Machine Learning Accelerated Prediction of Self-Trapped Excitons in Double Halide Perovskites
ADVANCED ENERGY AND SUSTAINABILITY RESEARCH(2023)
摘要
Broadband emission induced by self-trapped excitons (STEs) in double halide perovskites (DHPs) has received continuous attention in recent years. However, the comprehensive understanding of the STEs formation mechanism is still in its early stage. The corresponding roles of different B-site cations also remain unclear in these advanced materials. The lack of an effective STEs database for DHPs hinders the efficient discovery of potential optoelectronic materials with strong STEs. Herein, a systematic STEs database is built for DHPs through density functional theory (DFT) calculations and proposed a highly efficient predictive machine learning (ML) model of the Huang-Rhys factor S for the first time. Results reveal the different contributions of two B-site metal cations to the formation of STEs in DHPs, which helps to understand the in-depth nature of STEs. Based on the accurate predictions of the effective phonon frequency ?(LO), it is further realized that the prediction of S without conducting the time-consuming phonon property calculations of DHPs offers new opportunities for exploring the STEs. Combining DFT calculations and ML techniques, this study supplies an effective approach to efficiently discover the potential novel optoelectronic materials, which provides important guidance for the future exploration of promising solid-state phosphors. The in-depth investigations of self-trapped excitons in double halide perovskites still have significant challenges, which are important for solid-state phosphors developments. Herein, the introduction of machine learning techniques has successfully realized the predictions of the Huang-Rhys factor for the first time and the contributions of B site metals, offering effective guidance for future research. image (C) 2023 WILEY-VCH GmbH
更多查看译文
关键词
density functional theory calculations, double halide perovskites, electron-phonon coupling, machine learning, self-trapped excitons
AI 理解论文
溯源树
样例
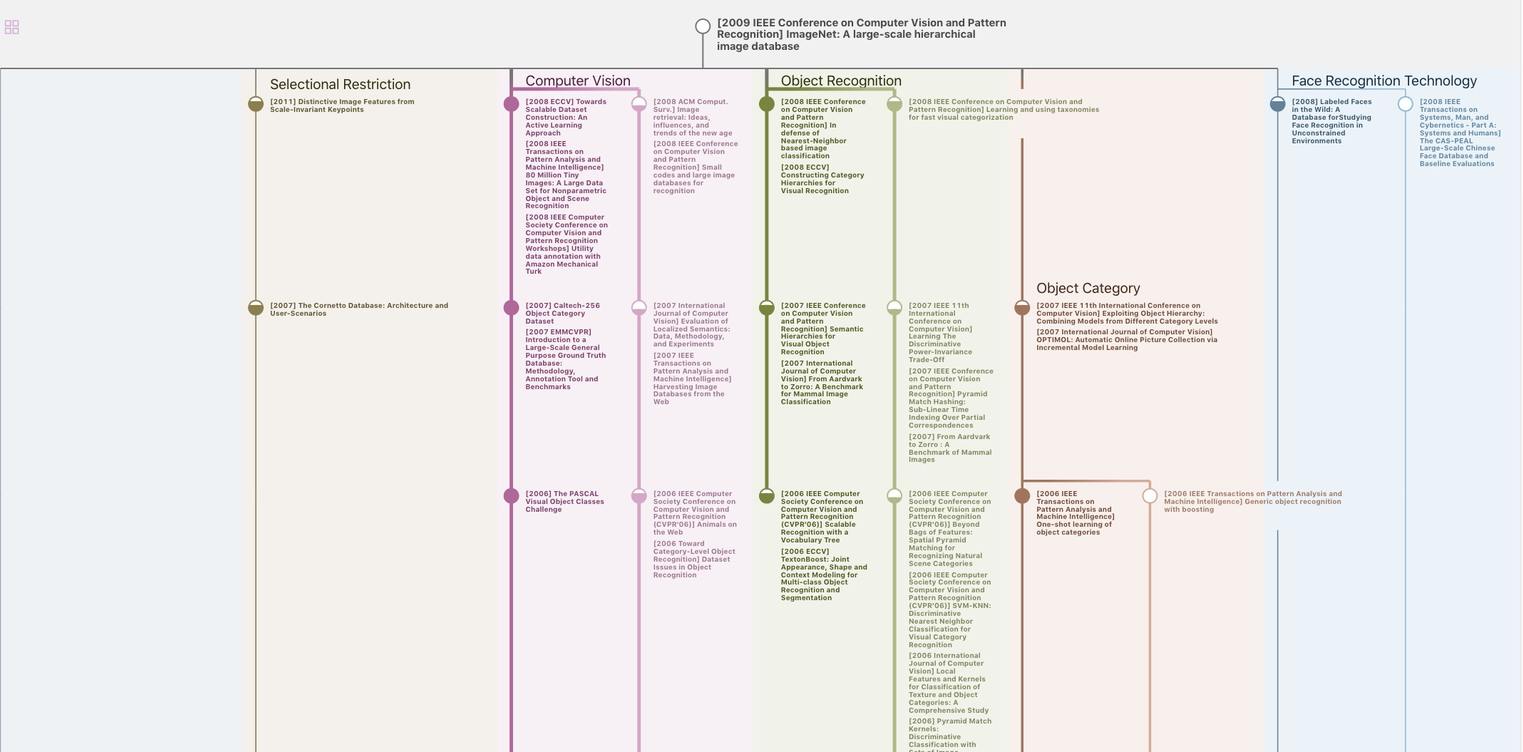
生成溯源树,研究论文发展脉络
Chat Paper
正在生成论文摘要