Adama II wind farm long-term power generation forecasting based on machine learning models
SCIENTIFIC AFRICAN(2023)
摘要
The present article develops time series machine learning models to forecast the Adama II wind farm's long-term power production using SCADA data. The study applied data from the previous six years (2016 to 2021) with five years of data for training the model and the remaining one year for testing and validation. The study compares six supervised learning algorithms: Elastic net regression, Random forest regression, SARIMA, XGBoost, Prophet, and combined Prophet and XGBoost model. The projections for the 1-hour, 1-week, and 12-month forecasting ranges are compared for each forecasting model. The findings demonstrate that SARIMAX models outperform other models for forecasting one hour and one week, with a result of a 90% of R2 score and a 24% mean absolute percentage error (MAPE). However, XGBoost (with Fourier terms for seasonality) provides the foremost long-term forecasting result which is 7.33% MAPE for yearly prediction. Moreover, a combined Prophet and XGBoost for year-ahead wind power predictions has yield superior performance when compared to using each model individually which is 6.9% MAPE. Hence, for wind farms such as Adama II's long-term power forecasting, a combined prophet and XGBoost model fits well and provides accurate power generation insight.
更多查看译文
关键词
Adama II Wind Farm,Wind farm,Long-term power forecasting,Machine learning,XGBoost,SARIMAX,Prophet,Elastic net regression,Random forest regression
AI 理解论文
溯源树
样例
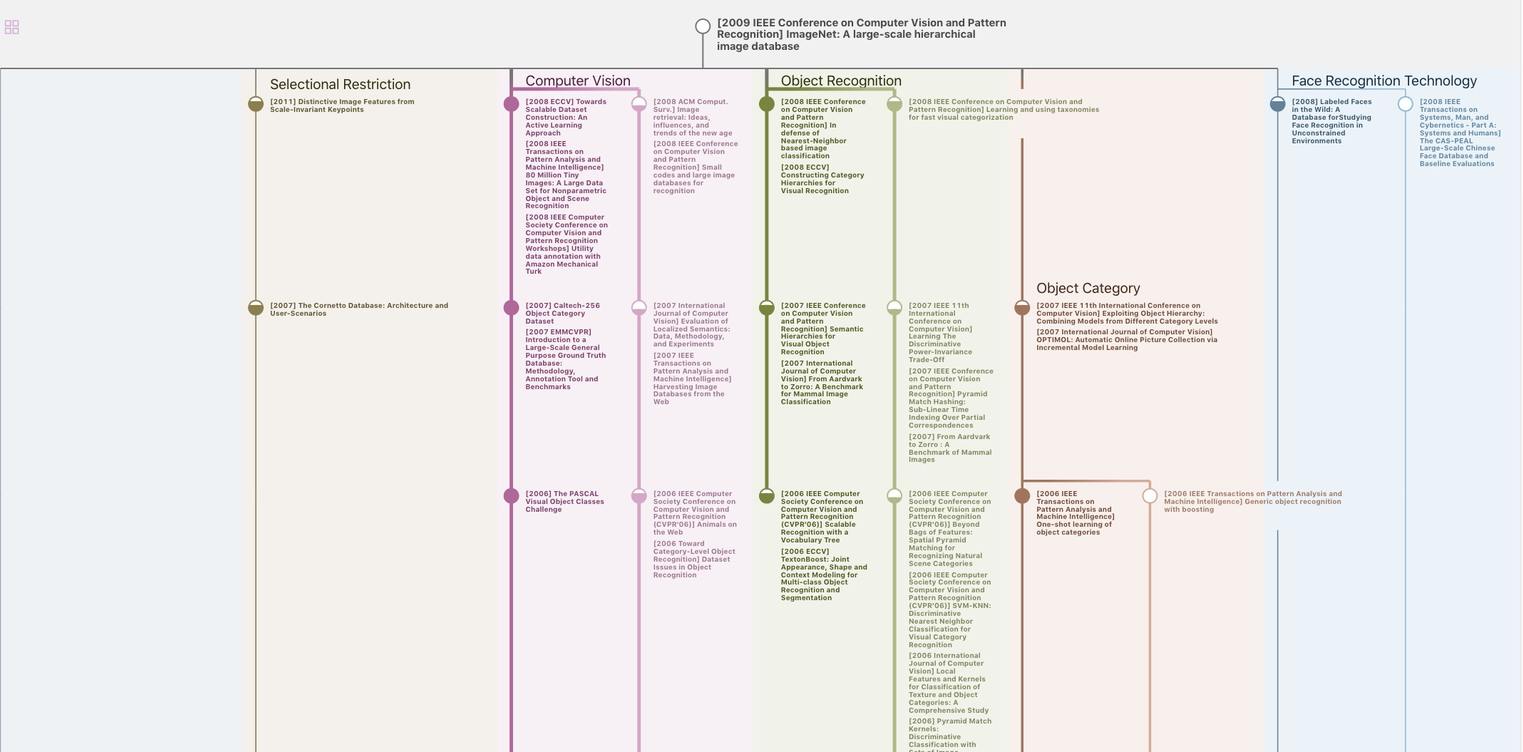
生成溯源树,研究论文发展脉络
Chat Paper
正在生成论文摘要