Dictionary Learning-Guided Deep Interpretable Network for Hyperspectral Change Detection.
IEEE Geosci. Remote. Sens. Lett.(2023)
摘要
Hyperspectral image (HSI) change detection is a technique to observe the change information between the multitemporal HSIs, which is currently considered a major focus of research in the field of remote-sensing intelligent interpretation. Most existing deep-learning-based methods have created satisfactory performance, but these methods lack transparency and have poor generalization. To tackle the problems outlined above, we propose a dictionary learning-guided deep interpretable network (DLDINet) for hyperspectral change detection, which unfolds a dictionary learning-based change detection model into an interpretable deep neural network. Specifically, we first design a dictionary learning-based change detection model, whose solution process can be decomposed into two iterative subproblems. Then, the mathematical model can be unfolded into a dual-branch deep neural network with two modules iterating with each other. Finally, the difference map of the coefficient's output from the ultimate stage is classified to obtain the change detection result. Experimental results prove that the proposed method has comparable or even better performance than state-of-the-art methods.
更多查看译文
关键词
Dictionaries, Iterative methods, Feature extraction, Hyperspectral imaging, Noise reduction, Learning systems, Decoding, Change detection, deep neural network, dictionary learning, hyperspectral image (HIS), interpretable
AI 理解论文
溯源树
样例
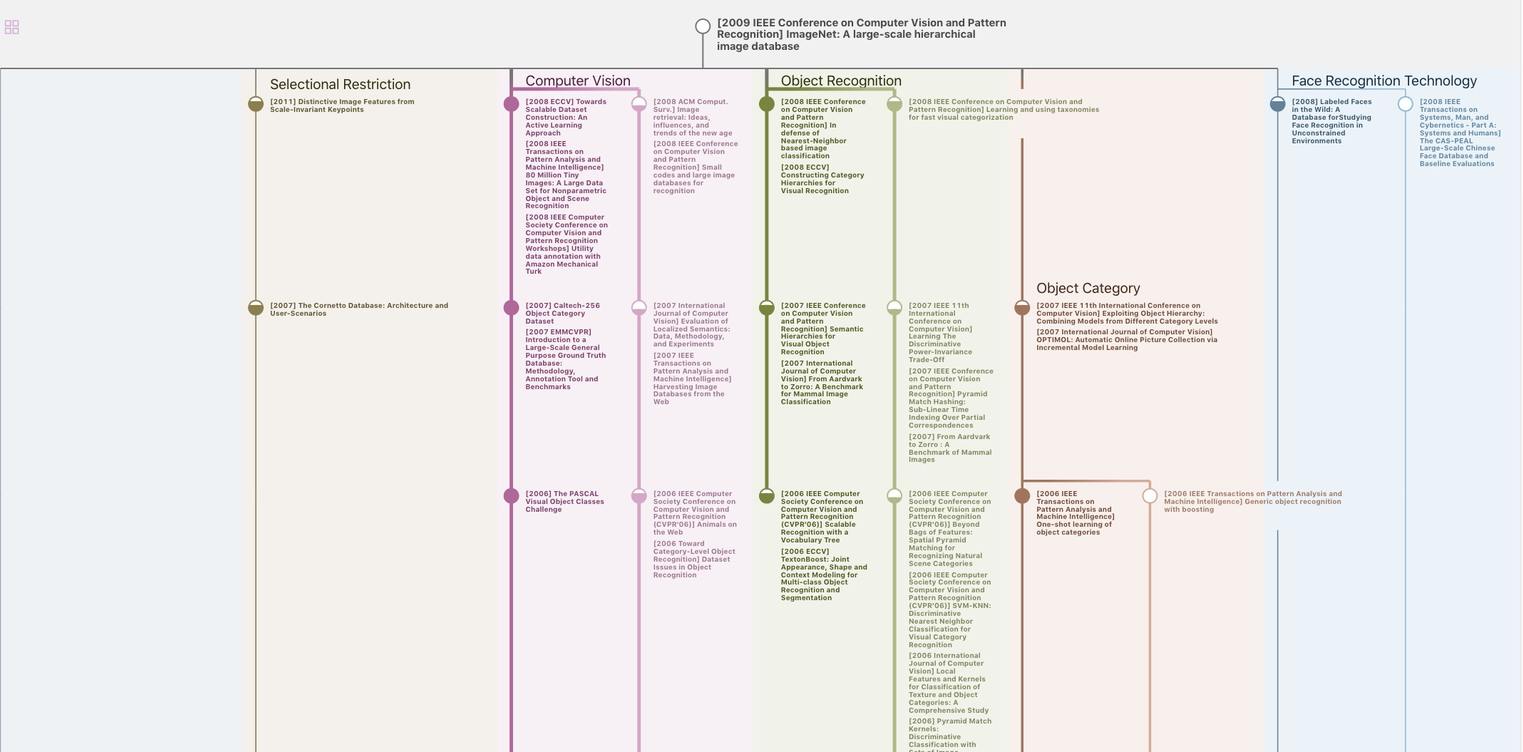
生成溯源树,研究论文发展脉络
Chat Paper
正在生成论文摘要