Integrating Model Predictive Control With Federated Reinforcement Learning for Decentralized Energy Management of Fuel Cell Vehicles
IEEE TRANSACTIONS ON INTELLIGENT TRANSPORTATION SYSTEMS(2023)
摘要
The optimization-based energy management strategy (EMS) enables expertise to improve the performance of fuel cell vehicles (FCVs). Ongoing efforts are mostly focused on optimizing a centralized EMS using a variety of high-computing technologies without offering appropriate scalability and modularity for the onboard powertrain components. In real-time applications, the time-accomplishment capability of EMSs is crucial; hence, decentralized EMSs with low-cost components and limited processing capability are necessary. Local units handle the computation load on a modular platform. In addition, the decentralized system's plug-and-play functionality minimizes the total cost. This paper presents a decentralized model predictive control (D-MPC) based on the consensus-based alternating direction method of multipliers (C-ADMM) that explicitly considers the coordination of the dynamic reactions of powertrain components and future driving profiles. In addition, a decentralized learning method is proposed to seek the optimal policy for the moving horizon dimensions in the D-MPC using the federated reinforcement learning (FRL) algorithm in order to improve processing time. Due to the deployment of a fully modular system in the proposed learning technique, agents are restricted from sharing their trajectories. Using a highly dynamic module-to-module communication layer in a fully decentralized arrangement, the powertrain components utilize the multi-step method to attain the global optimum. The performance of the proposed framework is evaluated with regards to its precision, convergence speed, and scalability. The results of numerical simulation and implementation demonstrated that the proposed method is superior to the centralized and fixed-horizon MPC approaches.
更多查看译文
关键词
Alternating direction method of multipli-ers (ADMM), federated reinforcement learning (FRL), proton exchange membrane fuel cell (PEMFC), distributed optimization algorithms, energy management strategy (EMS), fuel cell vehicle (FCV), model predictive control (MPC)
AI 理解论文
溯源树
样例
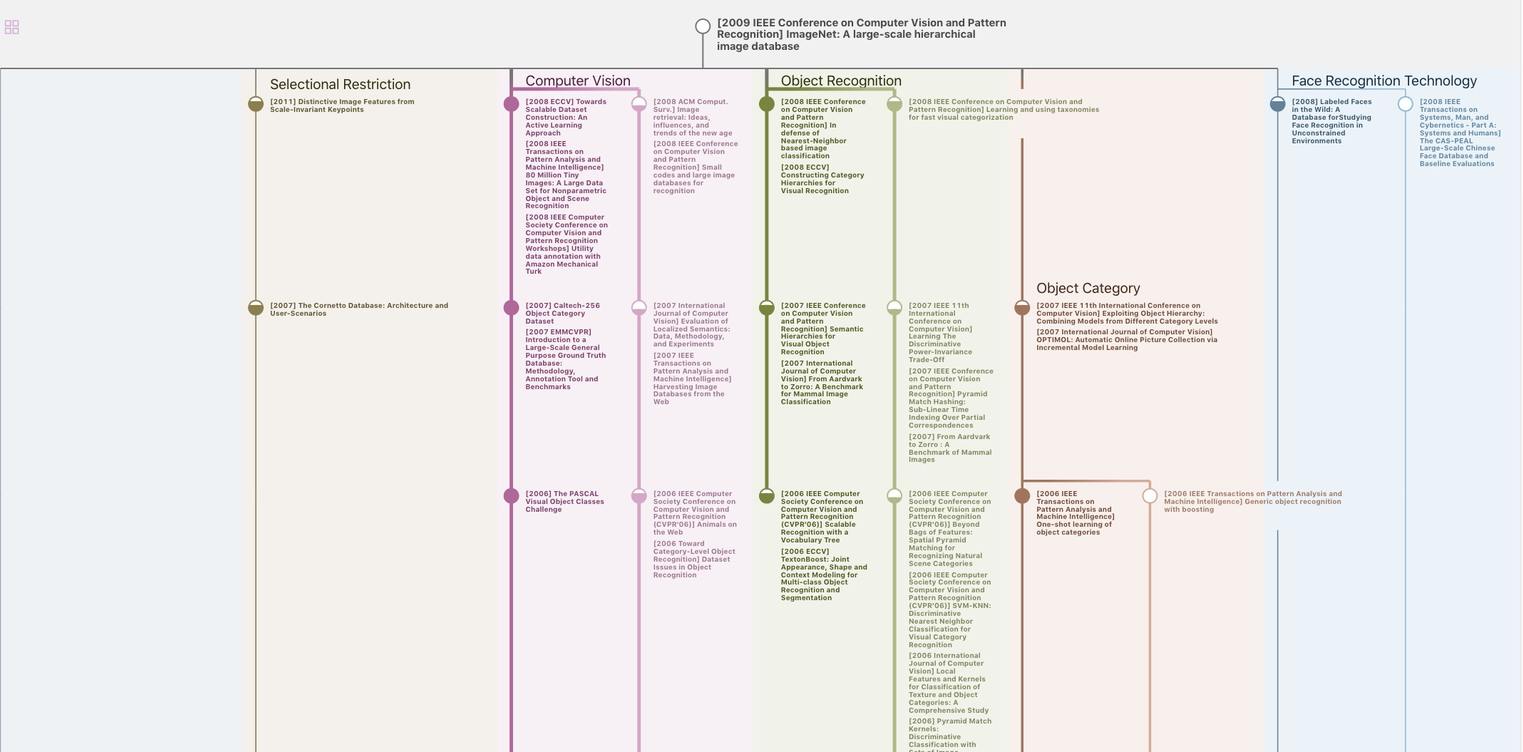
生成溯源树,研究论文发展脉络
Chat Paper
正在生成论文摘要