Construction and testing of a model for predicting droplet deposition based on Gaussian process regression.
Comput. Electron. Agric.(2023)
Abstract
To realize precision spraying, predicting the pesticide application dose based on the structural characteristics of the plant canopy is a crucial step. However, most of the current precision spraying techniques are based on canopy characteristics with no consideration given to the effect of canopy characteristics on droplet particle population. As a result, the droplet deposition value is far from being ideal. Herein, we explored the effects of canopy density and spraying volume on the distribution of droplet deposition in simulated citrus tree canopies and constructed a prediction model for droplet deposition. In this research, the three-dimensional (3D) reconstruction of fruit tree canopy was obtained using binocular camera and a new method was proposed to describe simulated citrus tree canopy density using three-dimensional point cloud. The optimal description of the interrelationship between 3D point cloud and fruit tree canopy density was determined by comparing the determination coefficients, Akaike information criterion and relative errors of the four fitting functions. Through our experiments, a droplet deposition prediction model based on Gaussian process regression was established by changing the duty cycle of the solenoid valve to adjust the spray volume, with simulated citrus trees as the target and water sensitive paper as the sampling method for collecting droplet deposition data. The experimental results show that the distribution of droplet deposition in the upper and lower layers of the canopy varies, but within the same layer droplets can produce relatively uniform deposition in similar canopy density areas with correlation coefficients greater than 0.6. When the canopy density reached 4.35 m2/m3, the amount of droplet deposition was anomalous. The prediction model, after training, has good ability of predicting droplet deposition with R2 higher than 0.79. At the same time, it was found in the research that differences occurred between the prediction accuracy of the droplet deposition prediction model in the upper canopy layer and that in the lower canopy layers, in which the R2 of the prediction model in the lower layer could reach 0.82, while in the upper layer it was 0.79. It is expected that our research on droplet deposition prediction can provide a new research perspective for precision spraying technology in plant protection operations.
MoreTranslated text
Key words
Gaussian process regression,Three-dimensional reconfiguration,Canopy density,Droplet deposition prediction model
AI Read Science
Must-Reading Tree
Example
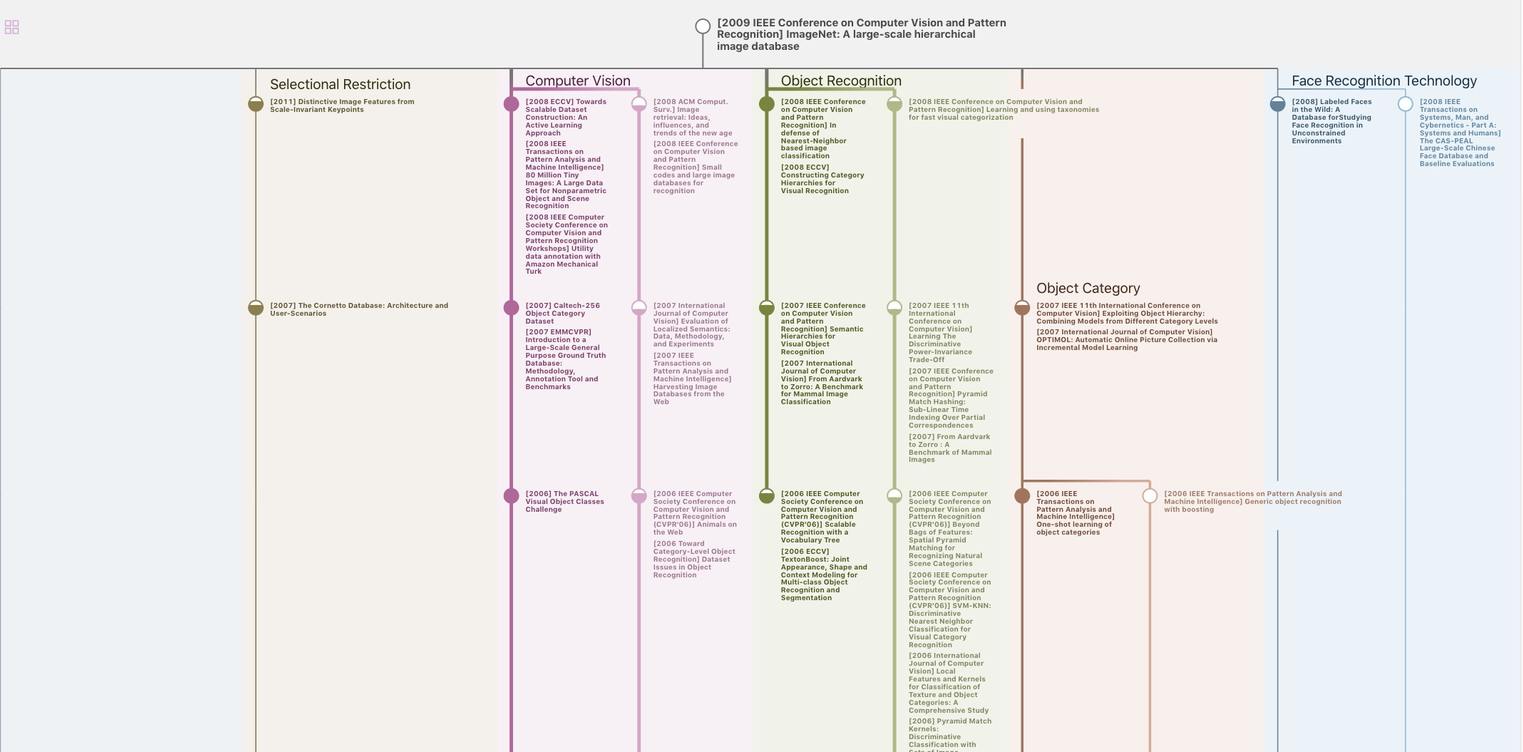
Generate MRT to find the research sequence of this paper
Chat Paper
Summary is being generated by the instructions you defined