An edge-aided parallel evolutionary privacy-preserving algorithm for Internet of Things.
Internet Things(2023)
摘要
Data sanitization in the context of Internet of Things (IoT) privacy refers to the process of permanently and irreversibly hiding all sensitive information from vast amounts of stream-ing data. Taking into account the dynamic and real-time characteristics of streaming IoT data, we propose a parallel evolutionary Privacy-Preserving Data Mining (PPDM), called High-performance Evolutionary Data Sanitization for IoT (HEDS4IoT), and implement two mechanisms on a Graphics Processing Units (GPU)-aided parallelized platform to achieve real-time streaming protected data transmission. The first mechanism, the Parallel Indexing Engine (PIE), generates retrieval index lists from the dataset using GPU blocks. These lists are used in place of the dataset during the PPDM process. The second mechanism, called Parallel Fitness Function Engine (PF2E), parallelizes the index lists on the GPU threads to speed up the computation of the quality of solutions generated by the evolutionary algorithm, in which deferential evolution is adopted as the evolutionary algorithm. In addition to the ability for Big data, the HEDS4IoT can be adaptively adjusted for dynamic nature of IoT where new streaming data is considered for data sanitization. Our experimental results with extensive benchmarks show that, at the kernel level, the PIE and PF2E mechanisms are averagely 33.5x and 53.7x faster than their CPU-implemented version, respectively. At the application level, our findings demonstrate that the HEDS4IoT can perform the PPDM process 47.7x faster than some of the state-of-art methods.
更多查看译文
关键词
evolutionary,edge-aided,privacy-preserving
AI 理解论文
溯源树
样例
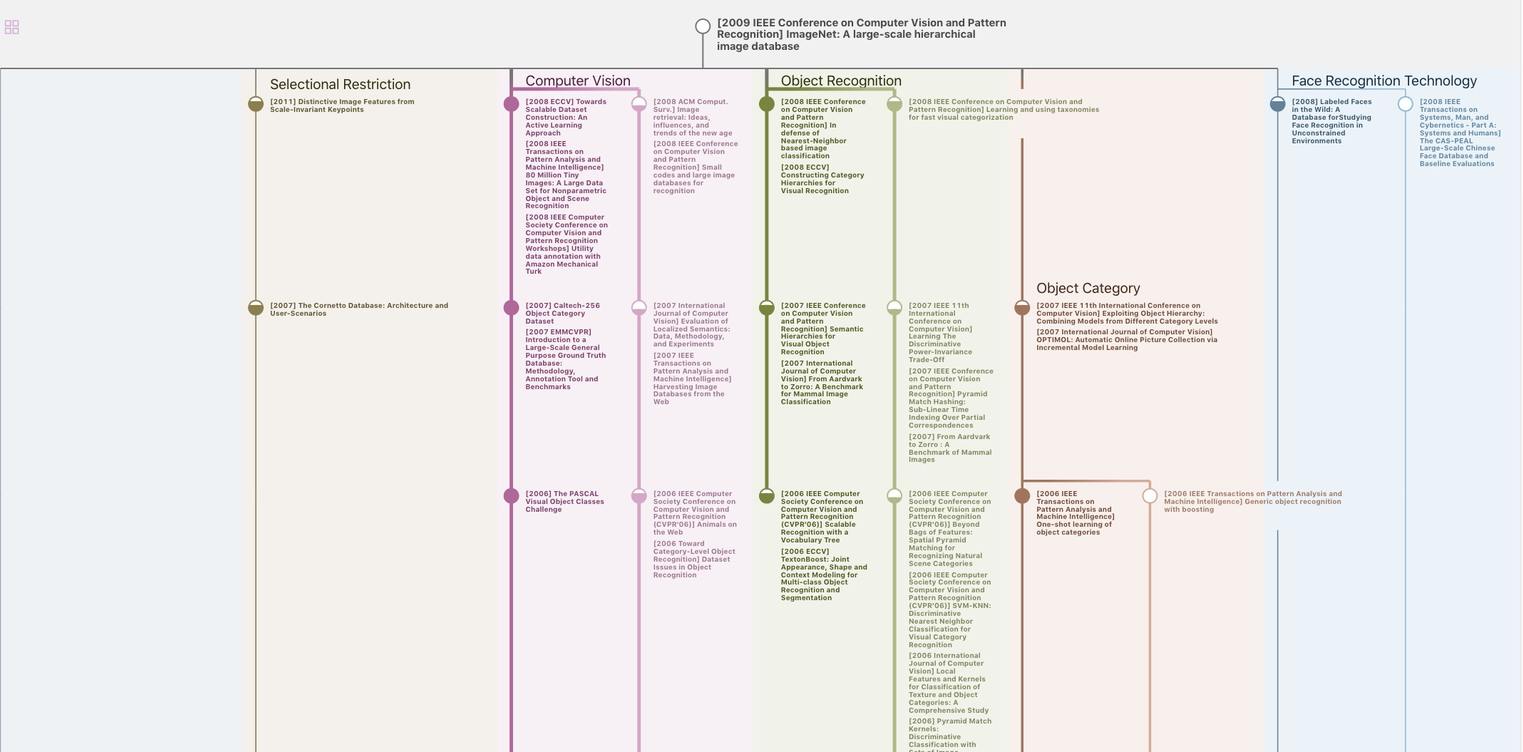
生成溯源树,研究论文发展脉络
Chat Paper
正在生成论文摘要