Static and Dynamic Collision Avoidance for Autonomous Robot Navigation in Diverse Scenarios based on Deep Reinforcement Learning
2023 20TH INTERNATIONAL CONFERENCE ON UBIQUITOUS ROBOTS, UR(2023)
摘要
This paper proposes an efficient robot training method to navigate environments with static and dynamic obstacles and reach their goal autonomously using deep reinforcement learning algorithms. Previous methods have focused on specific scenarios, such as crowded environments. However, in practice, the variety of scenarios with static and dynamic obstacles tends to make the real robotic system fail or be restricted. In this work, the training is performed in six scenarios per episode, whereas traditional methods only consider one scenario. Several neural networks are trained and compared based on the following metrics: success rate, collision rate, uncomfortable rate, travel time, and average travel distance. In addition, we conducted Gazebo simulations using ROS and experimental tests across four scenarios to demonstrate that our approach has a better performance compared to previous studies. The results show a greatly enhanced robot's ability to act in various situations, as shown in the following link: https://youtu.be/mvkMFZjlaQo
更多查看译文
关键词
autonomous robot navigation,collision avoidance,collision rate,deep reinforcement learning,dynamic obstacles,Gazebo simulations,neural networks,robot training method,robotic system,ROS,static obstacles
AI 理解论文
溯源树
样例
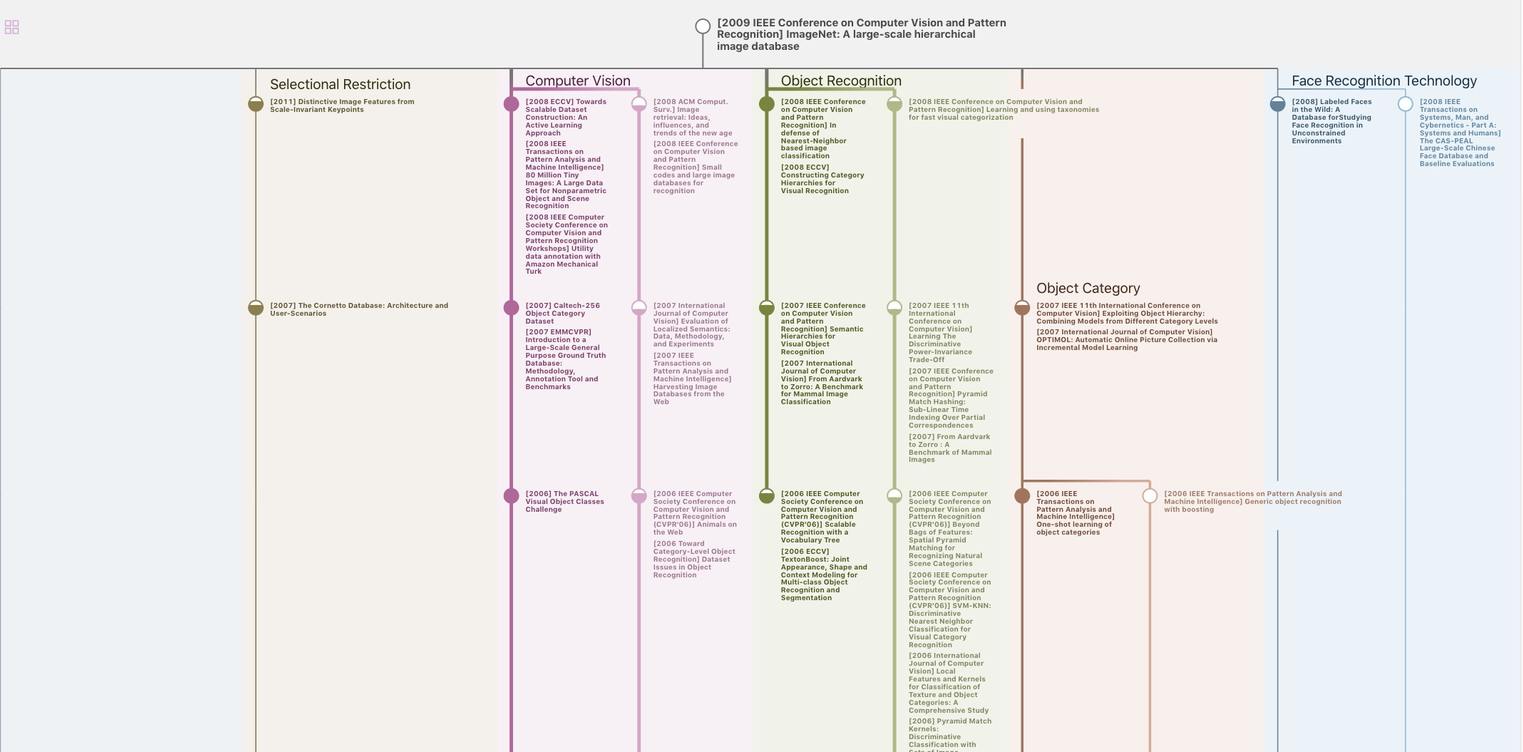
生成溯源树,研究论文发展脉络
Chat Paper
正在生成论文摘要