Learning-driven ubiquitous mobile edge computing: Network management challenges for future generation Internet of Things.
Int. J. Netw. Manag.(2023)
摘要
Ubiquitous edge computing facilitates efficient cloud services near mobile devices, enabling mobile edge computing (MEC) to offer services more efficiently by presenting storage and processing capability within the proximity of mobile devices and in general IoT domains. However, compared with conventional mobile cloud computing, ubiquitous MEC introduces numerous complex challenges due to the heterogeneous smart devices, network infrastructures, and limited transmission bandwidth. Processing and managing such massive volumes of data generated from these devices is complex and challenging in edge infrastructures. On the other side, time-critical applications have stringent requirements such as ultra-low-latency, energy cost, mobility, resource, and security issues that cannot be neglected. For example, smart healthcare or industrial networks generate emergency information very frequently (i.e., often in terms of milliseconds), which needs to be processed near the sensing devices with minimal processing delay. In this context, future generation IoT requires robust and intelligent network management approaches that can handle the system complexity (e.g., scalability and orchestration) with little or no little human intervention and offer a better service to end-users. More precisely, AI/ML approaches designed explicitly for networks under high traffic volume of data help overcome several management challenges, such as (i) improving performance by balancing load and traffic, (ii) distributing the bandwidth spectrum based on demand, and (iii) traffic predictions. Moreover, this need also opens several new research directions such as new MEC architecture, service provisioning technique, security mechanisms, advanced 5G or beyond communication technology, ambient intelligence, and AI/ML-based solutions. This issue collect surveys and contribution articles on emerging trends and technologies in ubiquitous MEC for future generation IoT networks and their managements. The papers related to machine learning, deep learning, optimization, blockchain, 5G, or beyond solutions, especially for domain-specific IoT network management, which use MEC environments, are collected after evaluating the review process. Each paper submitted to this special issue was reviewed by three to seven experts during the assessment process. At the end we consider one survey paper and four research contributions. The first paper Ravi et al. proposed a survey on “Stochastic modeling and performance analysis in balancing load and traffic for vehicular ad hoc networks.” This survey presents recently published stochastic modeling-based algorithms for VANETs. This article briefly covers various queueing models for the reader's convenience. This paper discusses a variety of VANET issues such as mobility, routing, data dissemination, cooperative communication, congestion control, and traffic load balancing issues addressed by stochastic modeling techniques. The authors provided extensive open challenges for young researchers on several network management topics such as zero-touch provisioning, blockchain, intelligent digital twin, cooperative communication among vehicles, and routing predictions according to vehicle mobility. These topics are emerging in this area, and they are open to further research. The second paper Song et al. contributed on “A group key exchange and secure data sharing based on privacy protection for federated learning in edge-cloud collaborative computing environment.” The proposed work aims to secure the transmission of model parameters between IoT terminals during a federated learning process. With the key self-verification algorithm, the model legitimizes the public and private keys of the terminal, which greatly enhances their security. In order to protect against terminal identity leakage, Song et al. provide an attribute-based cryptographic method; the terminal generates ciphertext attributes and gives them to the cloud server. The security of sharing the parameters of each model in the FL process is guaranteed. Terminal self-adaptive is achieved by defining access structures to meet shared resource access rights. Upon satisfying the access authority, each terminal stores and downloads the shared ciphertext model parameters from the edge-cloud server. Third, Arora et al. contributed a paper entitled “Blockchain-inspired lightweight trust-based system in vehicular networks.” This paper proposes a trust-based vehicle registration paradigm that uses certificate authorities. Further a blockchain-based system is used to provide efficient two-way authentication and key agreement through encryption and digital signatures. As a result, the proposed scheme is efficient for establishing distributed trust management, which offers privacy protection for vehicles. The source code for the proposed scheme is openly available in Github for the readers to explore further. Fourth, Cao et al. contributed a paper on “Dynamic management network system of automobile detection applying edge computing.” This paper proposes an architectural architecture to detect vehicle deviation dynamically through greedy methods and a mixed-integer linear programming. In this architecture, the authors used various sensors to acquire data from vehicles and edge computing to detect deviation. This model can quickly analyze any vehicle's deviation and control congestion during data transmissions. Fifth, Jafar et al. proposes “A blockchain-enabled security management framework for mobile edge computing,” which guarantees secure data storage and includes blockchain features like immutability and traceability. The framework also includes a smart contract-based access and sharing mechanism, and simulations show that it offers high security, low latency, and low operations cost for resource-constrained MEC devices. Data offloading is one of the primary challenges in edge computing, and it is more complex in MEC. These devices benefit from efficient data offloading by improving performance, reducing latency, and conserving energy. To address this challenge, Samriya et al. proposed “Secured data offloading using reinforcement learning and Markov decision process in mobile edge computing.” In this paper, a reinforcement learning-based Markov decision process offloading model is proposed to overcome computational complexities, secured and efficient