Nonnegative matrix factorization constrained by multiple labelled spanning trees for label propagation
Inf. Sci.(2023)
摘要
Label propagation is an important semi-supervised learning method that generalizes the attributes of labelled samples to unlabelled samples based on the correlation of the data distribution. However, handling data containing imbalanced labels, multiple subclasses, bridging points, and nonlinear distributions is challenging. These challenges often make it difficult for existing methods to learn accurate relationships between labelled and unlabelled samples. In this paper, we propose multiple labelled spanning trees (MLSTs). Each labelled sample is initialized as the root node of a tree, and all trees are grown asynchronously according to the manifold structure of their neighbours such that all of the unlabelled samples can establish a connection with the labelled samples. The MLST construction method can better adapt to different disturbances. In addition, MLSTs-constrained nonnegative matrix factorization (MLSTs-NMF) is proposed for label propagation; it constructs a global relationship by using nonlinear representation with labelled samples and combines the manifold structure information from multiple labelled spanning trees to comprehensively estimate the labels of unlabelled samples. The results of the experiments demonstrate that the prediction accuracy of the proposed method is higher than that of the existing methods, and it exhibits better robustness to various interferences.
更多查看译文
关键词
Label propagation,Multiple labelled spanning trees,Nonnegative matrix factorization,Graph construction
AI 理解论文
溯源树
样例
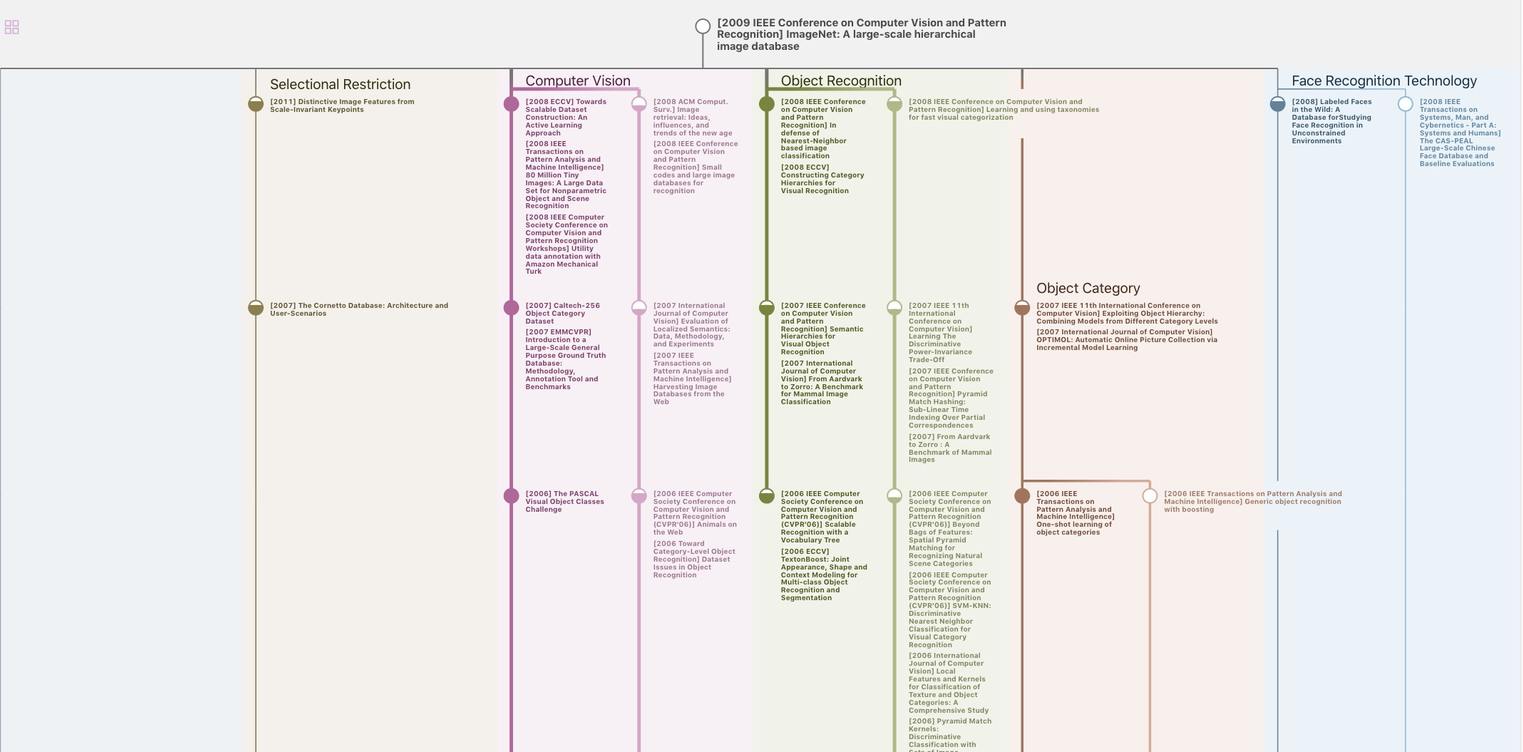
生成溯源树,研究论文发展脉络
Chat Paper
正在生成论文摘要