A hierarchical outlier detection method for spare parts transaction
INTERNATIONAL JOURNAL OF SPACE-BASED AND SITUATED COMPUTING(2023)
摘要
In nuclear power production, seamless equipment maintenance is integral, significantly achieved through spare parts transactions. Yet, abnormal transaction data can obstruct operational efficiency. Traditional anomaly detection methods, often subjective and non-scalable, struggle to handle these abnormalities effectively. Addressing these shortcomings, this paper presents a Hierarchical Outlier Detection Method, specifically for nuclear power spare parts transaction data. This method integrates data cleansing, probability statistics, and model recognition modules, offering an innovative, robust approach to anomaly detection. Leveraging statistical and neural network approaches, our method exhibits superior adaptability, computational efficiency, and detection performance, substantiated through a real-world dataset of nuclear power plant spare parts transaction records. Its versatile design suggests potential applicability to other industrial scenarios.
更多查看译文
关键词
anomaly detection,spare parts transactions,data cleansing,probability statistics,model recognition
AI 理解论文
溯源树
样例
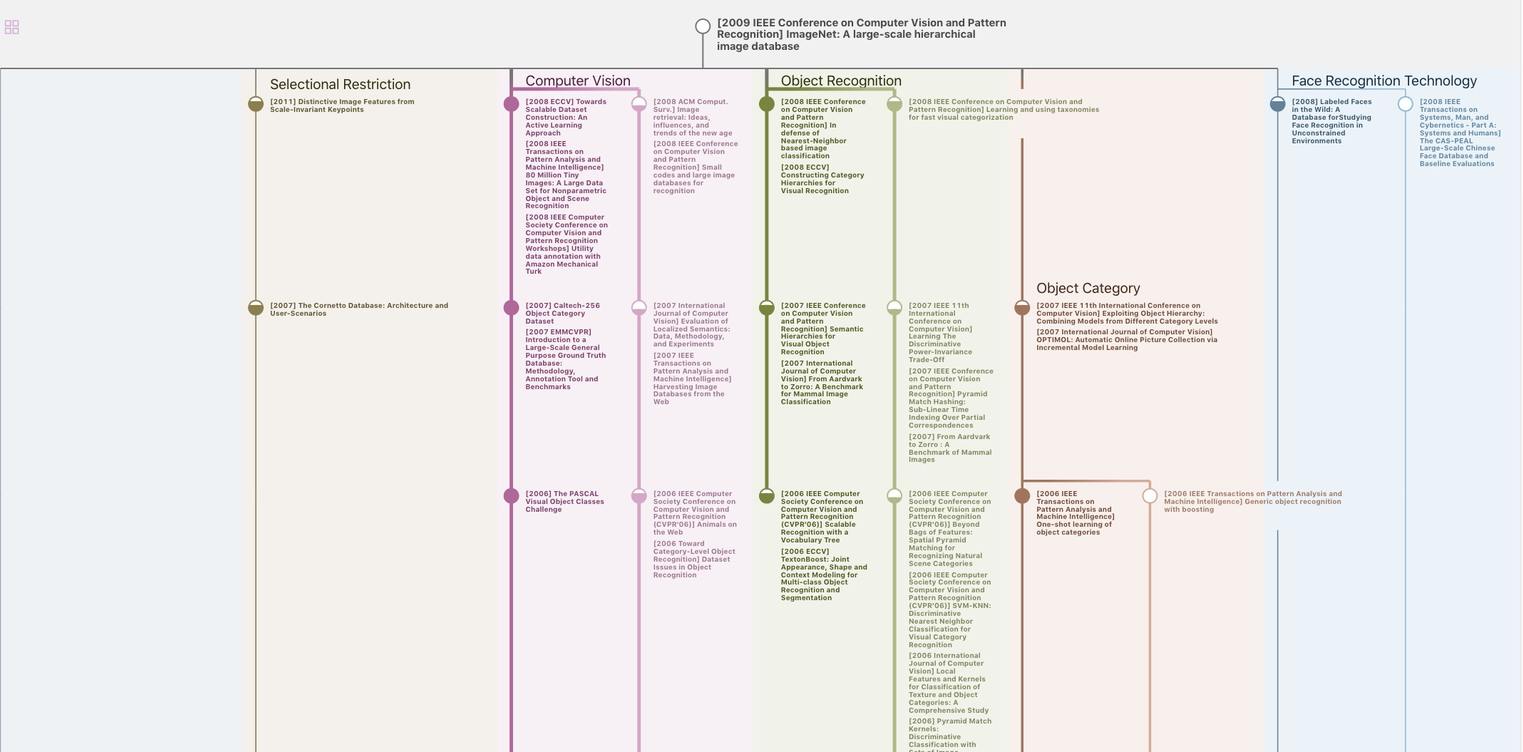
生成溯源树,研究论文发展脉络
Chat Paper
正在生成论文摘要