Indirect Prediction of Salt Affected Soil Indicator Properties through Habitat Types of a Natural Saline Grassland Using Unmanned Aerial Vehicle Imagery
LAND(2023)
摘要
Salt meadows, protected within National Parks, cannot be directly surveyed, yet understanding their soil condition is crucial. Our study indirectly estimates soil parameters (Total Salt Content (TSC), Na, and pH) related to salinization/sodification/alkalinization using spectral indices and UAV survey-derived elevation model, focusing on continental lowland salt meadows. A vegetation map was created using 16 spectral indices and a Digital Elevation Model calculated from RGB orthophotos using photogrammetry. Field observations helped define habitat types based on the General National Habitat Classification System (Hungary), and quadrats with complete coverage of specific plant species were identified. Machine learning was employed on 84 training quadrats to develop a prediction algorithm for vegetation patterns. Five saline habitat types, representing variations in soil properties and topography, were identified. Spectral and topomorphometric indices derived from UAV were key to the spatial prediction of soil properties, employing random forest and co-kriging methods. TSC, Na, and pH data served as indicators of salt-affected soils (SAS), and thematic maps were generated for each indicator (57 samples). Overlapping with the vegetation map, the probability range of estimated SAS indicator values was determined. Consequently, a model-based estimation of soil pH, TSC, and Na conditions is provided for habitat types without disturbing protected areas.
更多查看译文
关键词
protected salt meadows, vegetation map, machine learning methods, UAV, spectral indices, SAS indicator prediction
AI 理解论文
溯源树
样例
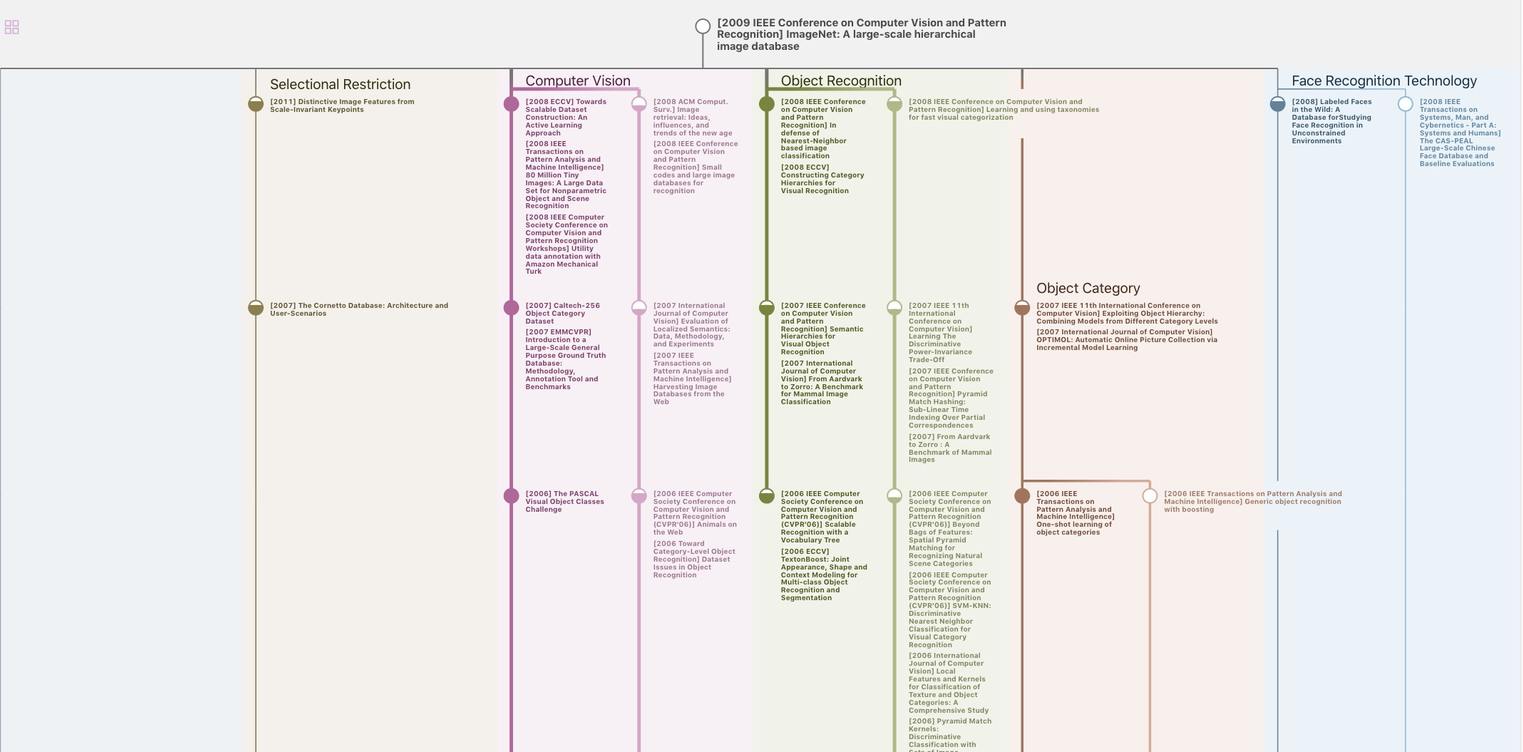
生成溯源树,研究论文发展脉络
Chat Paper
正在生成论文摘要