offloading. This work optimizes energy efficiency, and mobile users' time by considering the constrained computation of IoT devices, and also ensures efficient resource sharing among multiple users. Seventh, Singh et al. proposed “Load balancing clustering and routing for IoT-enabled wireless sensor networks,” in which an energy-efficient and reliable routing protocol is designed using fuzzy C-means and grey wolf optimization. This approach confirms improvements in different parameters such as total energy consumed, packet delivery ratio, packet drop rate, throughput, delay, remaining energy, and total network lifetime. The authors provide their simulation code open through Github (https://github.com/Shanky197197/WSN-code) to the researchers for further exploration. Lastly, Babu et al. contributed an article entitled “Fog-Sec: Secure end-to-end communication in fog-enabled IoT network using permissioned blockchain system.” This paper shows a proof of concept to secure the fog-based IoT nodes with device-to-device communication through blockchain system. In this paper, blockchain is used primarily to achieve localized authentication, which further reduces latency and increases throughput. The results of this work confirm the superiority of the proposed Fog-Sec in terms of time complexity, and efficiency such as low latency and high throughput. The source code for the proposed Fog-Sec is available for readers on request. Readers can access it through the link https://drive.google.com/drive/folders/16jbzCrBbaBhbm-dwZmMxfFXg_jOO-Hen?usp=share_link or Reference 31 in the paper. We believe that these eight papers make significant contributions and offer valuable insights into the challenges and opportunities of ubiquitous MEC. As a result of this special issue, we hope that more research will be conducted in this area to address network management challenges for the future generation of IoT through ubiquitous MEC and various learning models. We are extremely grateful to academic experts who voluntarily gave their time and contributed works to maintaining the high quality standards of the Journal. We would like to sincerely thank all reviewers who participated in the manuscript assessment process and timely returning the review reports to made decisions timely for this special issue. We sincerely thank Prof. James Won-Ki Hong (EiC), Prof. Lisandro Zambenedetti Granville (Associate EiC), and Prof. Jérôme François (Associate EiC). We also thank the editorial office of the IJNM, Wiley Journal who were instrumental in providing a rapid and efficient editorial process. The guest editors declare that they have no known competing financial interests or personal relationships that could have appeared to influence the work reported in this paper. Dr. Praveen Kumar Donta (Senior Member, IEEE and Professional Member, ACM) is currently working at Distributed Systems Group, TU Wien. He received his Ph.D. from the Indian Institute of Technology (Indian School of Mines), Dhanbad, from the Department of Computer Science and Engineering. He is a visiting Ph.D. fellow at Mobile & Cloud Lab, Institute of Computer Science, University of Tartu, Estonia, under the Dora plus grant. He received his Master in Technology and Bachelor in Technology from the Department of Computer Science and Engineering at JNTUA, Ananthapur, with Distinction in 2014 and 2012. He elevated as a senior member of IEEE (Young Professional, Computer Society, Communication Society, Sensors Council, Systems Council, etc.) and professional member in ACM. He serves as an editorial board member for PLOS One, Computer Communications, Measurements, and Measurement: Sensors journals. His current research on learning-driven distributed computing continuum systems and data lakes. Prof. Edmundo Monteiro (Senior Member, IEEE) is a full professor at the Department of Informatics Engineering (DEI) of the University of Coimbra (UC), Portugal. He is also a senior member of the Research Centre for Informatics and Systems of the University of Coimbra (CISUC). He graduated in Electrical Engineering (Informatics Specialty) from the University of Coimbra in 1984 and received his Ph.D. in Informatics Engineering (Computer Communications) and the Habilitation in Informatics Engineering from the same university in 1996 and 2007 respectively. He has more than 25 years of research and industry experience in the field of Computer Communications, Wireless Technologies, Quality of Service and Experience, Network Management, and Computer Security. He participated in many Portuguese and European research projects and initiatives. His publications include six books (authored and edited), several book chapters and journal publications, and over 200 papers in national and international refereed conferences. He is also co-author of nine international patents. He is a member of the editorial board of Springer Wireless Networks journals and involved in the organization of many national and international conferences and workshops. Edmundo Monteiro is a member of Ordem dos Engenheiros (the Portuguese Engineering Association) and a senior member of IEEE Communication and Computer Societies and ACM SIGCOMM. Dr. Chinmaya Kumar Dehury (Member, IEEE) is an assistant professor of Distributed Systems, member of Mobile & Cloud Lab in the Institute of Computer Science, University of Tartu, Estonia. He received his Ph.D. degree in the Department of Computer Science and Information Engineering, Chang Gung University, Taiwan. His research interests include scheduling, resource management and fault tolerance problems of cloud, fog computing, and clustered edge computing, IoT, and data management frameworks. He is a member of IEEE and ACM India. He is also serving as a PC member of several conferences and reviewer to several journals and conferences, such as IEEE TPDS, IEEE JSAC, IEEE TCC, IEEE TNNLS, and Wiley Software: Practice and Experience. Dr. Ilir Murturi (Member, IEEE) is a Postdoctoral Researcher in the Distributed Systems Group, TU Wien, Austria. He received a Ph.D. in the Distributed Systems Group, Technische Universität Wien (TU Wien), Vienna, Austria, and an M.Sc. in Computer Engineering from the Faculty of Electrical and Computer Engineering, University of Prishtina, Prishtina, Kosova. He is a member of IEEE and a reviewer for several journals and conferences. His current research interests include the Internet of Things, distributed computing continuum systems, EdgeAI, and privacy in distributed, self-adaptive, and cyber-physical systems.
