GHNet: Learning GNSS Heading From Velocity Measurements
IEEE SENSORS JOURNAL(2024)
摘要
By utilizing global navigation satellite system (GNSS) position and velocity measurements, the fusion between the GNSS and the inertial navigation system (INS) provides accurate and robust navigation information. When considering land vehicles, like autonomous ground vehicles, off-road vehicles, or mobile robots, a GNSS-based heading angle measurement can be obtained and used in parallel to the position measurement to bind the heading angle drift. Yet, at low vehicle speeds (less than 2 m/s) such a model-based (MB) heading measurement fails to provide satisfactory performance. This article proposes GHNet, a deep learning framework capable of accurately regressing the heading angle for vehicles operating at low speeds. GHNet utilizes only the current GNSS velocity measurement, from a single GNSS receiver, for the regression task. It is a shallow network utilizing its ability to reduce noise and capture nonlinear behavior. We demonstrate that GHNet outperforms the current MB approach for simulation and experimental datasets. GHNet can be applied to any type of ground vehicles such as passenger cars, autonomous vehicles, autonomous ground vehicles, off-road vehicles, and mobile robots
更多查看译文
关键词
Global navigation satellite system (GNSS),heading estimation,inertial navigation system (INS),INS/GNSS fusion
AI 理解论文
溯源树
样例
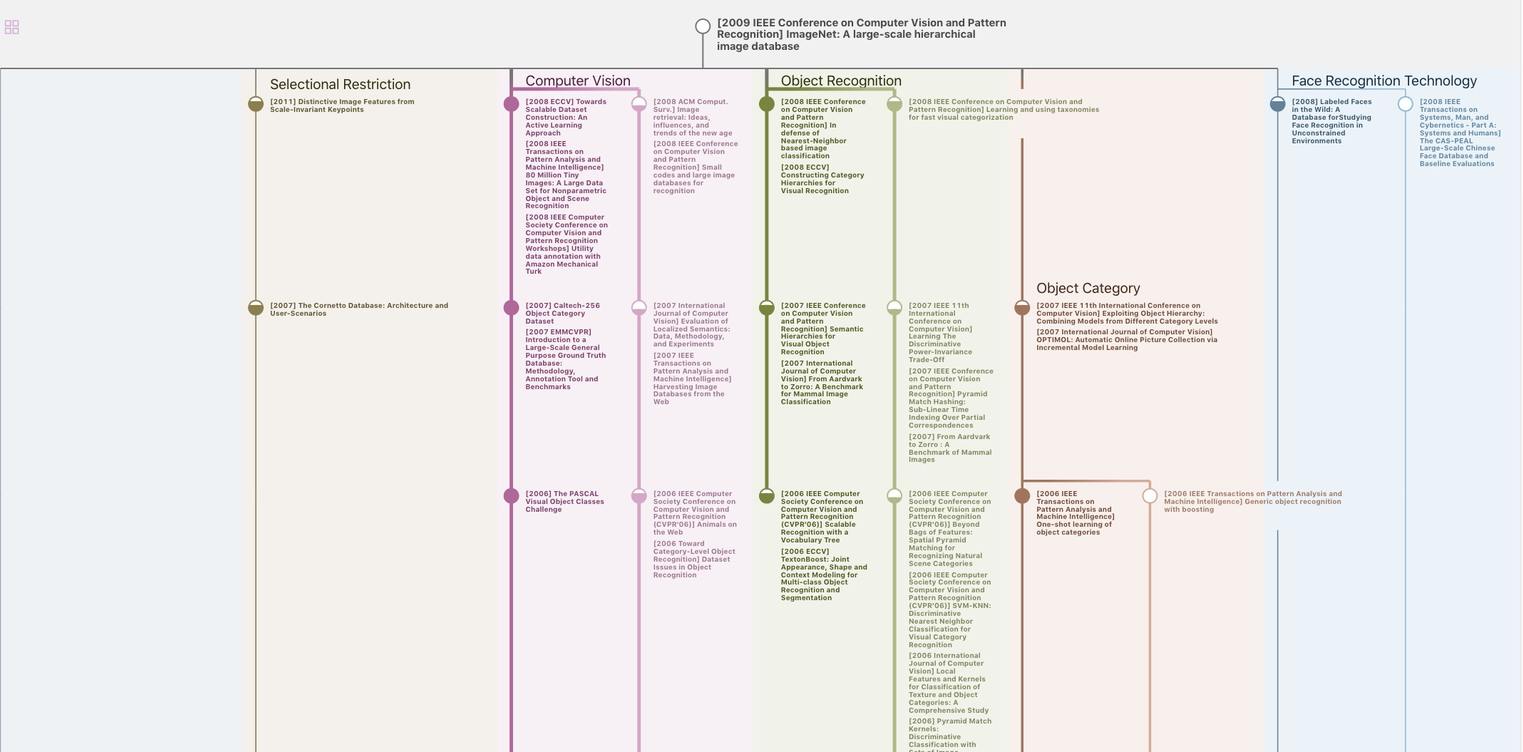
生成溯源树,研究论文发展脉络
Chat Paper
正在生成论文摘要