DeepCrackAT: An effective crack segmentation framework based on learning multi-scale crack features
ENGINEERING APPLICATIONS OF ARTIFICIAL INTELLIGENCE(2023)
摘要
The detection of cracks is essential for assessing and maintaining building and road safety. However, the large appearance variations and the complex topological structures of cracks bring challenges to automatic crack detection. To alleviate the above challenges, we propose a deep multi-scale crack feature learning model called DeepCrackAT for crack segmentation, which is based on an encoder-decoder network with feature tokenization mechanism and attention mechanism. Specifically, we use hybrid dilated convolutions in the first three layers of the encoder-decoder to increase the network's receptive field and capture more crack information. Then, we introduce a tokenized multilayer perceptron (Tok-MLP) in the last two layers of the encoder-decoder to tokenize and project high-dimensional crack features into low-dimensional space. This helps to reduce parameters and enhance the network's ability of noise resistance. Next, we concatenate the features corresponding to the encoder-decoder layers and introduce the convolutional block attention module (CBAM) to enhance the network's perception of the critical crack region. Finally, the five-layer features are fused to generate a binary segmentation map of the crack image. We conducted extensive experiments and ablation studies on two real-world crack datasets, and DeepCrackAT achieved 97.41% and 97.25% accuracy on these datasets, respectively. The experimental results show that the proposed method outperforms the current state-of-the-art methods.
更多查看译文
关键词
Crack detection,Attention mechanism,Hybrid dilated convolution,Deep learning
AI 理解论文
溯源树
样例
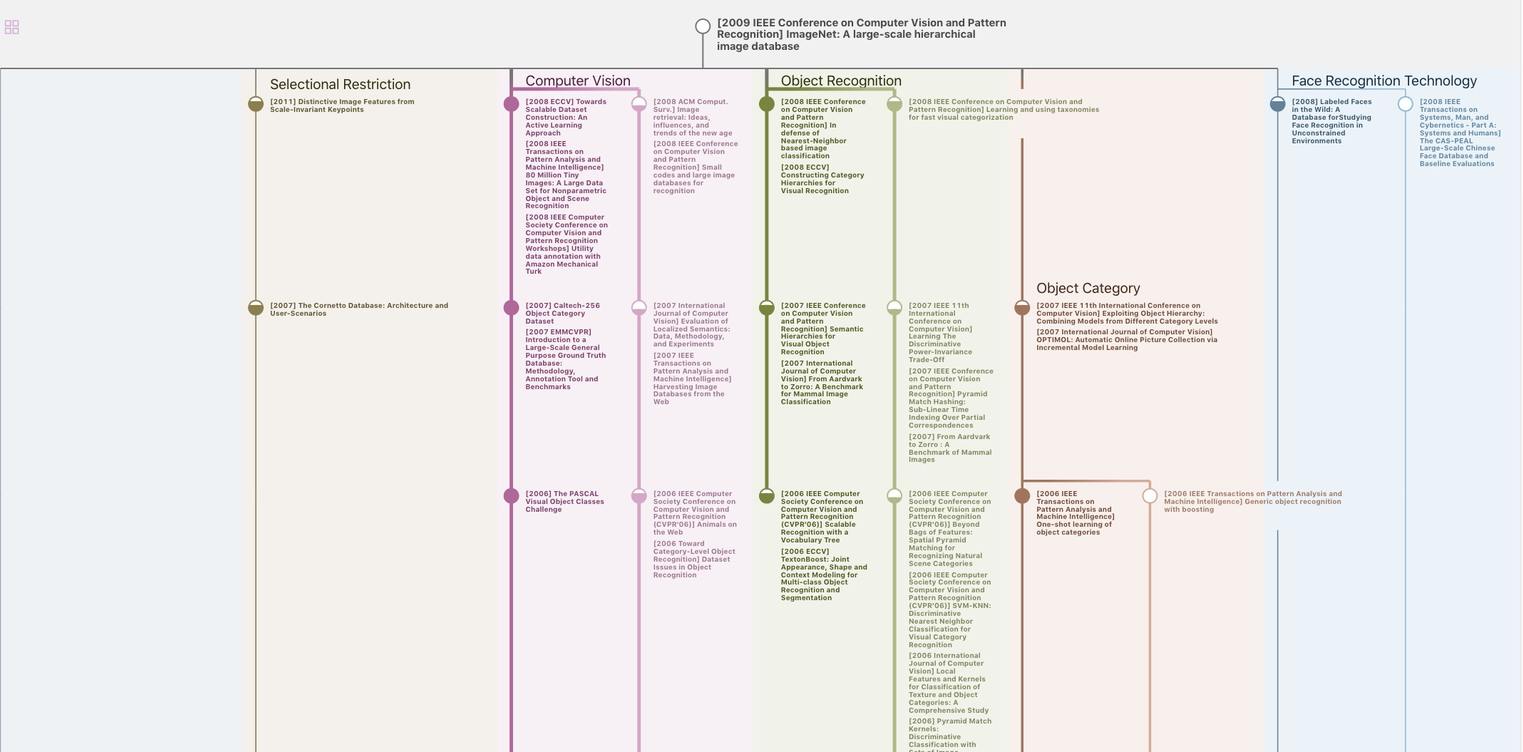
生成溯源树,研究论文发展脉络
Chat Paper
正在生成论文摘要