A crowd-AI dynamic neural network hyperparameter optimization approach for image-driven social sensing applications
KNOWLEDGE-BASED SYSTEMS(2023)
摘要
Image-driven social sensing (ISS) is emerging as a pervasive sensing paradigm that collects the status of the physical world by leveraging image data from human sensors. This paper studies a dynamic hyperparameter optimization problem in ISS applications and our objective is to dynamically determine the optimal hyperparameter configuration of an AI-based ISS solution that provides the accurate label for each image in the data stream of ISS applications. Our work is motivated by the observation that current ISS solutions leverage a static hyperparameter configuration that is often configured manually by AI experts, which fails to capture the potential large dynamics of ISS applications. To address this limitation, we develop DC-HPO, a dynamic crowd-assisted hyperparameter optimization system that utilizes crowdsourced human intelligence to dynamically steer the search of the optimal hyperparameter configuration for each image in the data stream of ISS applications. The experimental results on two ISS applications from real world (i.e., intelligent urban infrastructure monitoring and city environment cleanliness assessment) demonstrate that DC-HPO outperforms existing deep convolutional neural networks, crowd-AI models, and hyperparameter optimization methods in achieving the best ISS performance while keeping the lowest computational cost.& COPY; 2023 Elsevier B.V. All rights reserved.
更多查看译文
关键词
Crowdsourcing,Dynamic hyperparameter optimization,Crowd-AI collaboration,Social sensing
AI 理解论文
溯源树
样例
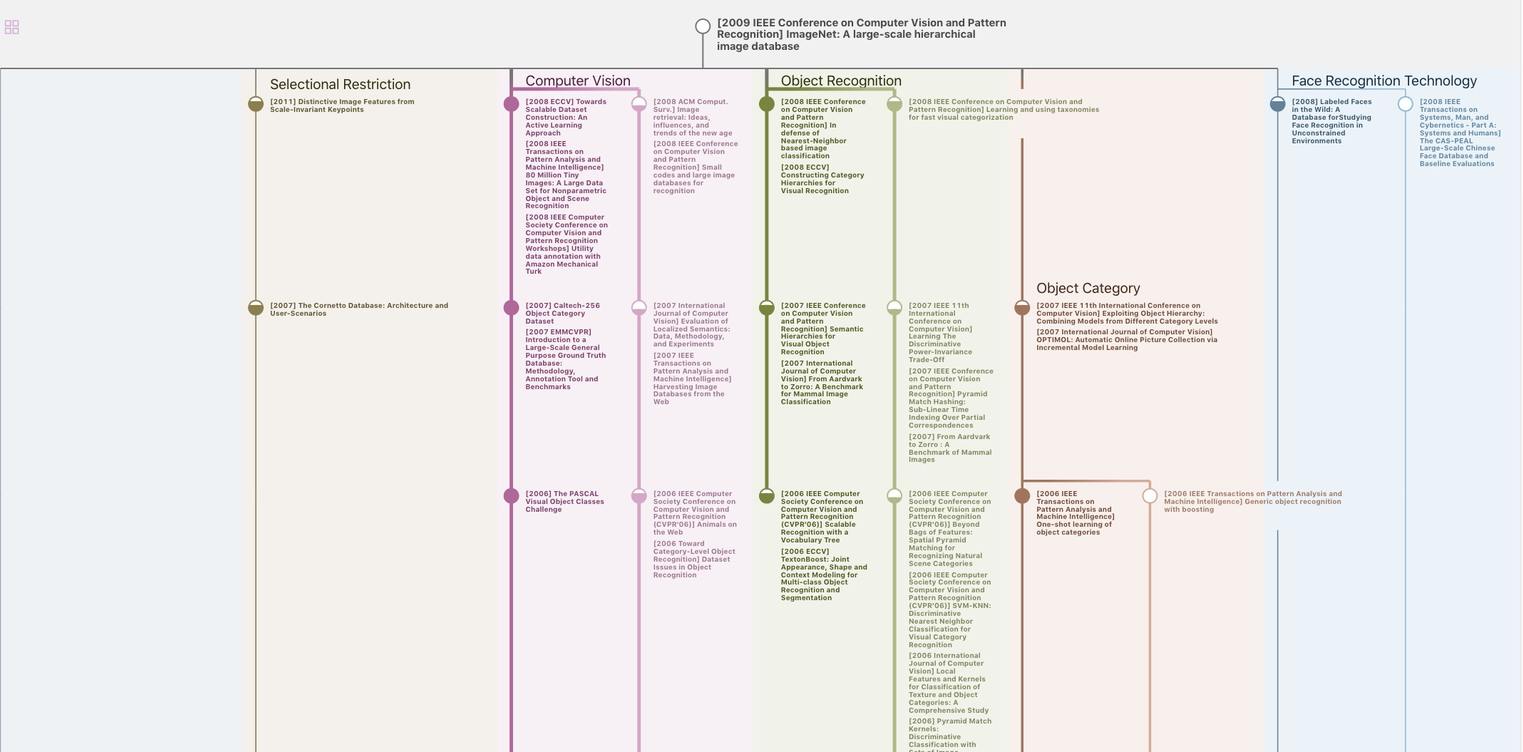
生成溯源树,研究论文发展脉络
Chat Paper
正在生成论文摘要