Quaternion Deformable Local Binary Pattern and Pose-Correction Facial Decomposition for Color Facial Expression Recognition in the Wild
IEEE TRANSACTIONS ON COMPUTATIONAL SOCIAL SYSTEMS(2023)
Abstract
Facial expression recognition (FER) in the wild is a more challenging topic than that under laboratory-controlled conditions. The major obstacles of FER in the wild are head pose variations, illumination changes, and different skin colors. To address these problems, we propose a framework named quaternion deformable local binary pattern (QDLBP)-Net for color FER in the wild. First, to eliminate the interferences of head pose variations, a pose-correction facial decomposition (PCFD) strategy is proposed to correct the head pose and decompose the facial image into five emotion-related regions. Then, to handle the problems of illumination changes and different skin colors, an effective feature descriptor named "QDLBP" is developed. QDLBP extracts color quaternion features from each emotional region, which not only computes the strength of emotional features, but also maintains the spectral correlation between color channels. Finally, a quaternion classification network (QC-Net) is proposed to classify the quaternion features from five emotional regions into seven basic expressions. The experimental results on three in-the-wild FER datasets and two nonfrontal pose variation datasets exhibit the effectiveness and superiority of QDLBP-Net by showing clear performance improvements over other state-of-the-art (SOTA) FER methods.
MoreTranslated text
Key words
Color facial expression recognition (FER),local binary pattern (LBP),quaternion
AI Read Science
Must-Reading Tree
Example
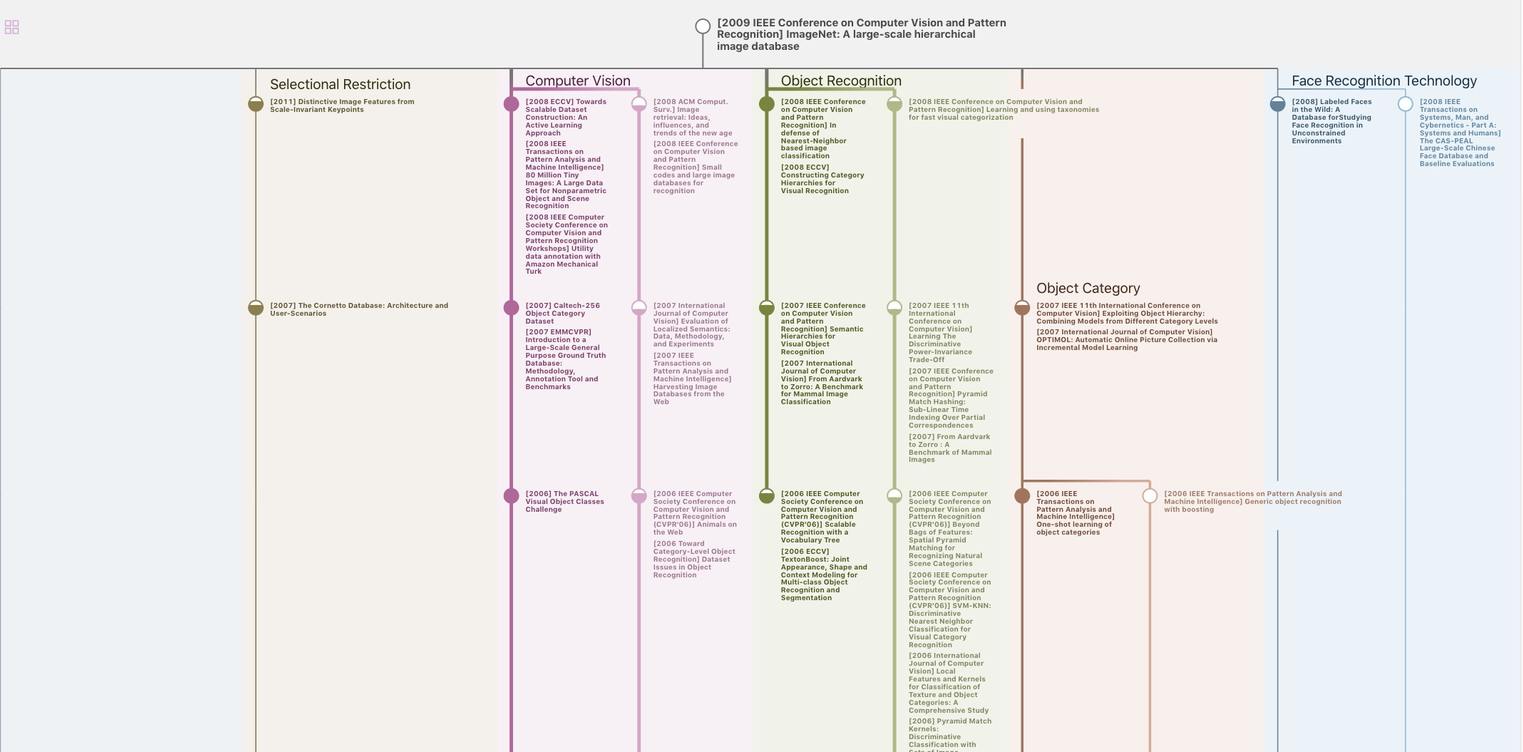
Generate MRT to find the research sequence of this paper
Chat Paper
Summary is being generated by the instructions you defined