Semisupervised Edge-Aware Road Extraction via Cross Teaching Between CNN and Transformer.
IEEE J. Sel. Top. Appl. Earth Obs. Remote. Sens.(2023)
摘要
Semisupervised semantic segmentation of remote sensing images has been proven to be an effective approach to reduce manual annotation costs and leverage available unlabeled data to improve segmentation performance. However, some existing methods that focus on self-training and consistent regularization fail to consider large-scale characteristics of remote sensing images and the importance of incorporating road edge information. In this article, we propose a novel semisupervised edge-aware network (SSEANet) for remote sensing image semantic segmentation by jointly training convolutional neural network and transformer. SSEANet focuses on the consistency loss of multiscale features and uses an attention mechanism to fuse road edge information. Extensive experiments on DeepGlobe, Massachusetts, and AerialKITTI-Bavaria datasets show that the proposed method outperforms state-of-the-art, demonstrating its effectiveness.
更多查看译文
关键词
Cross teaching (CT), edge-aware, road extraction, semisupervised learning, transformer
AI 理解论文
溯源树
样例
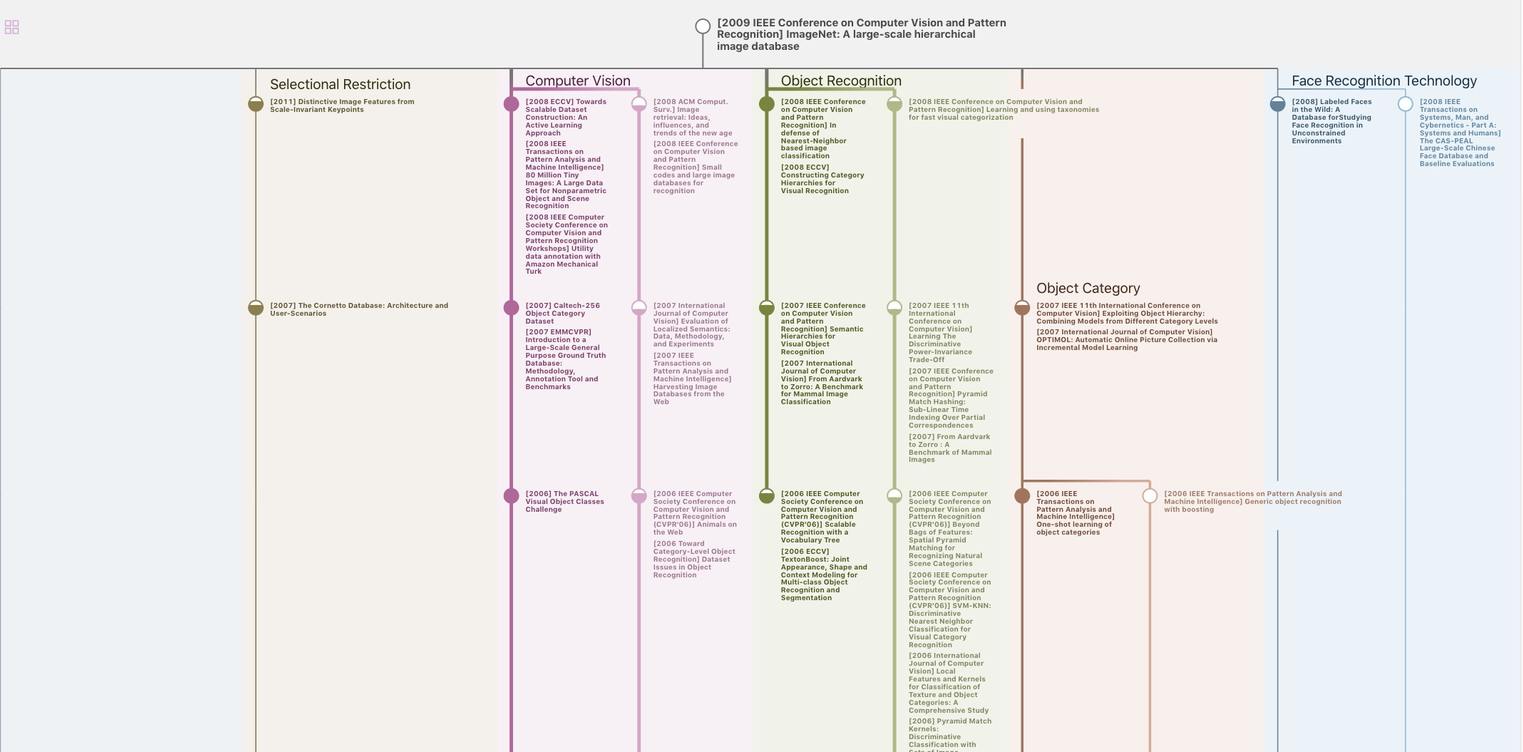
生成溯源树,研究论文发展脉络
Chat Paper
正在生成论文摘要