Towards evaluating robustness of violence detection in videos using cross-domain transferability.
J. Inf. Secur. Appl.(2023)
摘要
Recent studies have demonstrated the applicability of classification models based on deep learning for detecting human action. For this reason, automatic violence detection from videos has become a crying need to prevent the spreading of vulnerable content on digital platforms. In spite of the remarkable success of these neural networks, they are prone to fail against adversarial attacks and thus, highlighted the need for evaluating the robustness of these state-of-the-art violence detection classifiers. Here, we propose a transferable logit attack for binary misclassification of video data which can evade the system by our spatially perturbed synthesized adversarial samples. We utilize the adversarial falsification-based threat model to validate a non-sparse white box attack setting which will generate cross-domain adversarial video samples by perturbing only spatial features without affecting the temporal features. We carry out extensive experiments on the validation set of two popular violence detection datasets: Hockey Fight Dataset, and Movie Dataset and verify that our proposed attack method has high attack success rate for these datasets against the state-of-the-art violence detection classifier. This work aims to make future violence detection models more resistant to adversarial examples.
更多查看译文
关键词
Video adversarial attacks,Violence misclassification,White-box attack,Non-sparse attack,Transferable attacks,Adversarial falsification threat model
AI 理解论文
溯源树
样例
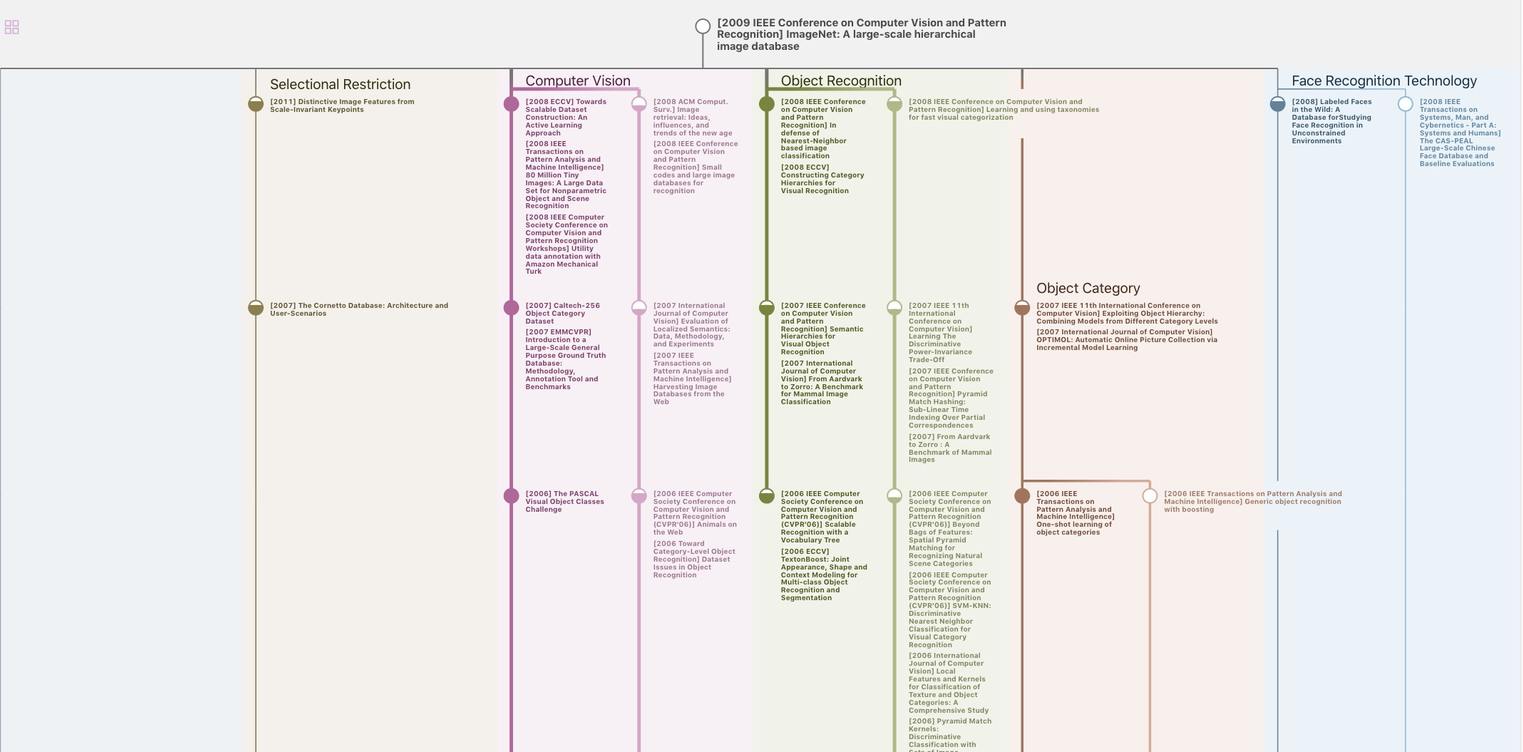
生成溯源树,研究论文发展脉络
Chat Paper
正在生成论文摘要