Convolutional Transformer-Inspired Autoencoder for Hyperspectral Anomaly Detection.
IEEE Geosci. Remote. Sens. Lett.(2023)
摘要
Hyperspectral anomaly detection (HAD) plays a vital role in military and civilian applications. However, compared with target detection or classification tasks, HAD is more challenging due to insufficient anomaly information and the difficulty of extracting local and global discriminative features. In this letter, a convolutional transformer-inspired autoencoder (CTA) is proposed for HAD. The CTA consists of a clustering-based module and an autoencoder-based module. First, note that the number of anomalies is small, and distinct from their surroundings, a clustering-based module is proposed to detect the pseudo-background and anomaly samples. Second, the autoencoder module is composed of an encoder and a decoder formed from several skip-connected convolutions and multihead attention-based transformers. The CTA is trained not only to distinguish the anomalies from the background but also to reconstruct the input hyperspectral images (HSIs). Benefiting from integrating the convolution and transformer, the CTA has local and global receptive fields. Moreover, both background and anomaly information explored by the clustering-based module can be adopted to improve the separability of anomalies. Experiments on two hyperspectral datasets demonstrate that the proposed CTA achieves superior detection performance to its counterparts. The code is available at https://github.com/hzhdhz/CTA.
更多查看译文
关键词
Anomaly detection,autoencoder,deep learning,hyperspectral image (HSI),transformer
AI 理解论文
溯源树
样例
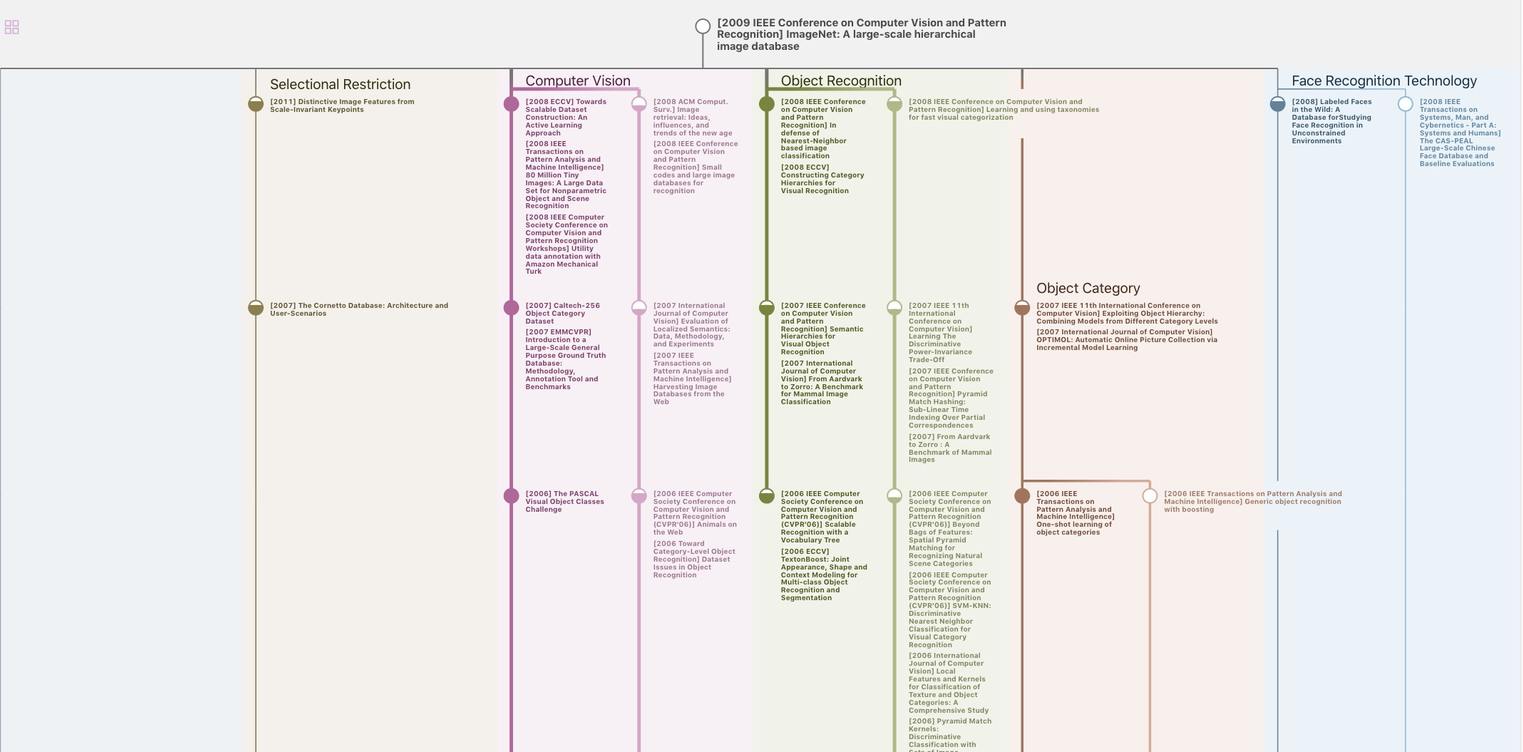
生成溯源树,研究论文发展脉络
Chat Paper
正在生成论文摘要