A novel model for tourism demand forecasting with spatial–temporal feature enhancement and image-driven method
Neurocomputing(2023)
摘要
Accurately forecasting tourism demand requires learning the spatial–temporal features of tourism demand, which is challenging due to constantly changing human behavior. This study presents a spatial–temporal feature enhancement model designed to maintain the integrity of tourism demand features. Specifically, the tourism system is modeled as an undirected graph and the steady-state analysis method is employed to learn spatial–temporal features. To enhance the feature learning ability for sparse features, we employ convolutional filters, and we convert the feature series into an image series while preserving the relationship of the spatial–temporal features. The method’s effectiveness is demonstrated using the digital footprints of tourists from the urban area of Zhuhai. Numerical experiments indicate that the proposed model outperforms state-of-the-art tourism demand forecasting models.
更多查看译文
关键词
Deep learning,Feature enhancement,Tourism demand forecasting,Spatial series to image series,Spatial–temporal learning
AI 理解论文
溯源树
样例
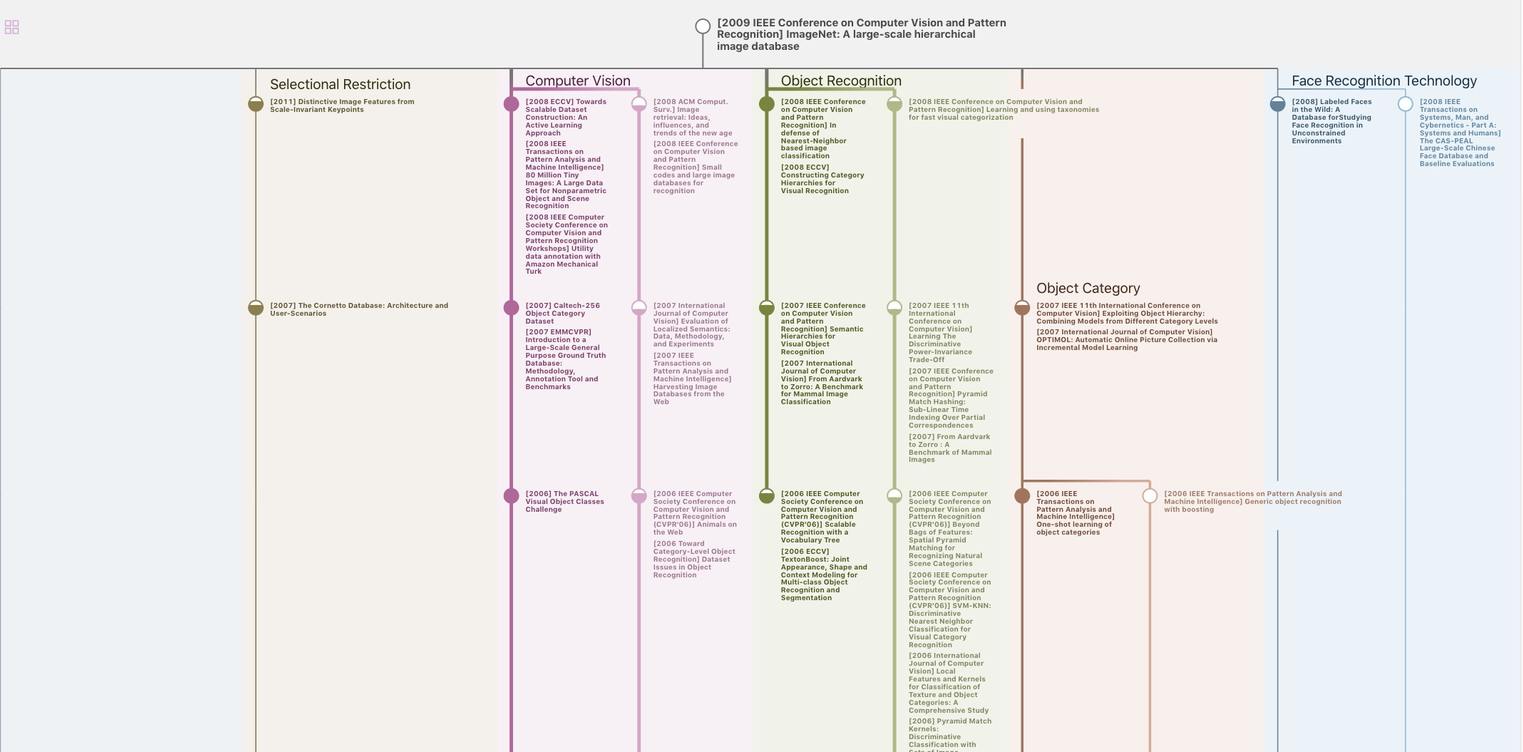
生成溯源树,研究论文发展脉络
Chat Paper
正在生成论文摘要