Bit-Plane and Correlation Spatial Attention Modules for Plant Disease Classification.
IEEE Access(2023)
摘要
The effective and accurate classification of plant diseases is an important task for agricultural production. Therefore, some studies have utilized convolutional neural networks to identify categories of plant diseases, which can effectively reduce the reliance on crop experts. To further improve the accuracy of plant disease classification methods based on convolutional neural network, this paper proposes an attention model designed for plant disease classification tasks. The proposed attention model contains bit-plane attention and a correlation spatial attention. The bit-plane attention localizes disease areas by exploiting bit-plane information. The correlation spatial attention enhances the weight of important areas in the feature map by establishing the correlation between different areas. The accuracy of the proposed attention model inserted into ResNet101 on the AI Challenger 2018 and PlantVillage datasets is 87.11% and 99.82%, respectively. The performance is better than that of other methods studied on the public plant disease classification dataset. Experiments show that the proposed attention model outperforms the widely used universal attention models SE, CBAM, CA, ECA, BAM and GC. In addition, ablation experiments are conducted to verify the influences of different variants of the proposed attention model on the results.
更多查看译文
关键词
correlation spatial attention modules,plant disease classification,bit-plane
AI 理解论文
溯源树
样例
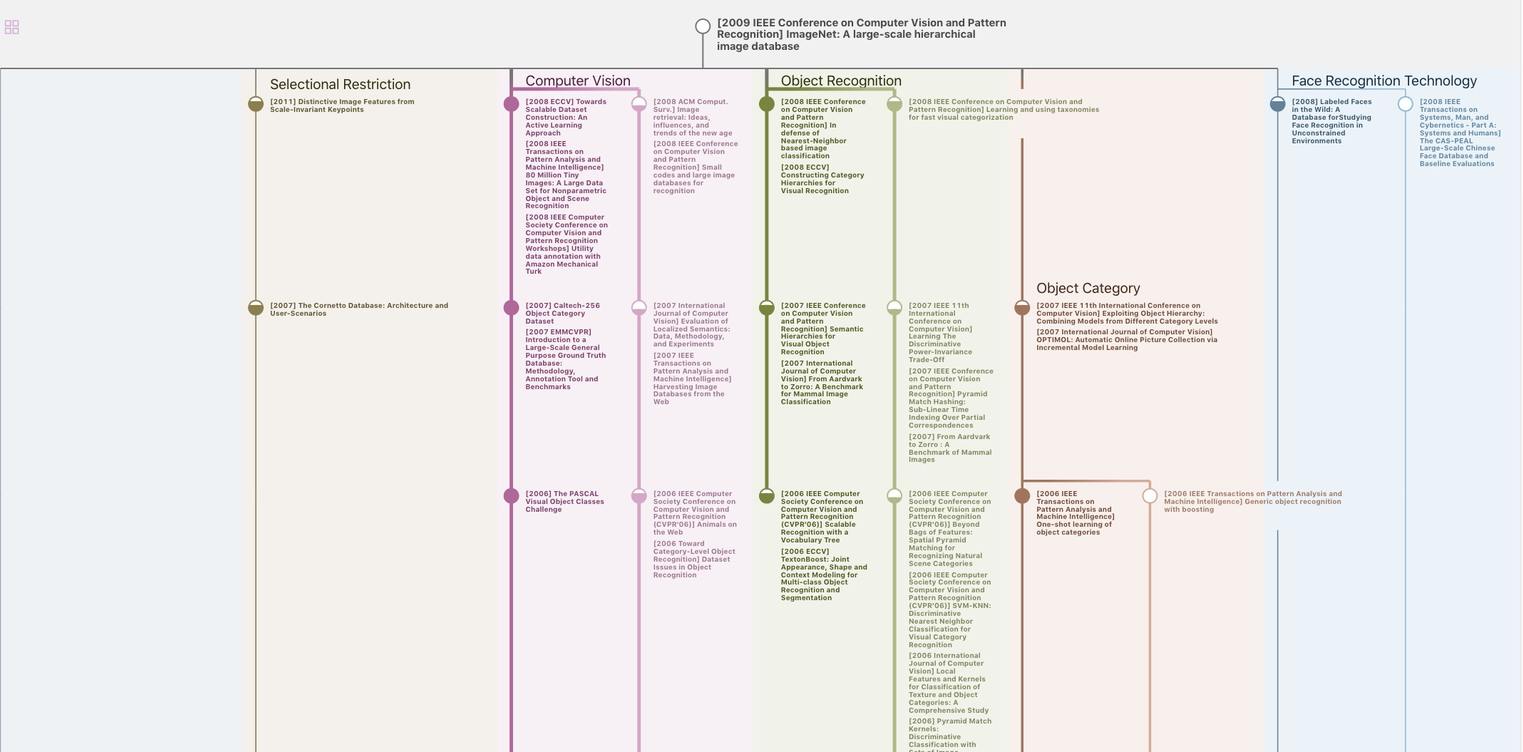
生成溯源树,研究论文发展脉络
Chat Paper
正在生成论文摘要