更多查看译文
关键词
ubiquitous mobile edge computing,future generation internet,network management challenges
AI 理解论文
溯源树
样例
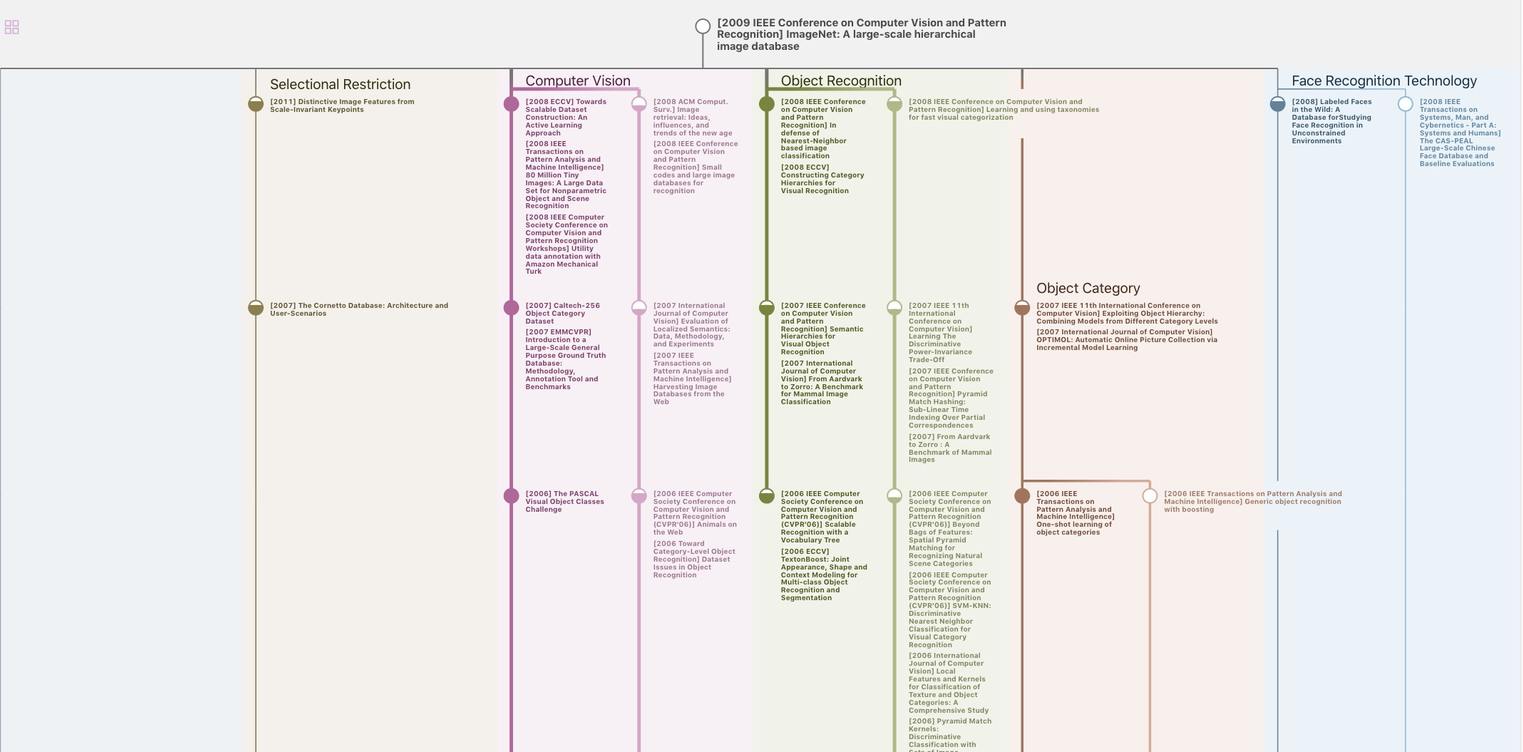
生成溯源树,研究论文发展脉络
Chat Paper
正在生成论文摘要