Self-supervised Distillation for Computer Vision Onboard Planetary Robots
2023 IEEE AEROSPACE CONFERENCE(2023)
摘要
In situ exploration of planets beyond Mars will largely depend on autonomous robotic agents for the foreseeable future. These autonomous planetary explorers need to perceive and understand their surroundings in order to make decisions that maximize science return and minimize risk. Deep learning has demonstrated strong performance on a variety of computer vision and image processing tasks, and has become the main approach for powering terrestrial autonomous systems from robotic vacuum cleaners to self-driving cars. However, deep learning systems require significant volumes of annotated data to optimize the models' parameters, which is a luxury not afforded by in situ missions to new locations in our Solar Sys-tem. Moreover, space-qualified hardware used on robotic space missions relies on legacy technologies due to power constraints and extensive flight qualification requirements (e.g., radiation tolerance), resulting in computational limitations that prevent the use of deep learning models for real-time robotic perception tasks (e.g., obstacle detection, terrain segmentation). In this paper, we address these two challenges by leveraging self-supervised distillation to train small, efficient deep learning models that can match or outperform state-of-the-art results obtained by significantly larger models on Mars image classification and terrain segmentation tasks. Using a set of 100,000 unlabeled images taken by Curiosity and large self-supervised vision models, we distill a variety of small model architectures and evaluate their performance on the published test sets for the MSL classification benchmark and the AI4Mars segmentation benchmark. Experimental results show that on the MSL v2.1 classification task, the best-performing student ResNet-18 model is able to achieve a model compression ratio of 5.2 when distilled from a pretrained ResNet-152 teacher model. In addition, we show that using in-domain images for distillation and increasing the dataset size for distillation has a positive effect on downstream vision tasks. Overall, results indicate that self-supervised distillation enables small models to achieve state-of-the-art performance on the benchmark datasets, supporting the feasibility of performing real-time inference using these small distilled models on next-generation flight hardware such as the High Performance Spaceflight Computer (HPSC).
更多查看译文
关键词
autonomous planetary explorers,autonomous robotic agents,computer vision onboard planetary robots,deep learning systems,high performance spaceflight computer,HPSC,image processing tasks,Mars image classification,next-generation flight hardware,power constraints,robotic space missions,robotic vacuum cleaners,self-driving cars,self-supervised distillation,self-supervised vision models,solar system,space-qualified hardware,terrain segmentation,terrain segmentation,terrestrial autonomous systems
AI 理解论文
溯源树
样例
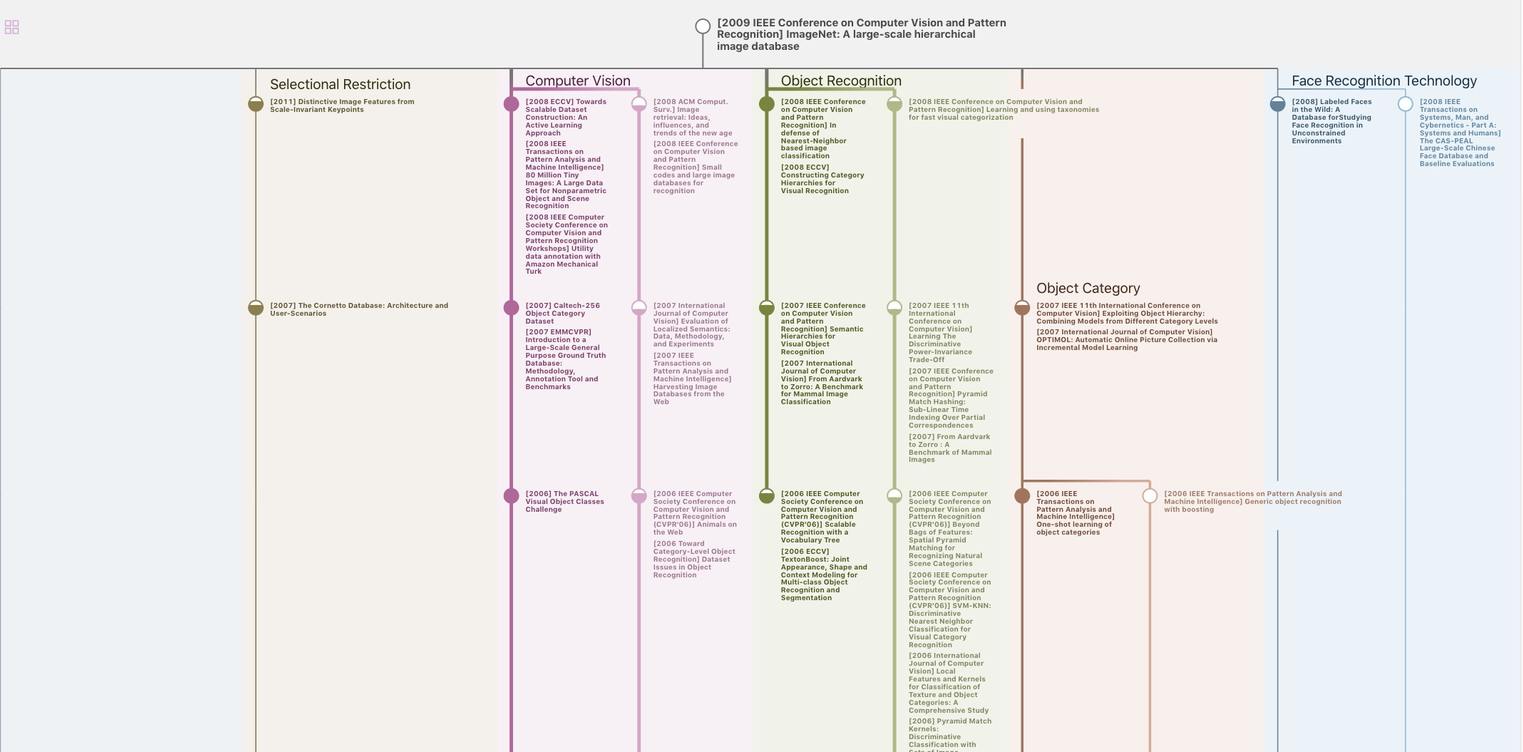
生成溯源树,研究论文发展脉络
Chat Paper
正在生成论文摘要