Body-Pose-Guided Action Recognition with Convolutional Long Short-Term Memory (LSTM) in Aerial Videos
APPLIED SCIENCES-BASEL(2023)
摘要
The accurate detection and recognition of human actions play a pivotal role in aerial surveillance, enabling the identification of potential threats and suspicious behavior. Several approaches have been presented to address this problem, but the limitation still remains in devising an accurate and robust solution. To this end, this paper presents an effective action recognition framework for aerial surveillance, employing the YOLOv8-Pose keypoints extraction algorithm and a customized sequential ConvLSTM (Convolutional Long Short-Term Memory) model for classifying the action. We performed a detailed experimental evaluation and comparison on the publicly available Drone Action dataset. The evaluation and comparison of the proposed framework with several existing approaches on the publicly available Drone Action dataset demonstrate its effectiveness, achieving a very encouraging performance. The overall accuracy of the framework on three provided dataset splits is 74%, 80%, and 70%, with a mean accuracy of 74.67%. Indeed, the proposed system effectively captures the spatial and temporal dynamics of human actions, providing a robust solution for aerial action recognition.
更多查看译文
关键词
deep neural network,convolutional LSTM,action recognition,body pose keypoints,aerial surveillance
AI 理解论文
溯源树
样例
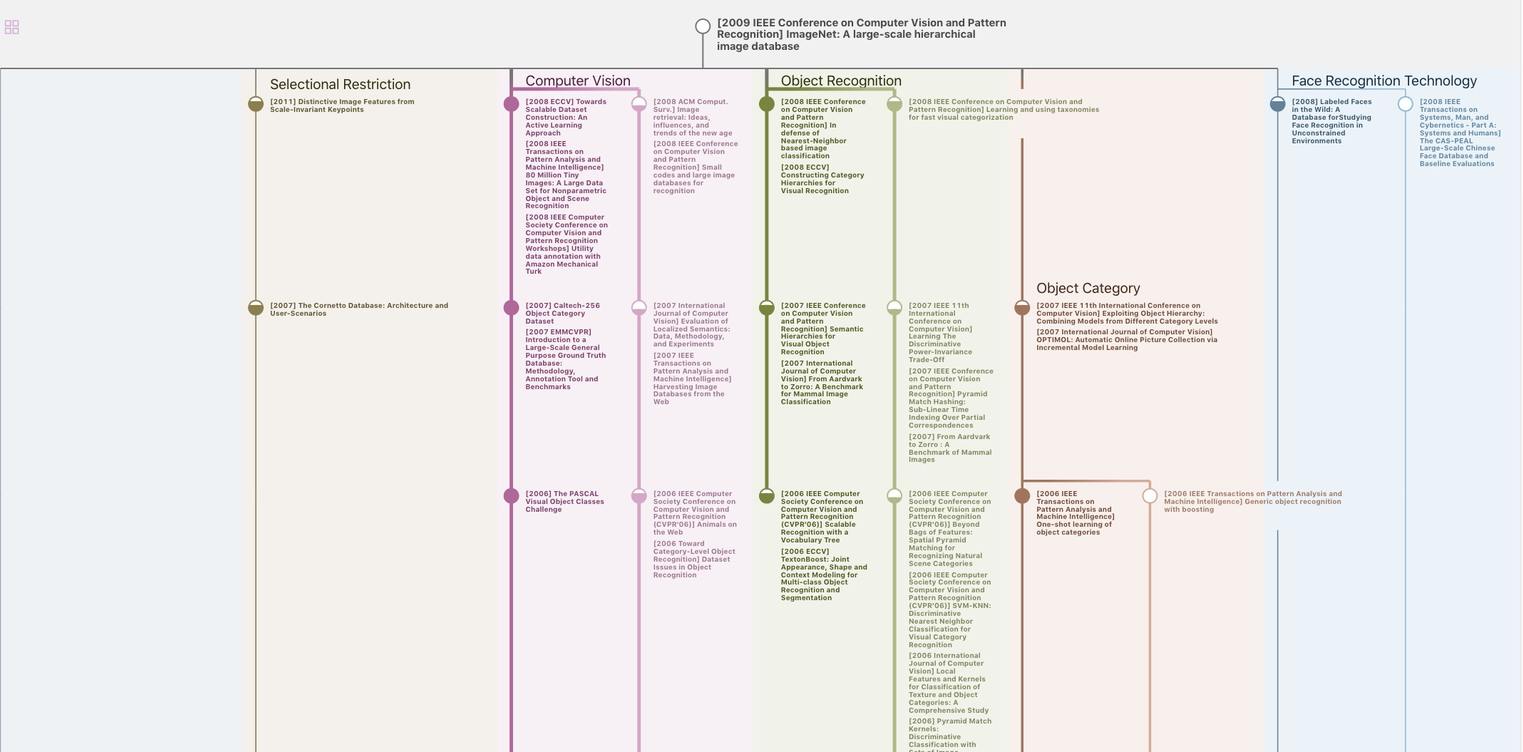
生成溯源树,研究论文发展脉络
Chat Paper
正在生成论文摘要