Data-driven templates with dictionary learning and sparse representations for photometric redshift estimation.
Astron. Comput.(2023)
摘要
The quality of photometric redshift estimates is fundamental for cosmological applications, as the constraints on the cosmological parameters obtained by the photometric surveys strongly rely on their precision and accuracy. In order to obtain reliable redshift estimates, a large number of studies have proposed different algorithms based on SED template fitting and machine learning methodologies. While template fitting strategies do not need spectroscopic data for training, they also provide consistent estimates in most scenarios. On the other hand, machine learning-based approaches succeed at building a mapping function between the input space (composed by magnitudes and other physical parameters) and the target photometric redshift space. These empirical methods lead to more accurate results as long as the spectroscopic ground truth properly represents the actual galaxy population distribution. Thus, combining template fitting and machine learning techniques would allow to leverage the advantages of both methodologies yielding hybrid methods. The goal is to provide a good estimation accuracy while maximizing the photometric range coverage. This is particularly important in the high -z regime, where the spectroscopic training sample is rarely available. We propose in this article a new method to derive data-driven templates from simulated spectra using dictionary learning. As these representations are obtained directly from the data, they allow to capture the physical properties of the galaxies better than theoretical templates. Inspired by hybrid algorithms, the dictionary is built hierarchically and the features of the different types of galaxies are separated. Once the dictionary has been created, the data-driven templates are artificially redshifted and the observed spectra are sparsely decomposed on this dictionary. The value providing the minimum reconstruction error is selected as the photometric redshift estimate. This new technique, astride template fitting and machine learning, builds representations for the galaxy spectra through unsupervised learning and computes the photometric redshifts by & chi;2 minimization. The performance of the algorithm has been evaluated on realistic galaxy photometric simulations as well as on real data from the Canada-France-Hawaii Telescope Lensing Survey. & COPY; 2023 Elsevier B.V. All rights reserved.
更多查看译文
关键词
dictionary learning,sparse representations,estimation,data-driven
AI 理解论文
溯源树
样例
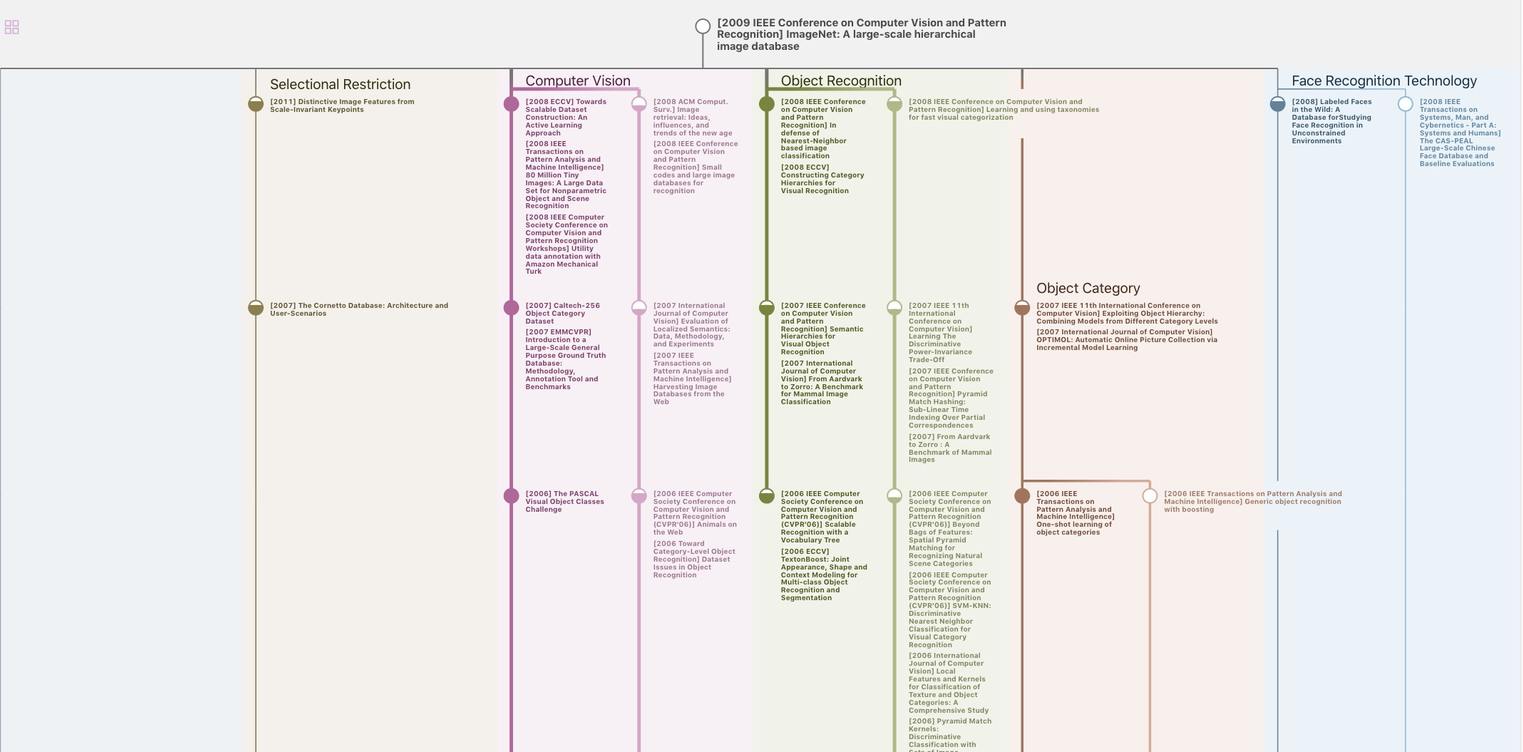
生成溯源树,研究论文发展脉络
Chat Paper
正在生成论文摘要