Leakage Detection in Water Distribution Network Using Machine Learning
PIPELINES 2023: CONDITION ASSESSMENT, UTILITY ENGINEERING, SURVEYING, AND MULTIDISCIPLINE(2023)
摘要
Recent advancements and interest in leakage detection of the water pipeline systems are due to the aging drinking water infrastructure and scarcity of fresh drinking water across the globe. Approximately 20% of treated drinking water is lost due to leakages caused by deteriorating water distribution networks. The current leakage detection techniques only provide information about the leaks during testing and do not support real-time monitoring. This study is focused on developing machine learning models based on supervised classification algorithms for fast and reliable leakage detection through flow-induced surface vibration data that can be used for near real-time monitoring. A two-looped real-life polyvinyl chloride (PVC) pipeline network comprising an 820-L reservoir, a pump, various bends, diameters, leakage simulators, soil backfills, and a flowmeter is used to collect flow-induced surface vibration data using six accelerometers. Six accelerometers are mounted on the unburied section of the test bed to collect vibration signals for various non-leaky and leaky scenarios for training and validating the machine learning models. The models are trained using 80% of the vibration signals and validated using the remaining 20%. The performance of machine learning models is assessed using accuracy, recall, precision, F-score, and area under the curve. A summary of hyperparameter tuning and training time is also included in this study to discuss the trade-off between the required computing resources and the accuracies of the machine learning model. The result presented in this study provides a methodology and machine learning models that can be used further to develop real-time monitoring approaches for drinking water pipeline systems.
更多查看译文
AI 理解论文
溯源树
样例
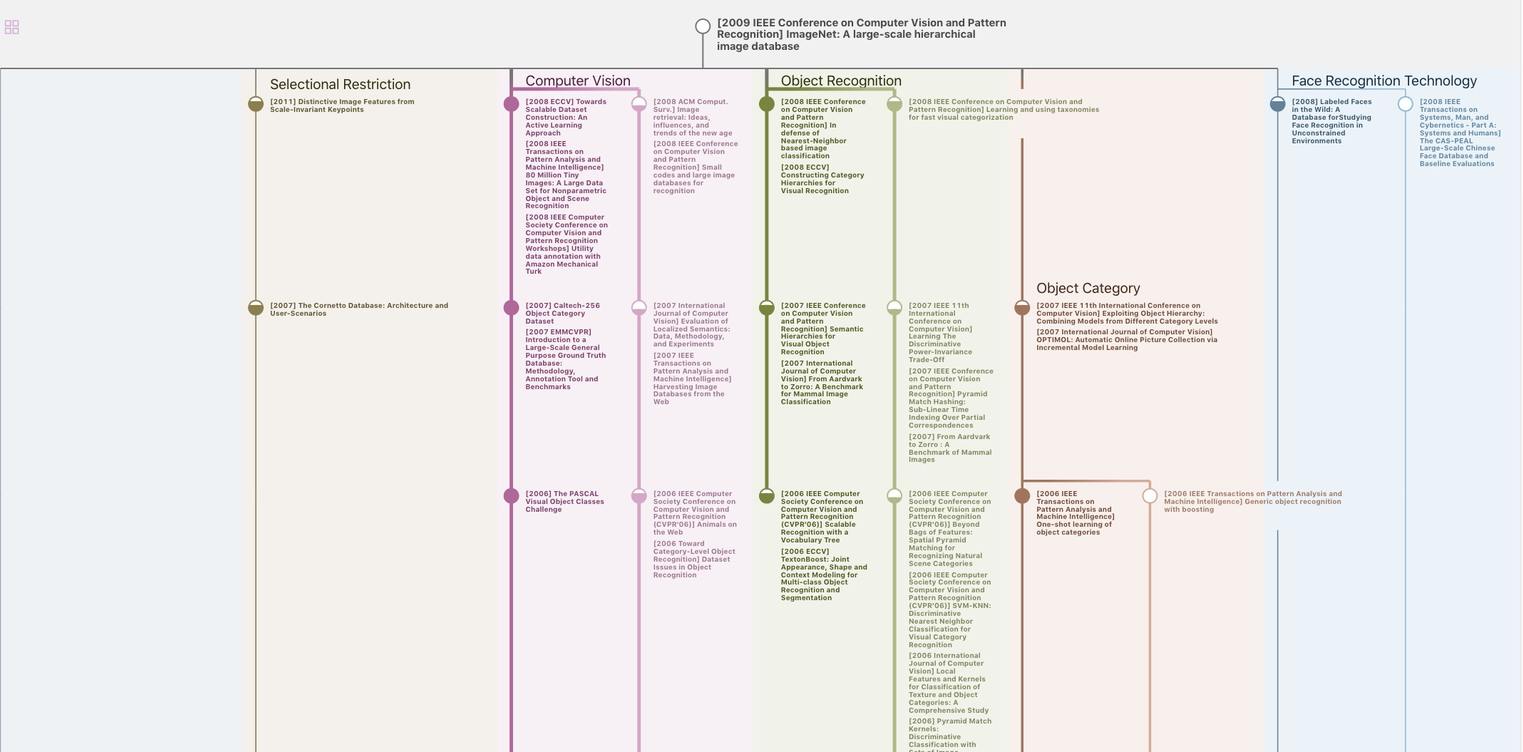
生成溯源树,研究论文发展脉络
Chat Paper
正在生成论文摘要