Lite-FENet: Lightweight multi-scale feature enrichment network for few-shot segmentation
KNOWLEDGE-BASED SYSTEMS(2023)
摘要
Current methods for few-shot segmentation focus on extracting information from support and query targets, however, most of these methods not only suffer from high model complexity but also fail to capture long-range interactions between query features and support features. To efficiently reduce the information loss due to the appearance inconsistency in spatial between support and query objects and make full use of the limited features extracted from support and query samples, we present a lightweight multi-scale feature enrichment network named Lite-FENet that enhances multi-scale feature interactions and ensures the discrimination with the final features under low computational cost. Specifically, we design a lightweight and efficient spatial interaction module for the Lite-FENet to achieve the contextual information transfer between different spatial scales over long distances. The repeated interactions generate a descriptive feature, and show superior robustness for pixel-level prediction, especially when there is a gap between different instances. Experimental results show that the proposed method achieves 63.5% and 64.7% MIoU on the PASCAL-5i dataset, and 39.5% and 43.1% MIoU on the COCO-20i datasets under 1-shot and 5-shot settings with only 6.7M learnable parameters, respectively, which significantly exceeds the results of current methods, while ensuring a far smaller model size. Code is available at: https://github.com/Sunbaoquan/Lite-FENet.& COPY; 2023 Elsevier B.V. All rights reserved.
更多查看译文
关键词
Image segmentation,Few-shot segmentation,Few-shot learning,Lightweight network,Multi-scale feature
AI 理解论文
溯源树
样例
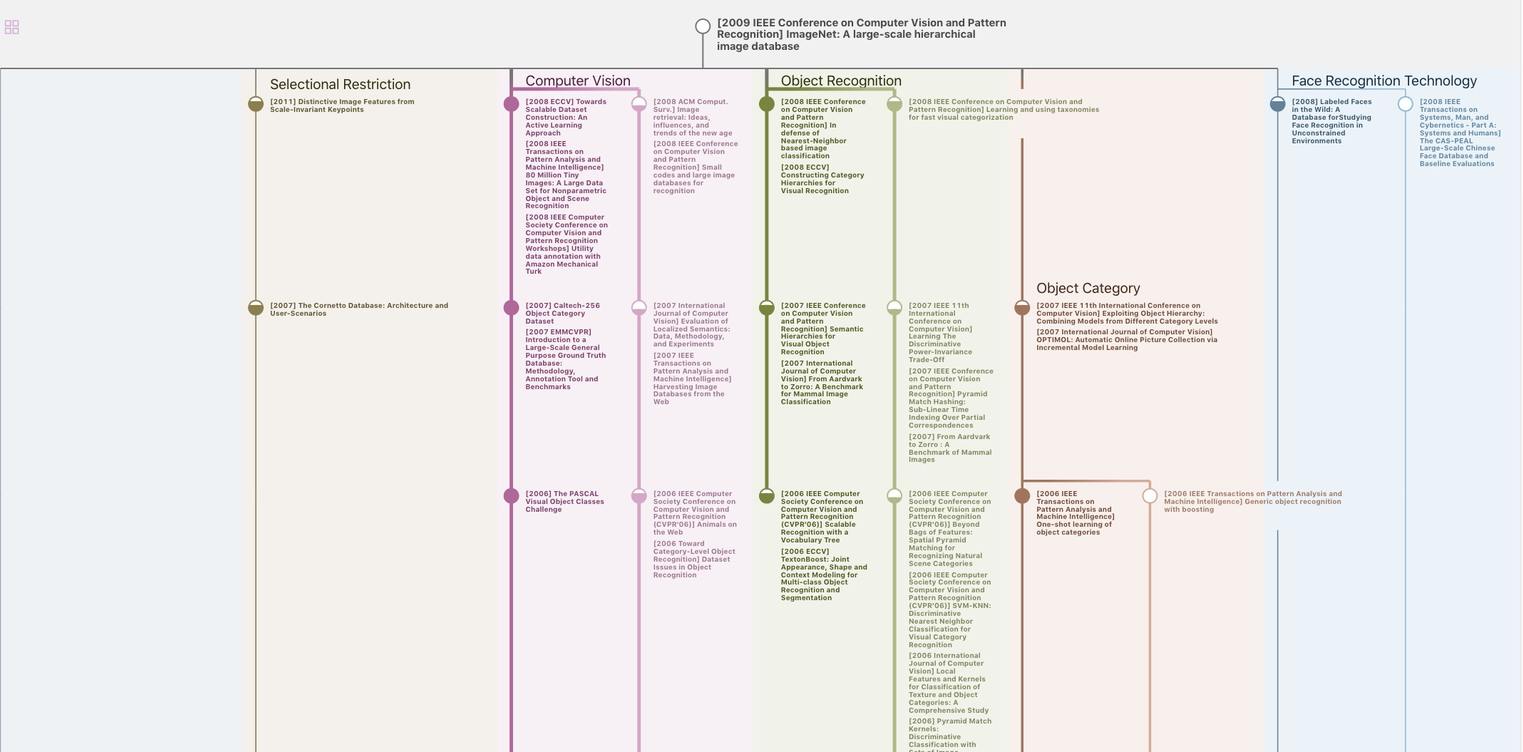
生成溯源树,研究论文发展脉络
Chat Paper
正在生成论文摘要