SMR-RS: An Improved Mask R-CNN Specialized for Rolled Rice Stubble Row Segmentation
APPLIED SCIENCES-BASEL(2023)
Abstract
As a highly productive rice, ratoon rice is widely planted worldwide, but the rolling of rice stubble in mechanical harvesting severely limits its total yield; based on this, some scholars have proposed rolled rice stubble righting machines. However, limited by the uncertainty of the field environment, the machine's localization accuracy of the target needs to be improved. To address this problem, real-time detection of rolled rice stubble rows is a prerequisite. Therefore, this paper introduces a deep learning method for the first time to achieve this. To this end, we presented a novel approach to improve a model that is used for the simplification of Mask R-CNN, which does not require any modules to be added or replaced on the original model. Firstly, two branches in the second stage were deleted, and the region proposals output from the stage was used directly as the mask generation region, and segmentation performance was substantially improved after a simple optimization of the region proposals. Further, the contribution of the feature map was counted, and the backbone network was simplified accordingly. The resulting SMR-RS model was still able to perform instance segmentation and has better segmentation performance than Mask R-CNN and other state-of-the-art models while significantly reducing the average image processing time and hardware consumption.
MoreTranslated text
Key words
ratoon rice,convolutional neural network,instance segmentation,model simplification,Mask R-CNN
AI Read Science
Must-Reading Tree
Example
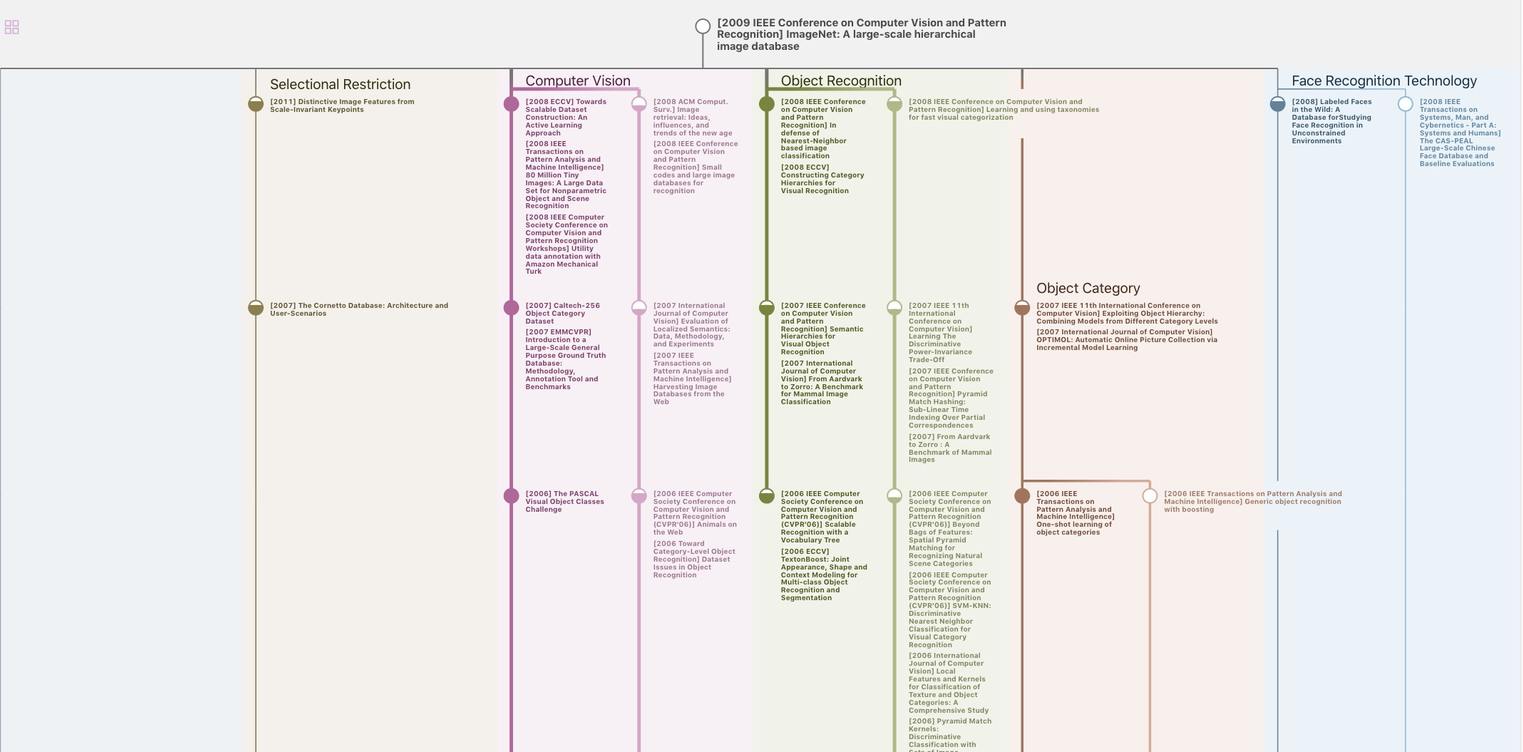
Generate MRT to find the research sequence of this paper
Chat Paper
Summary is being generated by the instructions you defined