A K-Net-based hybrid semantic segmentation method for extracting lake water bodies
Engineering Applications of Artificial Intelligence(2023)
摘要
Lakes have a crucial impact on natural disaster prevention, resource recycling, maintenance of agricultural production and daily life. The traditional way of acquiring lake water body information lacks efficiency, is dangerous, and is not suitable for lake water body information acquisition and real-time monitoring. For this reason, the automated lake water body extraction method based on deep learning semantic segmentation model is gradually becoming a mainstream method. However, most of the semantic segmentation models used for lake extraction today express features through static semantics, while ignoring the extraction relationships of different convolutional kernels for these features. In order to better extract lake water bodies from remote sensing images, this paper proposes a hybrid semantic segmentation method based on K-Net, which achieves high accuracy extraction of lake water bodies by introducing dynamic semantic kernels to iteratively refine the feature information. The superiority of the K-Net-based hybrid model on a Google remote sensing image dataset of lakes is validated. The experimental results show that (1) the hybrid model is able to achieve accurate extraction of lake water bodies, with the UperNet + K-Net model using Swin-l performing the best among all six evaluation metrics, with mean intersection over union (mIoU) reaching 97.77%; and that (2) after incorporating the K-Net module, all tested models obtain a larger mIoU than before.
更多查看译文
关键词
K-Net,Semantic segmentation,Water body,Hybrid model
AI 理解论文
溯源树
样例
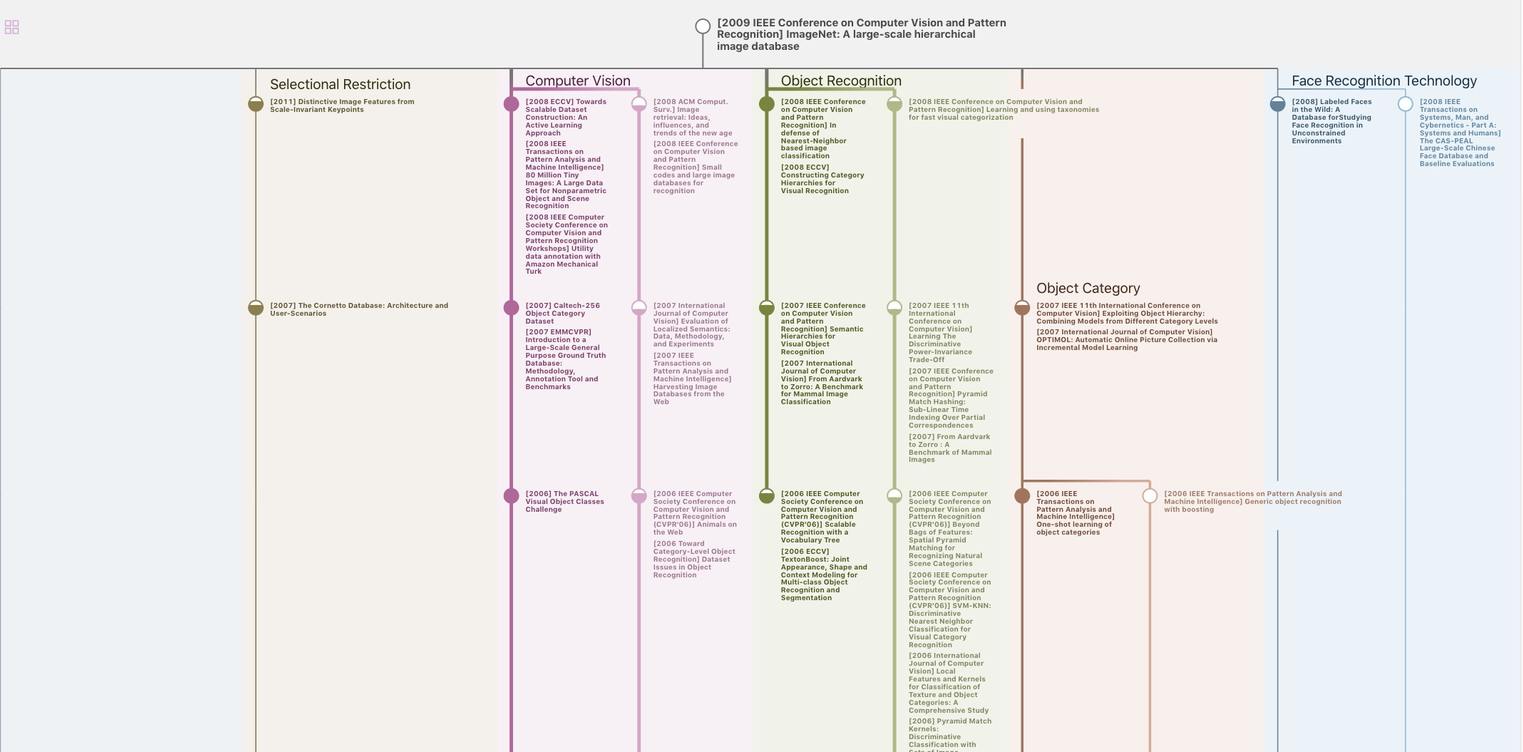
生成溯源树,研究论文发展脉络
Chat Paper
正在生成论文摘要