Improving High-Resolution Ensemble Forecast (HREF) System Mesoscale Snowband Forecasts with Random Forests
WEATHER AND FORECASTING(2023)
摘要
Mesoscale snowbands are impactful winter weather phenomena but are challenging to predict due to small-scale forcings and ingredients. Previous work has established that even deterministic convection-allowing models (CAMs) often struggle to represent these features with much precision and recommended the application of ingredients-based or probabilistic forecast strategies. Based on these recommendations, we develop and evaluate four different models for fore-casting snowbands. The first model, referred to as the "HREF threshold probability" model, detects band development in High-Resolution Ensemble Forecast (HREF) system members' 1000-m simulated reflectivities, then uses these detections to calculate a snowband probability. The second model is a random forest incorporating features explicitly linked to snow -bands, such as the detection of bands in each HREF member and statistical summaries of simulated reflectivity and the categorical snow field. The third model is a random forest model incorporating snowband ingredients, such as midtropo-spheric frontogenesis, moist symmetric stability, and vertical velocity. Last, the fourth model combines the features of the explicit and implicit random forests. Binary band predictions based upon the HREF threshold probability model resulted in a critical success index 27% higher than the average HREF member. The explicit feature random forest model further improved performance by an additional 11%, with statistics of the reflectivity field holding the most predictive value. The implicit and combined random forests slightly underperformed the explicit random forest, perhaps due to a large number of noisy, correlated features. Ultimately, we demonstrate that simple probabilistic snowband forecasting strategies can yield substantial improvements over deterministic CAMs.SIGNIFICANCE STATEMENT: Mesoscale snowbands have the potential for major societal impacts but are difficult to predict due to their small spatial scales. Previous work has shown that individual high-resolution numerical weather prediction (NWP) models struggle to predict whether or not a snowband will occur. In this work, we evaluate whether a probabilistic forecast strategy using high-resolution ensemble NWP output leads to improved snowband forecasts, and whether we can gain additional predictive skill by combining this output with artificial intelligence (AI) methods. AI can also help us understand the environmental factors associated with snowbands and compare environmental im-portance in forecasting to just using the model output snowfall forecasts explicitly.
更多查看译文
关键词
Snowbands, Forecast verification/skill, Probability forecasts/models/distribution, Artificial intelligence, Classification, Decision trees
AI 理解论文
溯源树
样例
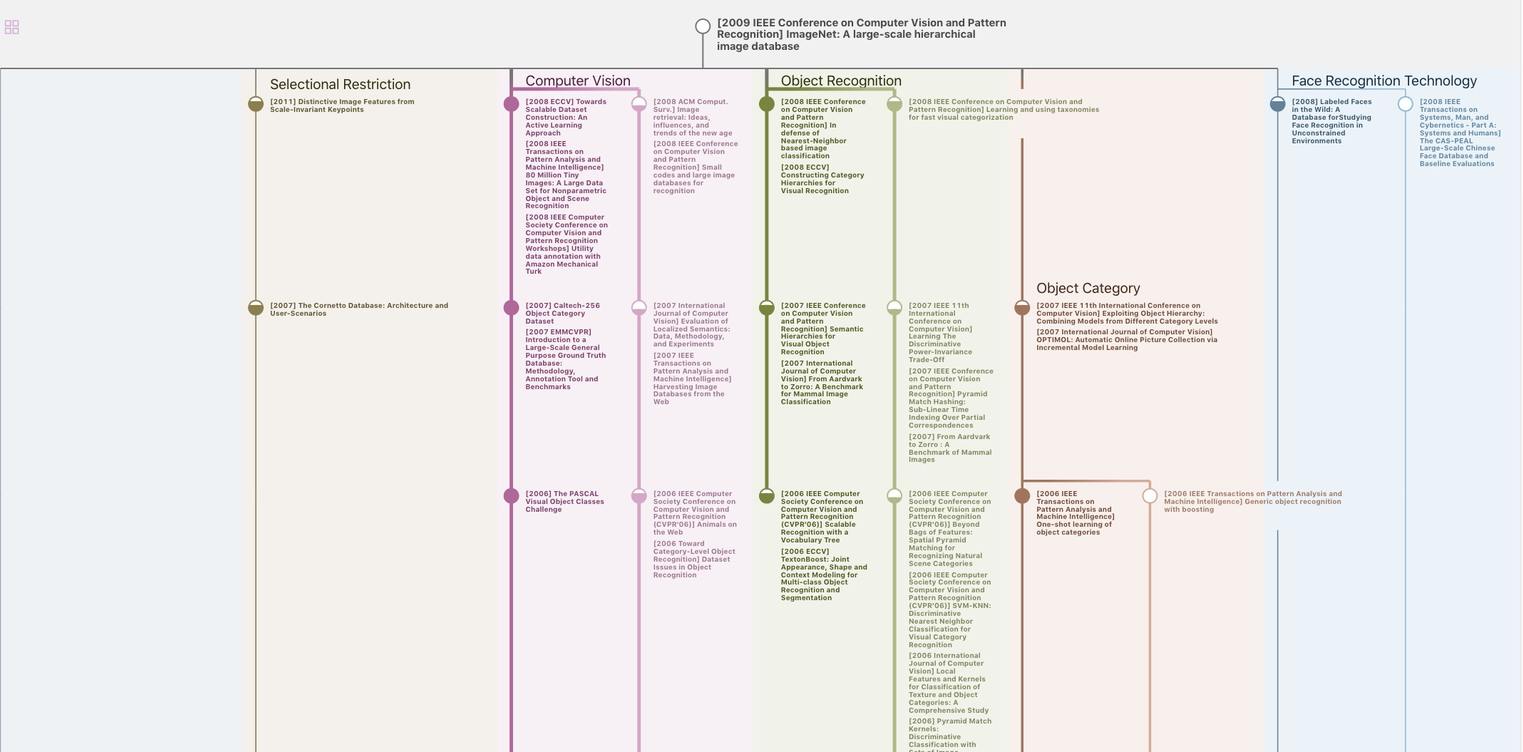
生成溯源树,研究论文发展脉络
Chat Paper
正在生成论文摘要