O-2A: Outlier-Aware Compression for 8-bit Post-Training Quantization Model.
IEEE Access(2023)
摘要
Post Training Quantization (PTQ) is a practical and cost-effective technique that reduces main memory footprint of Deep Neural Networks (DNNs). However, the effectiveness of PTQ is limited by a notable decrease in accuracy when the precision falls below 8 bits. To overcome this limitation of PTQ, we present a new compression method called Outlier Aware Approximation (O-2A) that compresses 8-bit PTQ models into lower precision with minimal accuracy loss. In O-2A, parameters are classified into outliers and non-outliers. Critical bits of outliers are preserved, while unnecessary bits of non-outliers are removed, resulting in reduced compression error. Nevertheless, compressing outliers using O-2A below 6 bits precision poses challenges due to significant compression error. To achieve more aggressive compression, we introduce multiple levels of O-2A (mO-2A), where outliers are divided into different levels, and compression error is minimized. We evaluate our techniques on the ImageNet dataset using the Pytorch framework. The results demonstrate that our methods outperform previous works in term of accuracy with the same compression efficiency.
更多查看译文
关键词
ourlier-aware,post-training
AI 理解论文
溯源树
样例
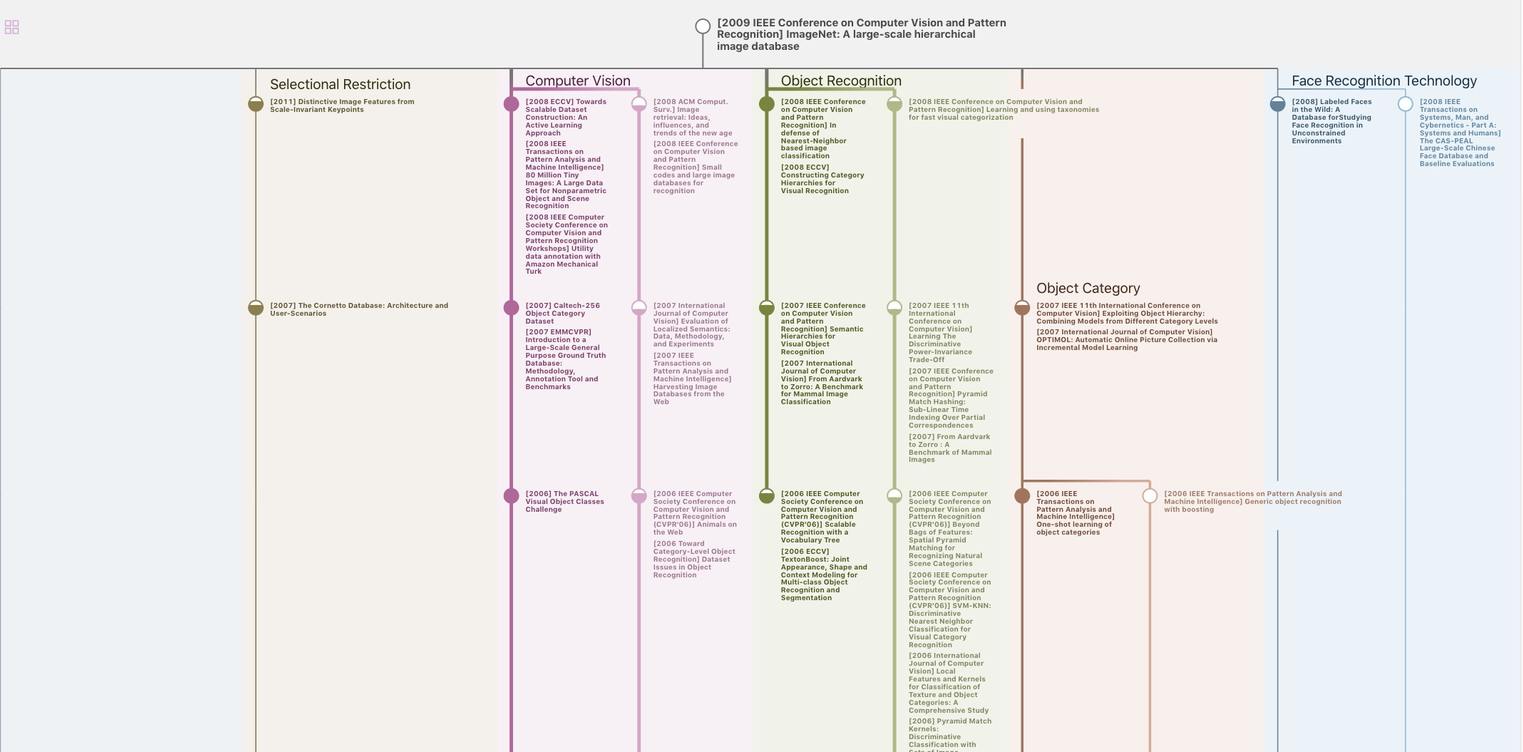
生成溯源树,研究论文发展脉络
Chat Paper
正在生成论文摘要