History Makes the Future: Iterative Learning Control for High-Speed Trains
IEEE INTELLIGENT TRANSPORTATION SYSTEMS MAGAZINE(2024)
摘要
With the development of high-speed rail transportation, the automatic train operation (ATO) of high-speed trains (HSTs) has attracted considerable attention in the fields of both theoretical research and engineering practice. The core task of ATO is trajectory tracking. As an intelligent control method that imitates human learning behavior, iterative learning control (ILC) has been widely applied to various trajectory tracking problems. Owing to the remarkable repetitiveness of HST operation and distinct characteristics of ILC, ILC is regarded as a promising and potential method to track the desired speed trajectory of HSTs. Therefore, various ILC schemes have been designed for HSTs. This article presents a survey on ILC for HSTs and provides an overview of related research results. First, we provide a brief background on ATO and ILC and explain why the ILC application is considered for the ATO of HSTs. Then, we divide the existing ILC schemes for HSTs into two categories, namely, model based and model free, according to whether they are based on the train dynamic model. Based on these schemes, we introduce model-based and model-free ILC, respectively. Next, considering that HST operation is a complex process that may encounter various issues, we introduce extensions of ILC for HSTs to address these issues. The extensions are summarized from three aspects: accurate dynamic models, safety, and diversified control objectives. Finally, we present promising directions for future research. We believe that the results of this survey can provide a general concept of the current progress in applying ILC to HSTs.
更多查看译文
关键词
Trajectory tracking,Task analysis,Rail transportation,Transportation,Trajectory,Surveys,PD control
AI 理解论文
溯源树
样例
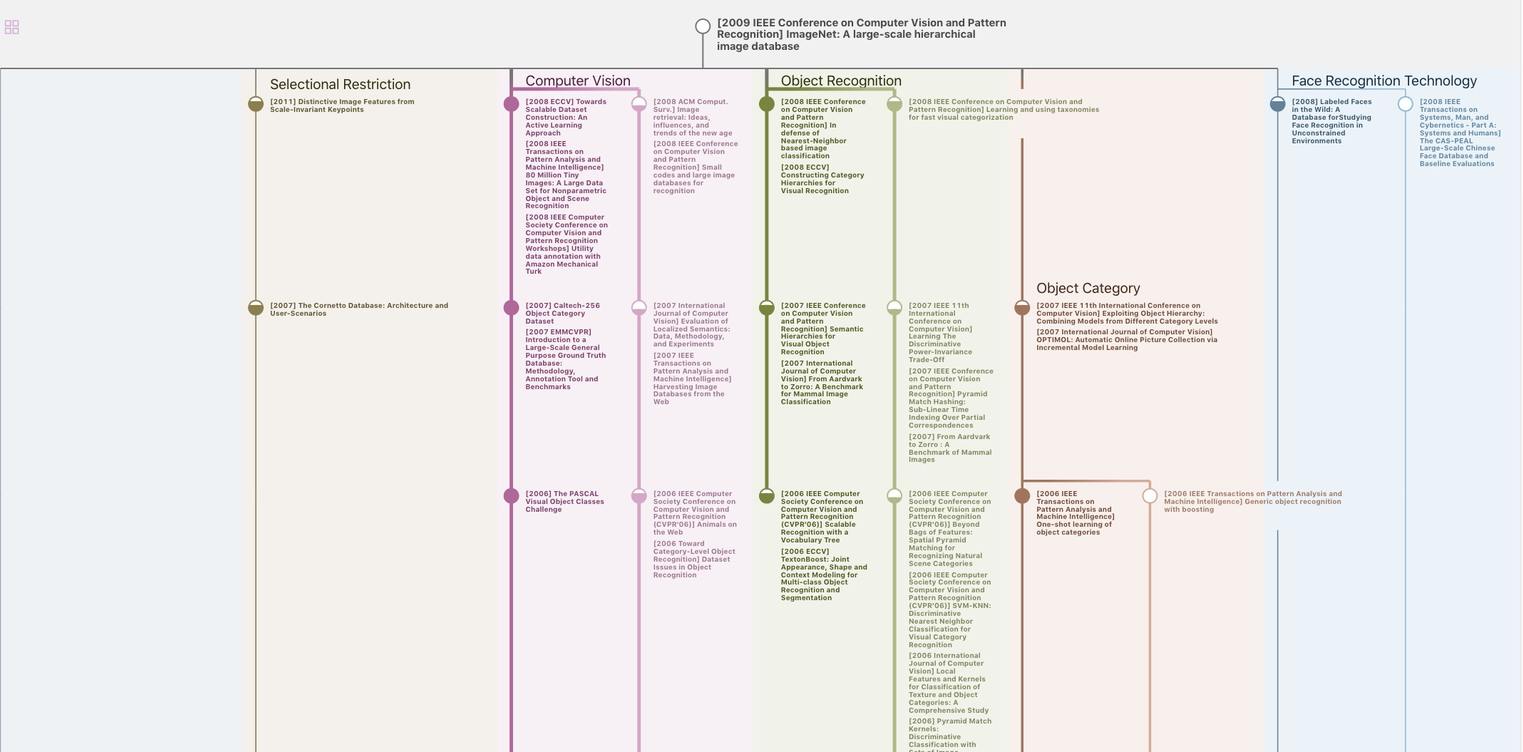
生成溯源树,研究论文发展脉络
Chat Paper
正在生成论文摘要