Feature importance measure of a multilayer perceptron based on the presingle-connection layer
KNOWLEDGE AND INFORMATION SYSTEMS(2024)
摘要
In many fields, the interpretability of machine learning models holds equal importance to their prediction accuracy. Highly accurate predictions are possible with a multilayer perceptron (MLP) neural network, but its application in high-risk fields is constrained by its lack of interpretability. To solve this issue, this paper introduces an MLP with a presingle-connection layer (SMLP). The SMLP incorporates a single-to-single connection layer with the ReLU function before the original MLP. By examining the weights of the single-connection layer after training the model, the significance of the input features can be determined. The experimental results demonstrate that this method can accurately measure the feature importance with the MLP. It offers advantages such as a straightforward theory, practical implementation, strong stability, and high reliability when compared with other widely used feature importance algorithms. Moreover, this measure effectively reveals the black box of the MLP, indicates the influence of input features on the prediction, and provides a quantitative standard for feature selection in MLP.
更多查看译文
关键词
Presingle-connection layer,Multilayer perceptron,Feature importance,Interpretability,Feature selection,Artificial neural network
AI 理解论文
溯源树
样例
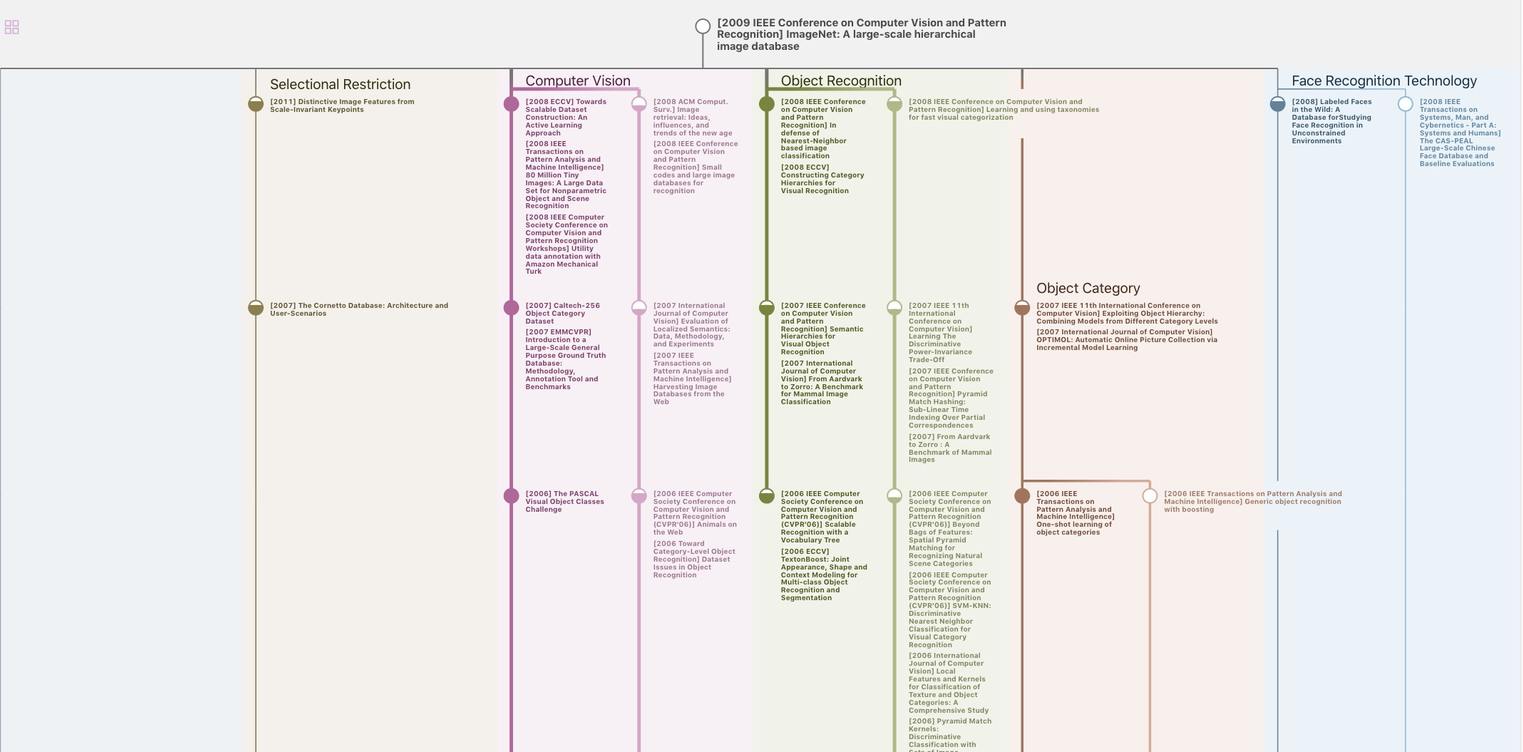
生成溯源树,研究论文发展脉络
Chat Paper
正在生成论文摘要