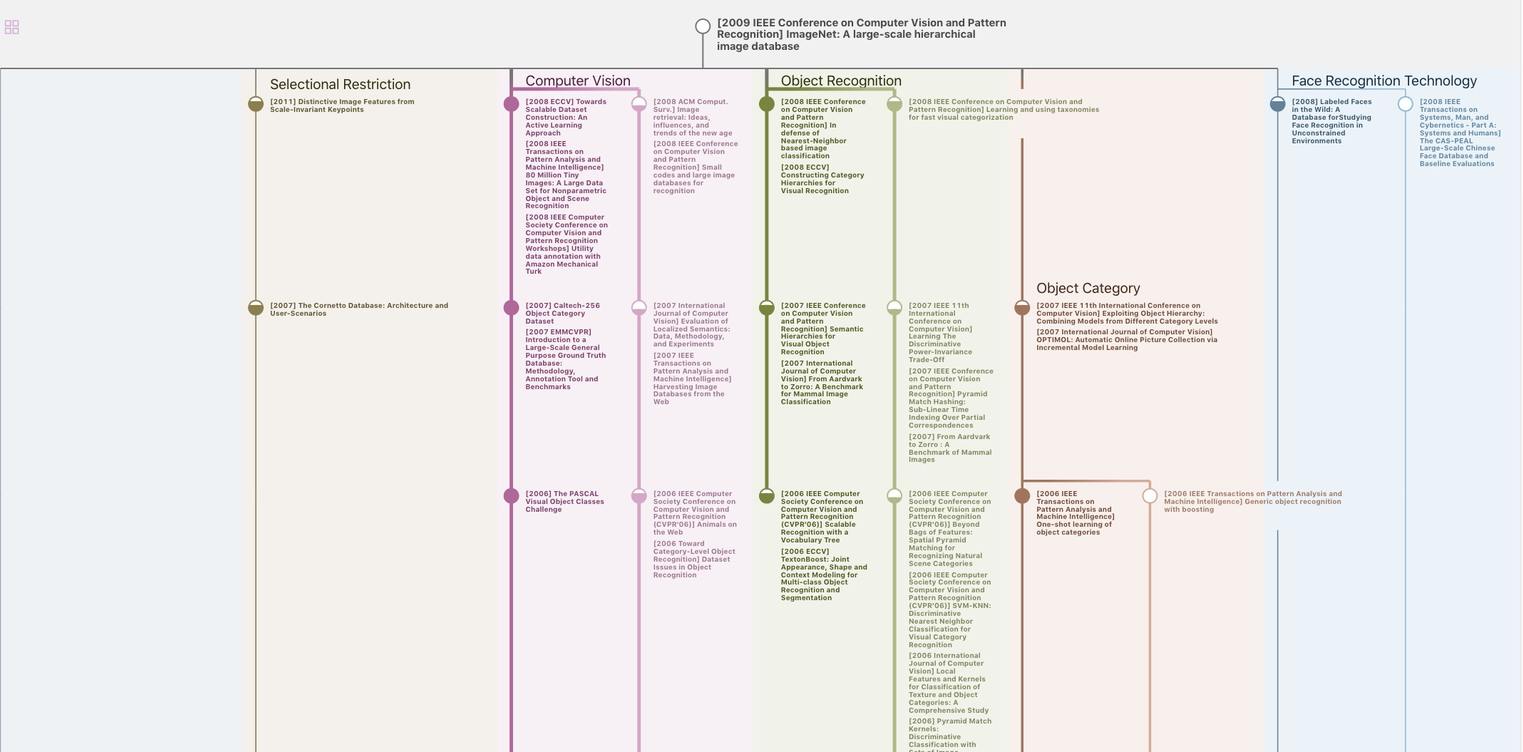
生成溯源树,研究论文发展脉络
Chat Paper
正在生成论文摘要